Cross-validation and model selection
We have already spoken about overfitting. It is something to do with the stability of a model since the real test of a model occurs when it works on unseen and new data. One of the most important aspects of a model is that it shouldn't pick up on noise, apart from regular patterns.
Validation is nothing but an assurance of the model being a relationship between the response and predictors as the outcome of input features and not noise. A good indicator of the model is not through training data and error. That's why we need cross-validation.
Here, we will stick with k-fold cross-validation and understand how it can be used.
K-fold cross-validation
Let's walk through the steps of k-fold cross-validation:
- The data is divided into k-subsets.
- One set is kept for testing/development and the model is built on the rest of the data (k-1). That is, the rest of the data forms the training data.
- Step 2 is repeated k-times. That is, once the preceding step has been performed, we move on to the second set and it forms a test set. The rest of the (k-1) data is then available for building the model:
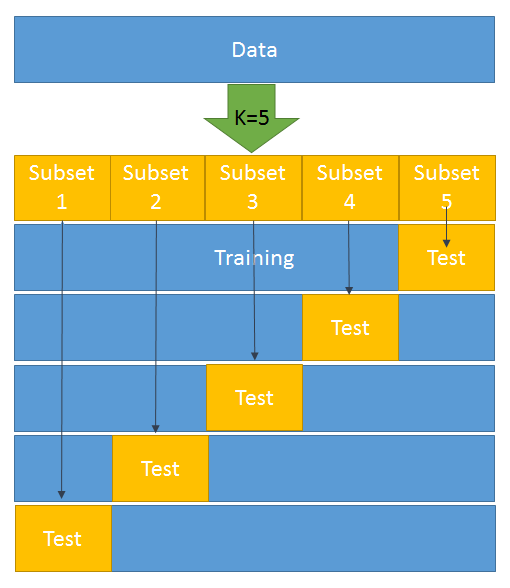
4. An error is calculated and an average is taken over all k-trials.
Every subset gets one chance to be a validation/test set since most of the data is used as a training set. This helps in reducing bias. At the same time, almost all the data is being used as validation set, which reduces variance.
As shown in the preceding diagram, k = 5 has been selected. This means that we have to divide the whole dataset into five subsets. In the first iteration, subset 5 becomes the test data and the rest becomes the training data. Likewise, in the second iteration, subset 4 turns into the test data and the rest becomes the training data. This goes on for five iterations.
Now, let's try to do this in Python by splitting the train and test data using the K neighbors classifier:
from sklearn.datasets import load_breast_cancer # importing the dataset from sklearn.cross_validation import train_test_split,cross_val_score # it will help in splitting train & test from sklearn.neighbors import KNeighborsClassifier from sklearn import metrics BC =load_breast_cancer() X = BC.data y = BC.target X_train, X_test, y_train, y_test = train_test_split(X, y, random_state=4) knn = KNeighborsClassifier(n_neighbors=5) knn.fit(X_train, y_train) y_pred = knn.predict(X_test) print(metrics.accuracy_score(y_test, y_pred)) knn = KNeighborsClassifier(n_neighbors=5) scores = cross_val_score(knn, X, y, cv=10, scoring='accuracy') print(scores) print(scores.mean())