A generic definition of transfer learning is that it is a deep learning technique that reuses knowledge gained from solving one problem by applying it to a related but different problem. Let's understand this by looking at an example. Let's say that we have three types of flowers—namely, a rose, a sunflower, and a tulip. We can use the standard pretrained models, such as VGG16/19, ResNet50, or InceptionV3 models (pretrained on ImageNet with 1,000 output classes, listed at https://gist.github.com/yrevar/942d3a0ac09ec9e5eb3a) to classify the flower images, but our model won't correctly classify them since these flower categories were not there in the ground-truth classes that the model was trained on. In other words, they are classes that the model is not aware of. The following image shows how the...




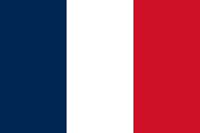


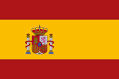



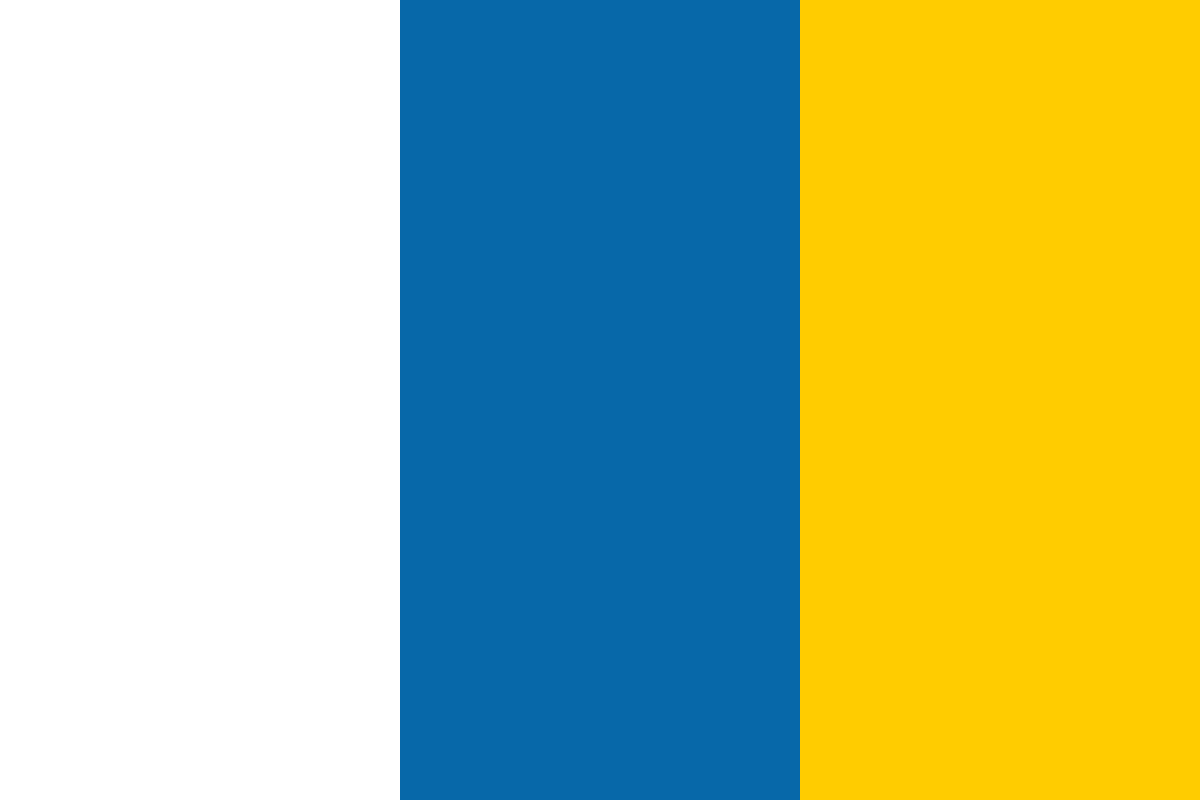
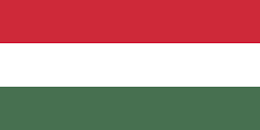

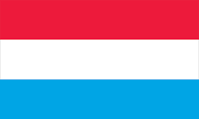





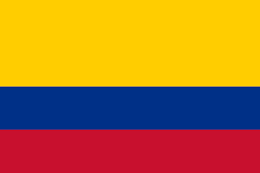
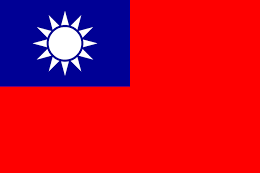
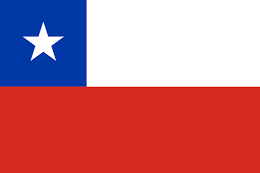



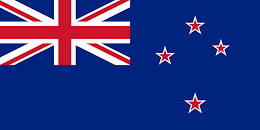
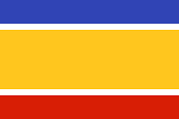
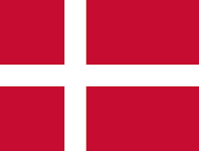
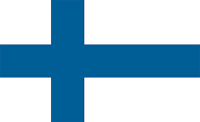







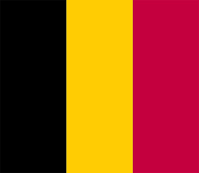

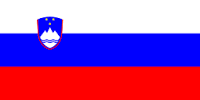





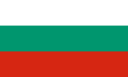


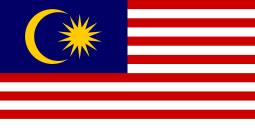



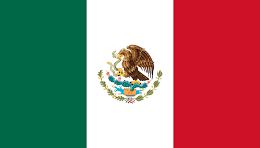
