SARSA, which is the process this method emulates. That is, the algorithm works by moving to a state, then choosing an action, receiving a reward, and then moving to the next state action. This makes SARSA an on-policy method, that is, the algorithm works by learning and deciding with the same policy. This differs from Q-learning, as we saw in Chapter 4, Temporal Difference Learning, where Q is a form of off-policy learner.
The following diagram shows the difference in backup diagrams for Q-learning and SARSA:
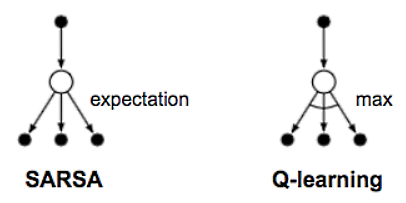
Recall that our Q-learner is an off-policy learner. That is, it requires the algorithm to update the policy or Q table offline and then later make decisions from that. However, if we want to tackle the TDL problem beyond one step or TD (0), then we need to have an on-policy learner. Our learning agent or...