Let's say we are training a small machine which is a simple classification task. Here's some nomenclature you'll need: the in-sample data is the data the model learns from and the out-of-sample data is the data the model has never seen before. One of the pitfalls many new data scientists make is that they measure their model's effectiveness on the same data that the model learned from. What this ends up doing is rewarding the model's ability to memorize, rather than its ability to generalize, which is a huge difference.
If you take a look at the two examples here, the first presents a sample that the model learned from, and we can be reasonably confident that it's going to predict one, which would be correct. The second example presents a new sample, which appears to resemble more of the zero class. Of course, the model doesn't know that. But a good model should be able to recognize and generalize this pattern, shown as follows:
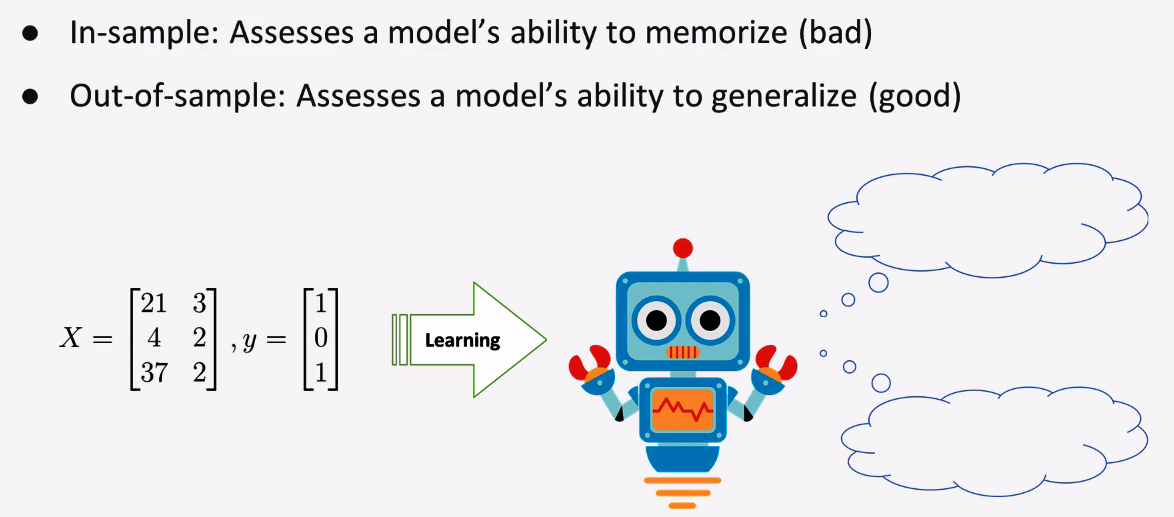
So, now the question is how we can ensure both in-sample and out-of-sample data for the model to prove its worth. Even more precisely, our out-of-sample data needs to be labeled. New or unlabeled data won't suffice because we have to know the actual answer in order to determine how correct the model is. So, one of the ways we can handle this in machine learning is to split our data into two parts: a training set and a testing set. The training set is what our model will learn on; the testing set is what our model will be evaluated on. How much data you have matters a lot. In fact, in the next sections, when we discuss the bias-variance trade-off, you'll see how some models require much more data to learn than others do.
Another thing to keep in mind is that if some of the distributions of your variables are highly skewed, or you have rare categorical levels embedded throughout, or even class imbalance in your y vector, you may end up getting a bad split. As an example, let's say you have a binary feature in your X matrix that indicates the presence of a very rare sensor for some event that occurs every 10,000 occurrences. If you randomly split your data and all of the positive sensor events are in your test set, then your model will learn from the training data that the sensor is never tripped and may deem that as an unimportant variable when, in reality, it could be hugely important, and hugely predictive. So, you can control these types of issues with stratification.