Up until now, we have used neural networks such as the MLP, feedforward neural network, and CNN in our projects. The constraint faced by these neural networks is that they only accept a fixed input vector such as an image, and output another vector. The high-level architecture of these neural networks can be summarized by the following diagram:

This restrictive architecture makes it difficult for CNNs to work with sequential data. To work with sequential data, the neural network needs to take in specific bits of the data at each time step, in the sequence that it appears. This provides the idea for an RNN. An RNN has high-level architecture, as shown in the following diagram:
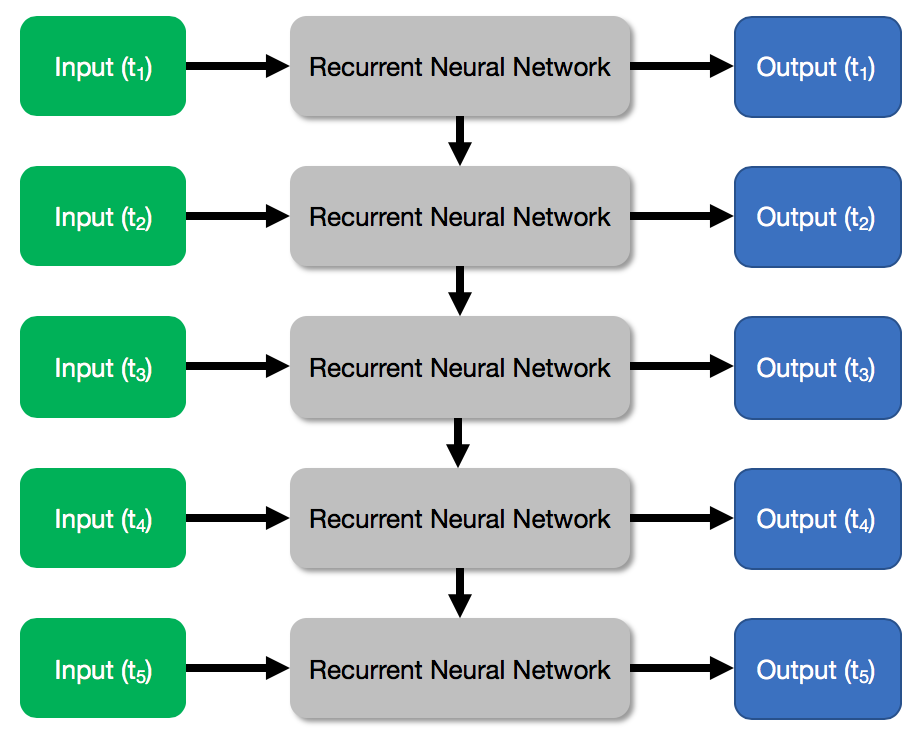
From the previous diagram, we can see that an RNN is a multi-layered neural network. We can break up the raw input, splitting it into time steps. For example, if the raw input is a sentence, we can...