Performing benchmarking on a Hadoop cluster
The Hadoop framework supports built-in libraries so that we can perform benchmarking in order to take a look at how the Hadoop cluster configurations/hardware are performing. There are plenty of tests available that will perform the benchmarking of various aspects of the Hadoop cluster. In this recipe, we are going to take a look at how to perform benchmarking and read the results.
Getting ready
To perform this recipe, you should have a Hadoop cluster up and running.
How to do it...
The Hadoop framework supports built-in support to benchmark various aspects. These tests are written in a library called hadoop-mapreduce-client-jobclient-2.7.0-tests.jar
To know the list of all the supported tests, you can execute the following command:
hadoop jar /usr/local/hadoop/share/hadoop/mapreduce/hadoop-mapreduce-client-jobclient-2.7.0-tests.jar
The result of the command will be similar to what is shown in this screenshot:
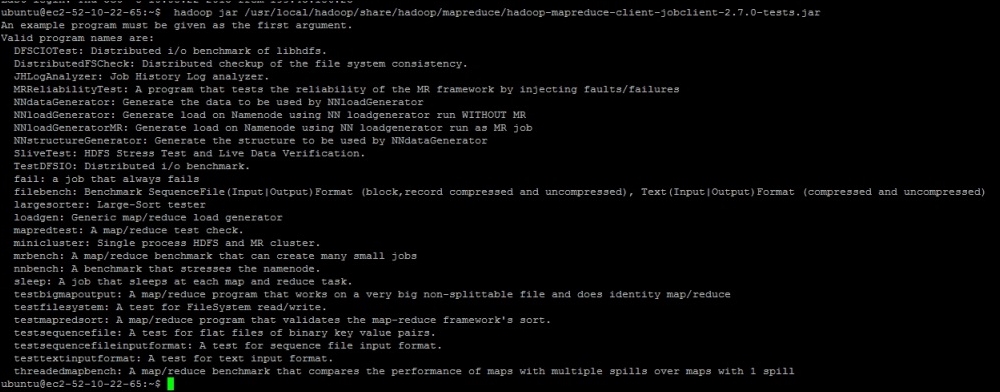
TestDFSIO
This is one the major tests that you may want to do in order to see how DFS is performing. So, we are now going to take a look at how to use these tests to know how efficiently HDFS is able to write and read data.
As seen in the preceding screenshot, the library provides tools to test DFS through an option called TestDFSIO
. Now, let's execute the write test in order to understand how efficiently HDFS is able to write big files. The following is the command to execute the write test:
hadoop jar /usr/local/hadoop/share/hadoop/mapreduce/hadoop-mapreduce-client-jobclient-2.7.0-tests.jar TestDFSIO -write -nrFiles 2 -fileSize 1GB -resFile /tmp/TestDFSIOwrite.txt
Once you initiate the preceding command, a map reduce job will start, which will write two files to HDFS that are 1GB in size . You can choose any numbers based on your cluster size. These tests create data in HDFS under the /benchmarks
directory. Once the execution is complete, you will see these results:
15/10/08 11:37:23 INFO fs.TestDFSIO: ----- TestDFSIO ----- : write 15/10/08 11:37:23 INFO fs.TestDFSIO: Date & time: Thu Oct 08 11:37:23 UTC 2015 15/10/08 11:37:23 INFO fs.TestDFSIO: Number of files: 2 15/10/08 11:37:23 INFO fs.TestDFSIO: Total MBytes processed: 2048.0 15/10/08 11:37:23 INFO fs.TestDFSIO: Throughput mb/sec: 26.637185406776354 15/10/08 11:37:23 INFO fs.TestDFSIO: Average IO rate mb/sec: 26.63718605041504 15/10/08 11:37:23 INFO fs.TestDFSIO: IO rate std deviation: 0.00829867575568246 15/10/08 11:37:23 INFO fs.TestDFSIO: Test exec time sec: 69.023
The preceding data is calculated from the RAW data generated by the Map Reduce program. You can also view the raw data as follows:
hdfs dfs -cat /benchmarks/TestDFSIO/io_read/part* f:rate 53274.37 f:sqrate 1419079.2 l:size 2147483648 l:tasks 2 l:time 76885
Tip
The following formulae are used to calculate throughput, the average IO rate, and standard deviation.
Throughput = size * 1000/time * 1048576
Average IO rate = rate/1000/tasks
Standard deviation = square root of (absolute value(sqrate/1000/tasks – Average IO Rate * Average IO Rate))
Similarly, you can perform benchmarking of HDFS read operations as well:
hadoop jar /usr/local/hadoop/share/hadoop/mapreduce/hadoop-mapreduce-client-jobclient-2.7.0-tests.jar TestDFSIO -read -nrFiles 2 -fileSize 1GB -resFile /tmp/TestDFSIOread.txt
At the end of the execution, a reducer will collect the data from the RAW results, and you will see calculated numbers for the DFSIO reads:
15/10/08 11:41:01 INFO fs.TestDFSIO: ----- TestDFSIO ----- : read 15/10/08 11:41:01 INFO fs.TestDFSIO: Date & time: Thu Oct 08 11:41:01 UTC 2015 15/10/08 11:41:01 INFO fs.TestDFSIO: Number of files: 2 15/10/08 11:41:01 INFO fs.TestDFSIO: Total MBytes processed: 2048.0 15/10/08 11:41:01 INFO fs.TestDFSIO: Throughput mb/sec: 33.96633220001659 15/10/08 11:41:01 INFO fs.TestDFSIO: Average IO rate mb/sec: 33.968116760253906 15/10/08 11:41:01 INFO fs.TestDFSIO: IO rate std deviation: 0.24641533955938721 15/10/08 11:41:01 INFO fs.TestDFSIO: Test exec time sec: 59.343
Here, we can take a look at the RAW data as well:
hdfs dfs -cat /benchmarks/TestDFSIO/io_read/part* f:rate 67936.234 f:sqrate 2307787.2 l:size 2147483648 l:tasks 2 l:time 60295
The same formulae are used to calculate the throughput, average IO rate, and standard deviation.
This way, you can benchmark the DFSIO reads and writes.
NNBench
Similar to DFS IO, we can also perform benchmarking for NameNode
:
hadoop jar /usr/local/hadoop/share/hadoop/mapreduce/hadoop-mapreduce-client-jobclient-2.7.0-tests.jar nnbench -operation create_write
MRBench
MRBench helps us understand the average time taken for a job to execute for a given number of mappers and reducers. The following is a sample command to execute MRBench with default parameters:
hadoop jar /usr/local/hadoop/share/hadoop/mapreduce/hadoop-mapreduce-client-jobclient-2.7.0-tests.jar mrbench
How it works...
Hadoop benchmark tests use the parameters and conditions provided by users. For every test, it executes a map reduce job and once complete, it displays the results on the screen. Generally, it is recommended that you run the benchmarking tests as soon as you have installed the Hadoop cluster in order to predict the performance of HDFS/Map Reduce and so on.
Most of the tests require a sequence in which they should be executed, for example, all write tests should be executed first, then read/delete, and so on.
Once the complete execution is done, make sure you clean up the data in the /benchmarks
directory in HDFS.
Here is an example command to clean up the data generated by the TestDFSIO
tests:
hadoop jar /usr/local/hadoop/share/hadoop/mapreduce/hadoop-mapreduce-client-jobclient-2.7.0-tests.jar TestDFSIO -clean