Kernel types
We're going to explain the types of in this section.
Linear kernel
Let's say there are two vectors, x1 and x2, so the linear kernel can be defined by the following:
K(x1, x2)= x1 . x2
Polynomial kernel
If there are two vectors, x1 and x2, the linear kernel can be defined by the following:
K(x1, x2)= (x1 . x2 + c)d
Where:
- c: Constant
- d: Degree of polynomial:
def polynomial_kernel(x1, x2, degree, constant=0): result = sum([x1[i] * x2[i] for i in range(len(x1))]) + constant return pow(result, degree)
If we use the same x1
and x2
as used previously, we get the following:
x1= [4,8] x2=[20,30] polynomial_kernel(x1,x2,2,0) # result would be 102400
If we increase the degree of polynomial, we will try to get influenced by other vectors as the decision boundary becomes too complex and it will result in overfitting:
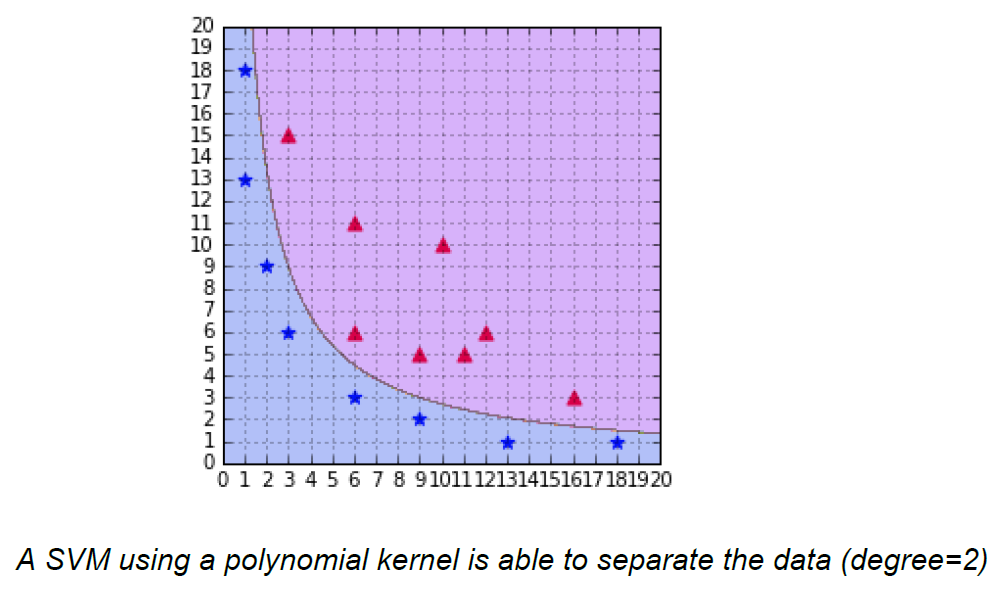
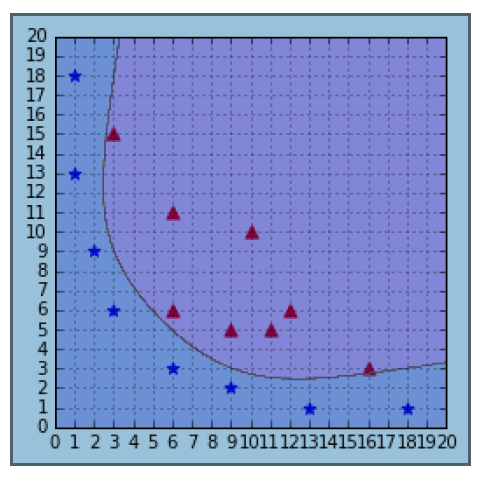
Polynomial kernel using degree as 6.
Gaussian kernel
The polynomial kernel has given us a good boundary line. But can we work with polynomial kernels all the time? Not in the following...