Introduction to long short term memory networks
The vanishing gradient problem has appeared as the biggest obstacle to recurrent networks.
As the straight line changes along the x axis with a slight change in the y axis, the gradient shows change in all the weights with regard to change in error. If we don't know the gradient, we will not be able to adjust the weights in a direction that will reduce the loss or error, and our neural network ceases to learn.
Long short term memories (LSTMs) are designed to overcome the vanishing gradient problem. Retaining information for a larger duration of time is effectively their implicit behavior.
In standard RNNs, the repeating cell will have an elementary structure, such as a singletanh layer:
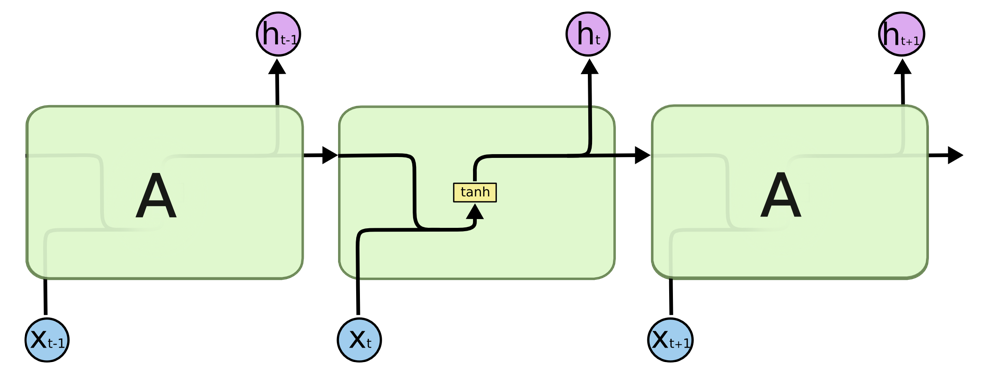
As seen in the preceding image, LSTMs also have a chain-like structure, but the recurrent cell has a different structure:
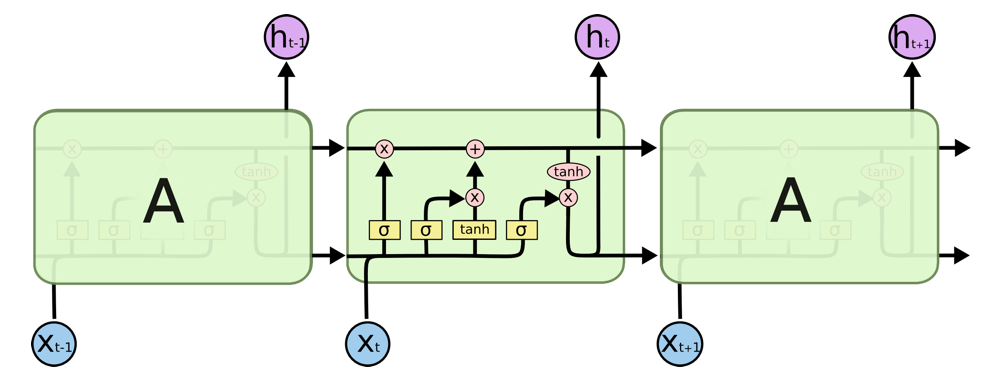
Life cycle of LSTM
The key to LSTMs is the cell state that is like a conveyor belt. It moves down the stream with minor linear interactions. It...