ML pipelines – how to use ML in the system in practice
Training and validating ML models on a local platform is the beginning of the process of using an ML pipeline. After all, it would be of limited use if we had to retrain the ML models on every computer from our customers.
Therefore, we often deploy ML models to a model repository. There are a few popular ones, but the one that is used by the largest community is the HuggingFace repository. In that repository, we can deploy both the models and datasets and even create spaces where the models can be used for experiments without the need to download them. Let us deploy the model trained in Chapter 11 to that repository. For that, we need to have an account at huggingface.com, and then we can start.
Deploying models to HuggingFace
First, we need to create a new model using the New button on the main page, as in Figure 12.2:
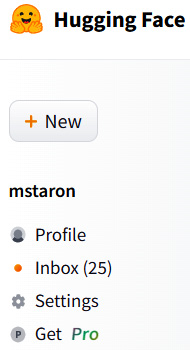
Figure 12.2 – New button to create a model
Then, we fill...