



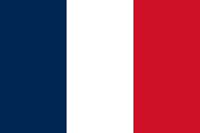


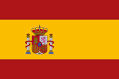



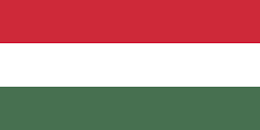

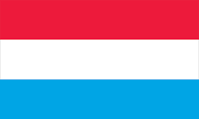





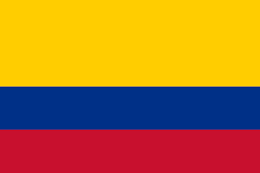
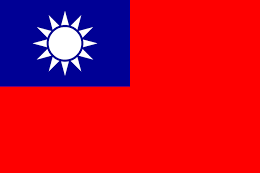
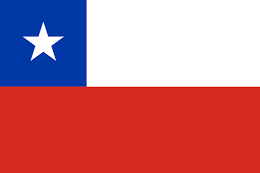



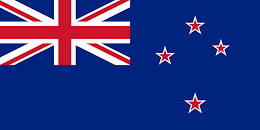
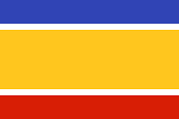
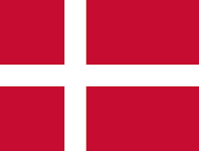
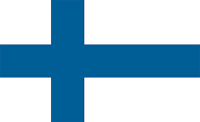







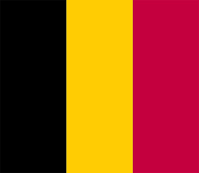

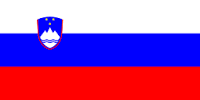





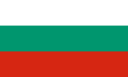


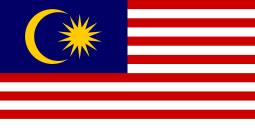



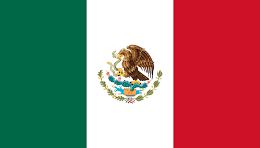

https://twitter.com/HanieSedghi/status/1125401294880083968
The first day of the conference started with a talk on Highlights of Recent Developments in Algorithmic Fairness by Cynthia Dwork, an American computer scientist at Harvard University. She focused on "group fairness" notions that address the relative treatment of different demographic groups. And she talked on research in the ML community that explores fairness via representations. The investigation of scoring, classifying, ranking, and auditing fairness was also discussed in this talk by Dwork.
https://twitter.com/NalKalchbrenner/status/1125455415553208321
Jacob Menick, a senior research engineer at Google, Deep Mind and Nal Kalchbrenner, staff research scientist and co-creator of the Google Brain Amsterdam research lab talked on Generating high fidelity images with Subscale Pixel Networks and Multidimensional Upscaling. They talked about the challenges involved in generating the images and how they address this issue with the help of Subscale Pixel Network (SPN). It is a conditional decoder architecture that helps in generating an image as a sequence of image slices of equal size. They also explained how Multidimensional Upscaling is used to grow an image in both size and depth via intermediate stages corresponding to distinct SPNs.
There were in all 10 workshops conducted on the same day based on AI and deep learning covering topics such as,
The first day also witnessed a few interesting talks on neural networks covering topics such as The Lottery Ticket Hypothesis: Finding Sparse, Trainable Neural Networks, How Powerful are Graph Neural Networks? etc. Overall the first day was quite enriching and informative.
https://twitter.com/natanielruizg/status/1125763990158807040
Tuesday, also the second day of the conference, started with an interesting talk on Can Machine Learning Help to Conduct a Planetary Healthcheck? by Emily Shuckburgh, a Climate scientist and deputy head of the Polar Oceans team at the British Antarctic Survey. She talked about the sophisticated numerical models of the Earth’s systems which have been developed so far based on physics, chemistry and biology. She then highlighted a set of "grand challenge" problems and discussed various ways in which Machine Learning is helping to advance our capacity to address these.
On the second day of ICLR 2019, Chris Sander, computational biologist, John Ingraham, Adam J Riesselman, and Debora Marks from Harvard University, talked on Learning protein structure with a differentiable simulator. They about the protein folding problem and their aim to bridge the gap between the expressive capacity of energy functions and the practical capabilities of their simulators by using an unrolled Monte Carlo simulation as a model for data. They also composed a neural energy function with a novel and efficient simulator which is based on Langevin dynamics for building an end-to-end-differentiable model of atomic protein structure given amino acid sequence information. They also discussed certain techniques for stabilizing backpropagation and demonstrated the model's capacity to make multimodal predictions.
https://twitter.com/natanielruizg/status/1125859734744117249
Day 2 was long and had Ian Goodfellow, a machine learning researcher and inventor of GANs, to talk on Adversarial Machine Learning. He talked about supervised learning works and making machine learning private, getting machine learning to work for new tasks and also reducing the dependency on large amounts of labeled data. He then discussed how the adversarial techniques in machine learning are involved in the latest research frontiers.
Day 2 covered poster presentation and a few talks on Enabling Factorized Piano Music Modeling and Generation with the MAESTRO Dataset, Learning to Remember More with Less Memorization, Learning to Remember More with Less Memorization, etc.
https://twitter.com/drew_jaegle/status/1125522499150721025
Day 3 of ICLR 2019 started with Pierre-Yves Oudeyer’s, research director at Inria talk on Developmental Autonomous Learning: AI, Cognitive Sciences and Educational Technology. He presented a research program that focuses on computational modeling of child development and learning mechanisms. He then discussed the several developmental forces that guide exploration in large real-world spaces. He also talked about the models of curiosity-driven autonomous learning that enables machines to sample and explore their own goals and learning strategies. He then explained how these models and techniques can be successfully applied in the domain of educational technologies.
Another interesting topic on the third day of ICLR 2019 was Generating knockoffs for feature selection using Generative Adversarial Networks (GAN) by James Jordon from Oxford University, Jinsung Yoon from California University, and Mihaela Schaar Professor at UCLA. The experts talked about the Generative Adversarial Networks framework that helps in generating knockoffs with no assumptions on the feature distribution. They also talked about the model they created which consists of 4 networks, a generator, a discriminator, a stability network and a power network. They further demonstrated the capability of their model to perform feature selection.
Followed by few more interesting topics like Deterministic Variational Inference for Robust Bayesian Neural Networks, there were series of poster presentations.
Today’s focus was more on neural models and neuro symbolic concepts. The day started with a talk on Learning natural language interfaces with neural models by Mirella Lapata, a computer scientist. She gave an overview of recent progress on learning natural language interfaces which allow users to interact with various devices and services using everyday language. She also addressed the structured prediction problem of mapping natural language utterances onto machine-interpretable representations. She further outlined the various challenges it poses and described a general modeling framework based on neural networks which tackle these challenges.
https://twitter.com/mmjb86/status/1126272417444311041
The next interesting talk was on Ordered neurons: Integrating tree structures into Recurrent Neural Networks by Professors Yikang Shen, Aaron Courville and Shawn Tan from Montreal University, and, Alessandro Sordoni, a researcher at Microsoft. In this talk, the experts focused on how they proposed a new RNN unit: ON-LSTM, which achieves good performance on four different tasks including language modeling, unsupervised parsing, targeted syntactic evaluation, and logical inference.
The last day of ICLR 2019 was exciting and helped the researchers present their innovations and attendees got a chance to interact with the experts.
To have a complete overview of each of these sessions, you can head over to ICLR’s Facebook page.
Paper in Two minutes: A novel method for resource efficient image classification
Google I/O 2019 D1 highlights: smarter display, search feature with AR capabilities, Android Q, linguistically advanced Google lens and more
Google I/O 2019: Flutter UI framework now extended for Web, Embedded, and Desktop