The Naïve Bayes classifier
We now have the necessary tools to learn about our first and simplest graphical model, the Naïve Bayes classifier. This is a directed graphical model that contains a single parent node and a series of child nodes representing random variables that are dependent only on this node with no dependencies between them. Here is an example:
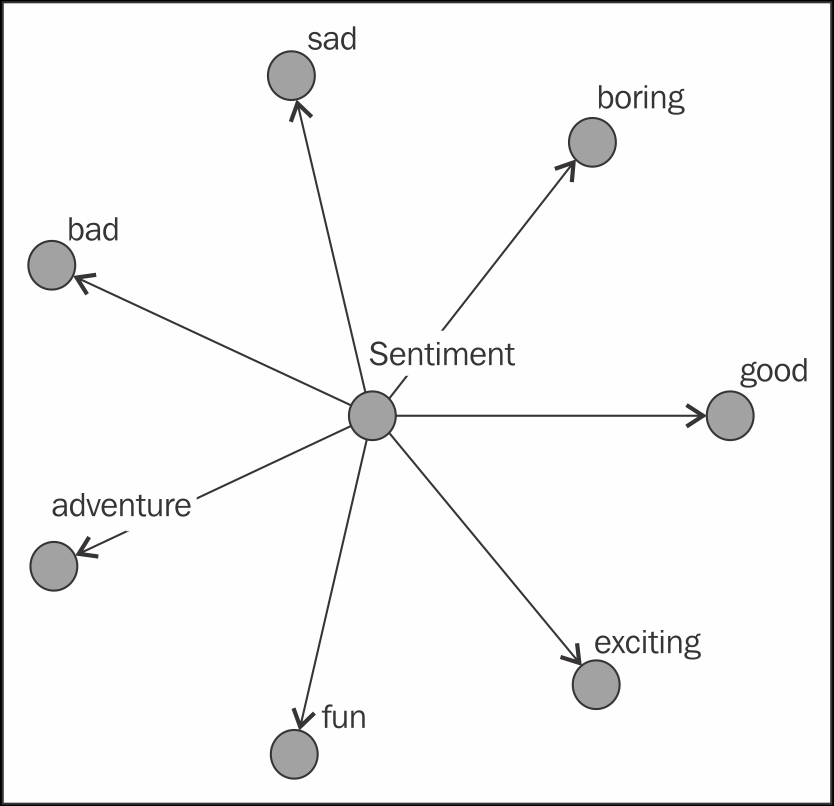
We usually interpret our single parent node as the causal node, so in our particular example, the value of the Sentiment node will influence the value of the sad node, the fun node, and so on. As this is a Bayesian network, the local Markov property can be used to explain the core assumption of the model. Given the Sentiment node, all other nodes are independent of each other.
In practice, we use the Naïve Bayes classifier in a context where we can observe and measure the child nodes and attempt to estimate the parent node as our output. Thus, the child nodes will be the input features of our model, and the parent node will be the output...