Data normalization
Data normalization is a critical step in machine learning to bring data to a similar scale. It is also known as feature scaling and is performed as data preprocessing.
Note
The correct normalization is very critical in neural networks, else it will lead to saturation within the hidden layers, which in turn leads to zero gradient and no learning will be possible.
Getting ready
There are multiple ways to perform normalization:
- Min-max standardization: The min-max retains the original distribution and scales the feature values between [0, 1], with 0 as the minimum value of the feature and 1 as the maximum value. The standardization is performed as follows:
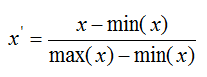
Here, x' is the normalized value of the feature. The method is sensitive to outliers in the dataset.
- Decimal scaling: This form of scaling is used where values of different decimal ranges are present. For example, two features with different bounds can be brought to a similar scale using decimal scaling as follows:
x'=x/10n
- Z-score...