In the previous recipe, we generated a model for a stationary time series using an ARMA model, which consists of an autoregressive (AR) component and an moving average (MA) component. Unfortunately, this model cannot accommodate time series that have some underlying trend; that is, they are not stationary time series. We can often get around this by differencing the observed time series one or more times until we obtain a stationary time series that can be modeled using ARMA. The incorporation of differencing into an ARMA model is called an ARIMA model, which stands for Autoregressive (AR) Integrated (I) Moving Average (MA).
Differencing is the process of computing the difference of consecutive terms in a sequence of data. So, applying first-order differencing amounts to subtracting the value at the current step from the value at the next step (ti+1 - ti). This has the effect of removing the underlying upward or downward...




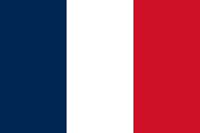


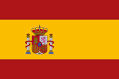



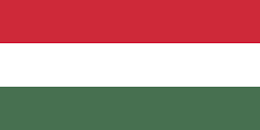

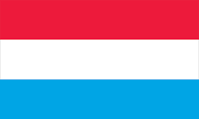





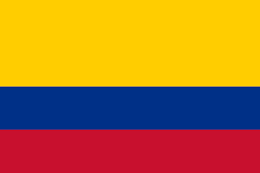
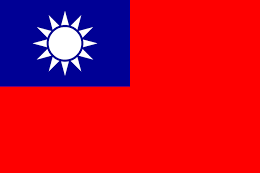
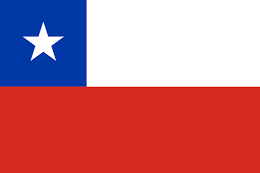



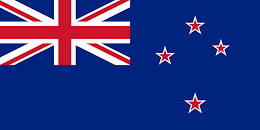
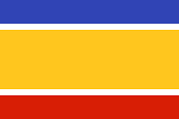
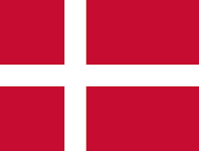
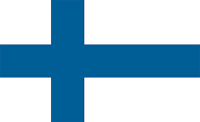







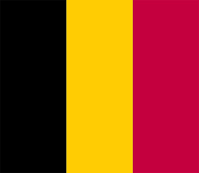

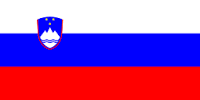





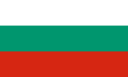


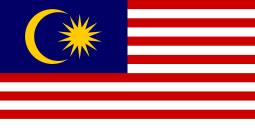



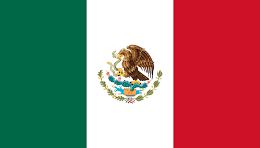
