Visualizing with R
There are severalvisualization mechanisms available in R.
- Produce a R Scatter plot
In this example, we produce a scatter plot using the standard R plot()
function. Built into the plot function, we can chart the relationship between the x
and y
values as well.
How to do it...
We can use this script:
# load the iris dataset data <- read.csv("http://archive.ics.uci.edu/ml/machine-learning-databases/iris/iris.data") #Let us also clean up the data so as to be more readable colnames(data) <- c("sepal_length", "sepal_width", "petal_length", "petal_width", "species") # make sure the data is as expected summary(data)
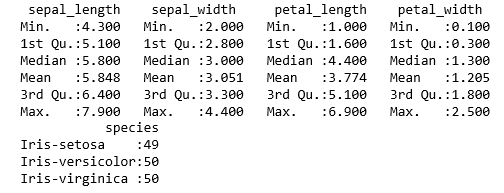
Produce the Scatter plot:
plot(data$sepal_length, data$petal_length)
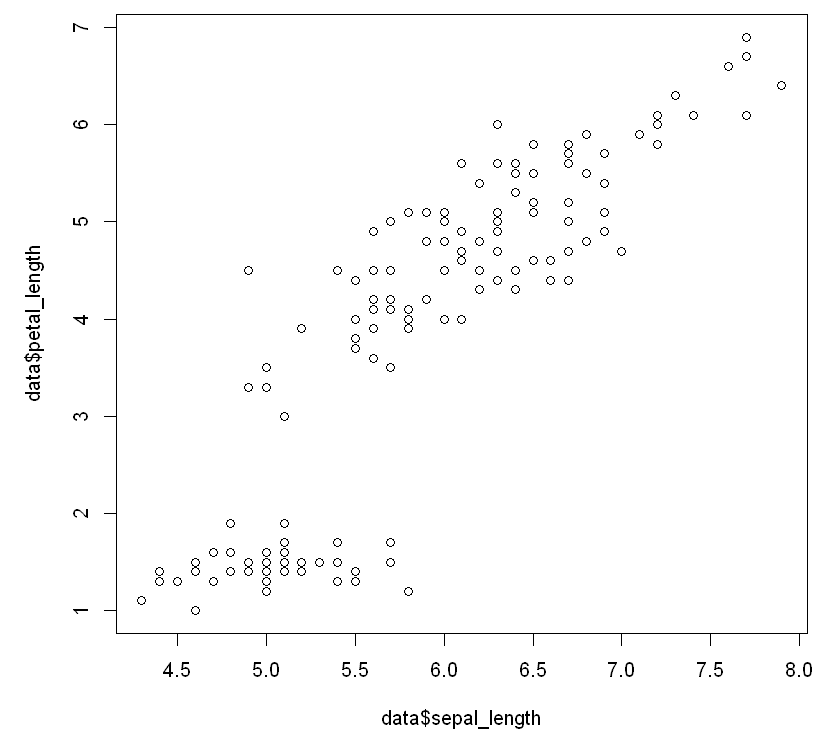
And produce the following visualizations of the relationships between the values. This plot is a stepwise look at how changing one value appears, to see the effect on the other:
plot(data$sepal_length, data$petal_length, type="s")
The output is as follows:
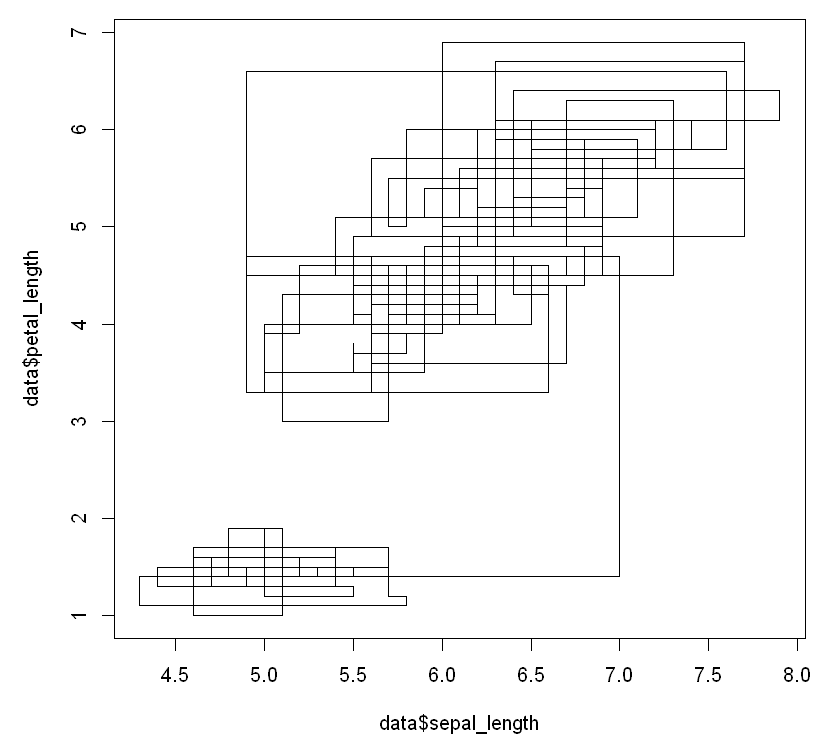
And we look at a histogram of the same data...