H2O is another very popular open source library to build machine learning models. It is produced by H2O.ai and supports multiple languages including R and Python. The H2O package is a multipurpose machine learning library developed for a distributed environment to run algorithms on big data.
Installing H2O in R
Getting ready
To set up H2O, the following systems are required:
- 64-bit Java Runtime Environment (version 1.6 or later)
- Minimum 2 GB RAM
H2O from R can be called using the h2o package. The h2o package has the following dependencies:
- RCurl
- rjson
- statmod
- survival
- stats
- tools
- utils
- methods
For machines that do not have curl-config installed, the RCurl dependency installation will fail in R and curl-config needs to be installed outside R.
How to do it...
- H2O can be installed directly from CRAN with the dependency parameter TRUE to install all CRAN-related h2o dependencies. This command will install all the R dependencies required for the h2o package:
install.packages("h2o", dependencies = T)
- The following command is used to call the h2o package in the current R environment. The first-time execution of the h2o package will automatically download the JAR file before launching H2O, as shown in the following figure:
library(h2o)
localH2O = h2o.init()
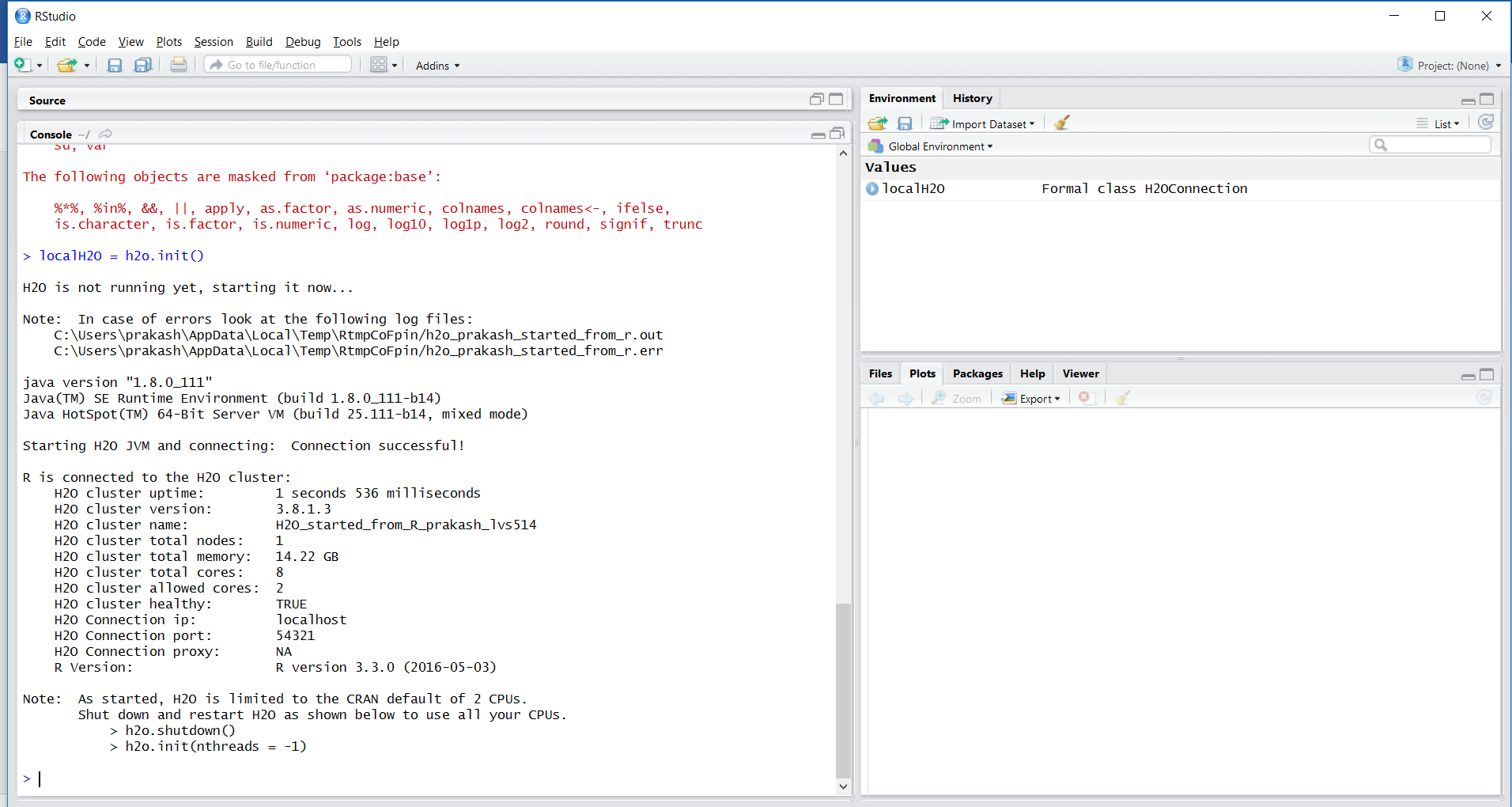
- The H2O cluster can be accessed using cluster ip and port information. The current H2O cluster is running on localhost at port 54321, as shown in the following screenshot:
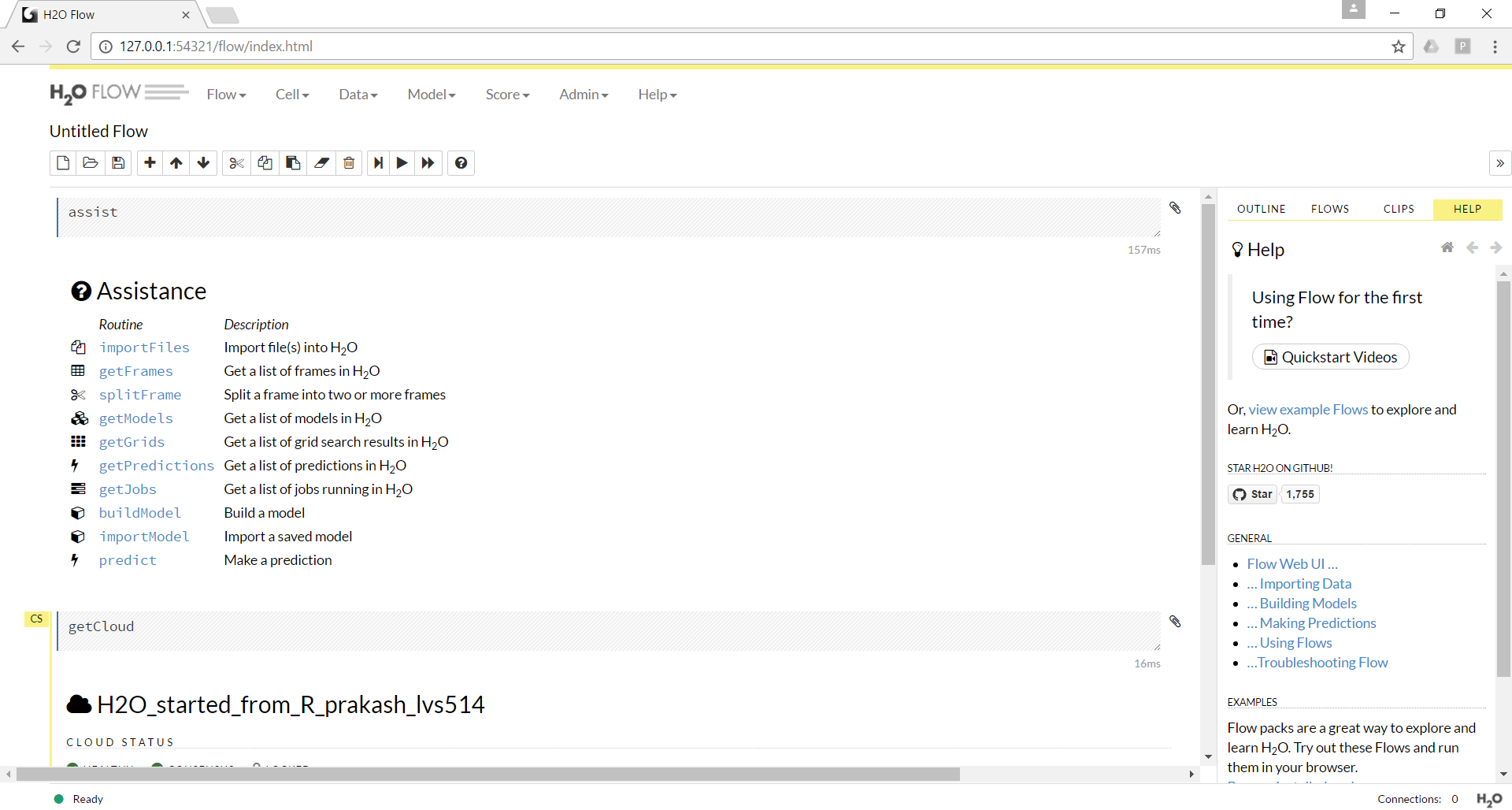
How it works...
Let's build a logistic regression interactively using the H2O browser.
- Start a new flow, as shown in the following screenshot:
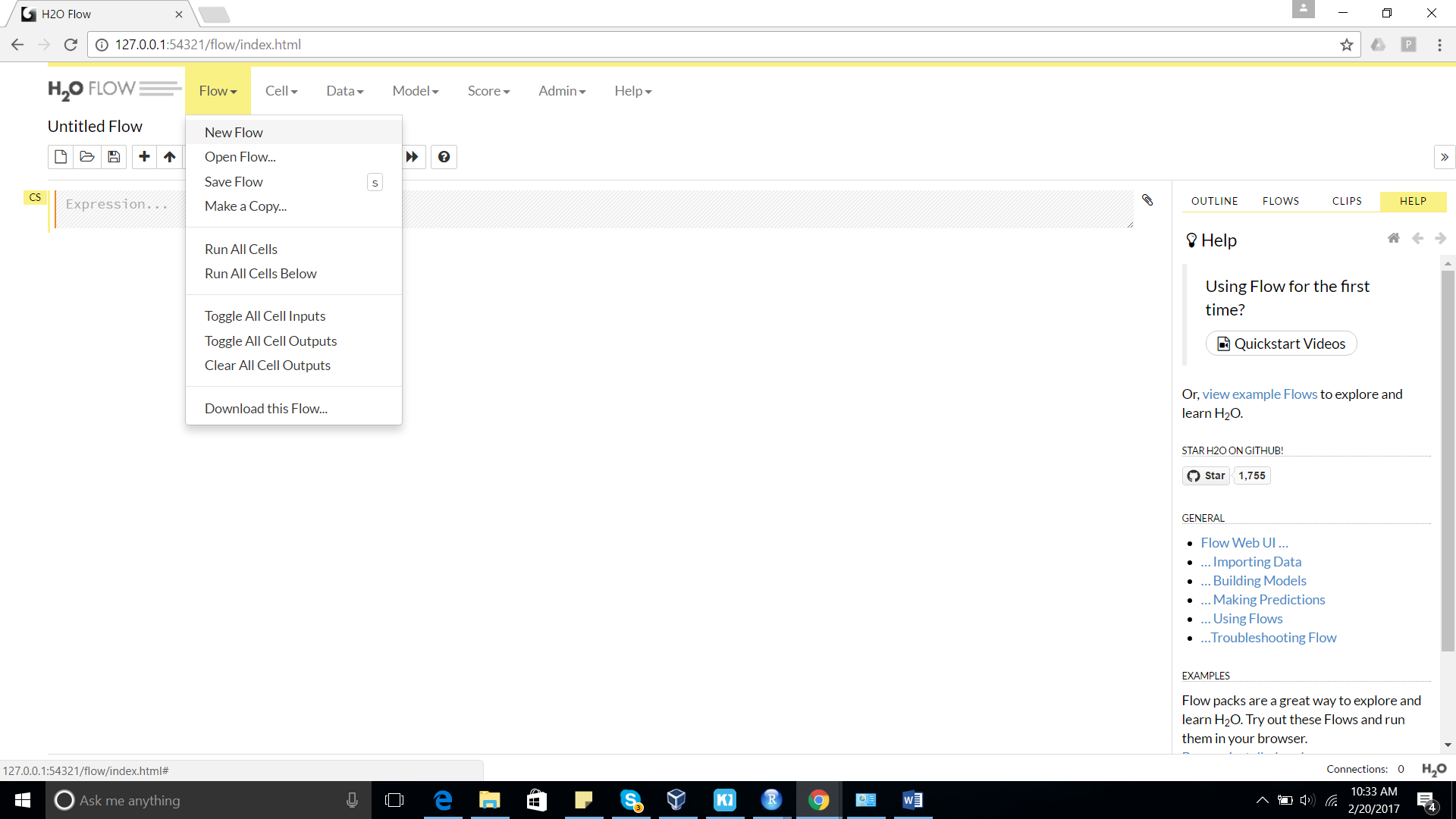
- Import a dataset using the Data menu, as shown in the following screenshot:
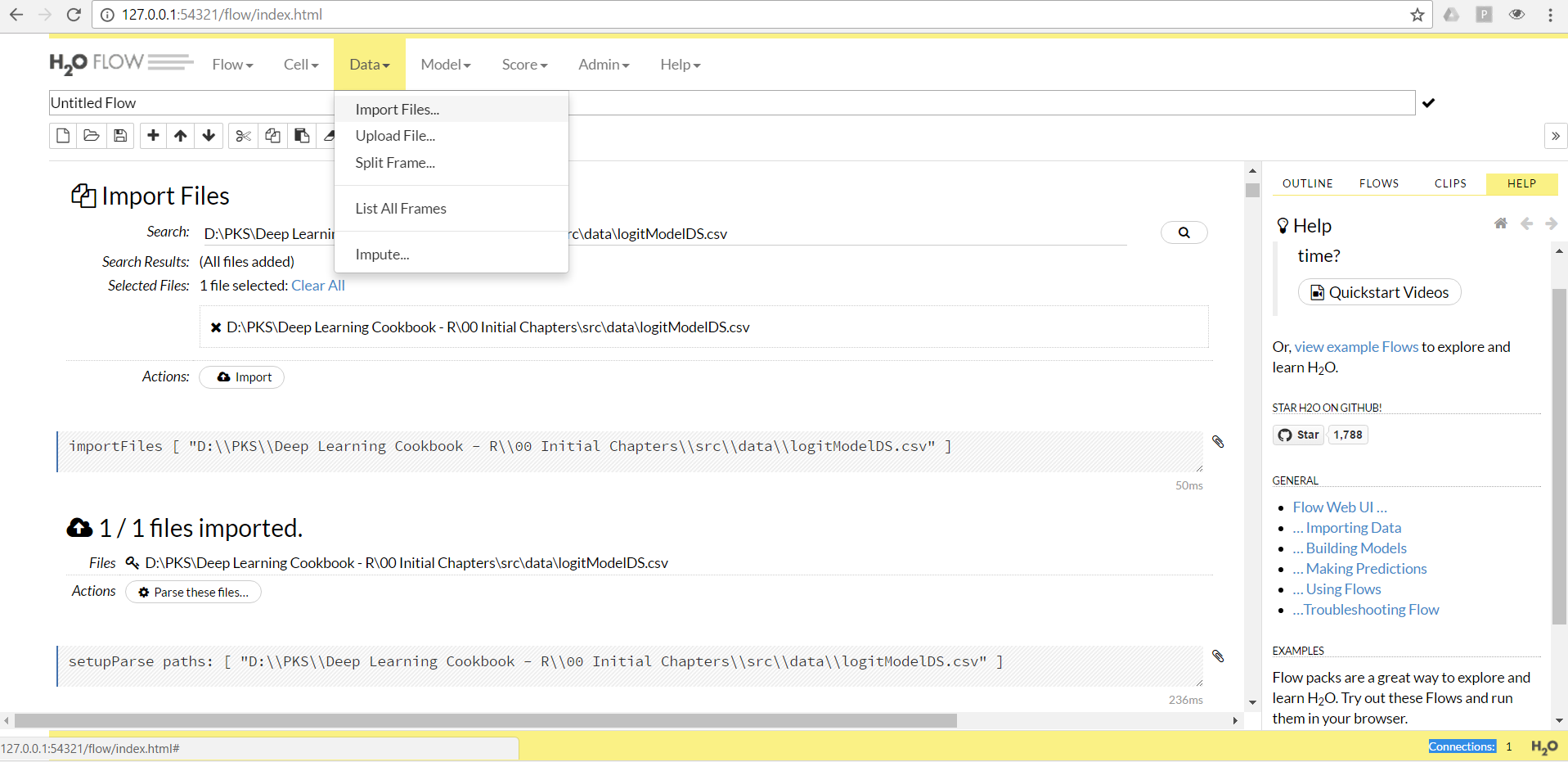
- The imported file in H2O can be parsed into the hex format (the native file format for H2O) using the Parse these files action, which will appear once the file is imported to the H2O environment:
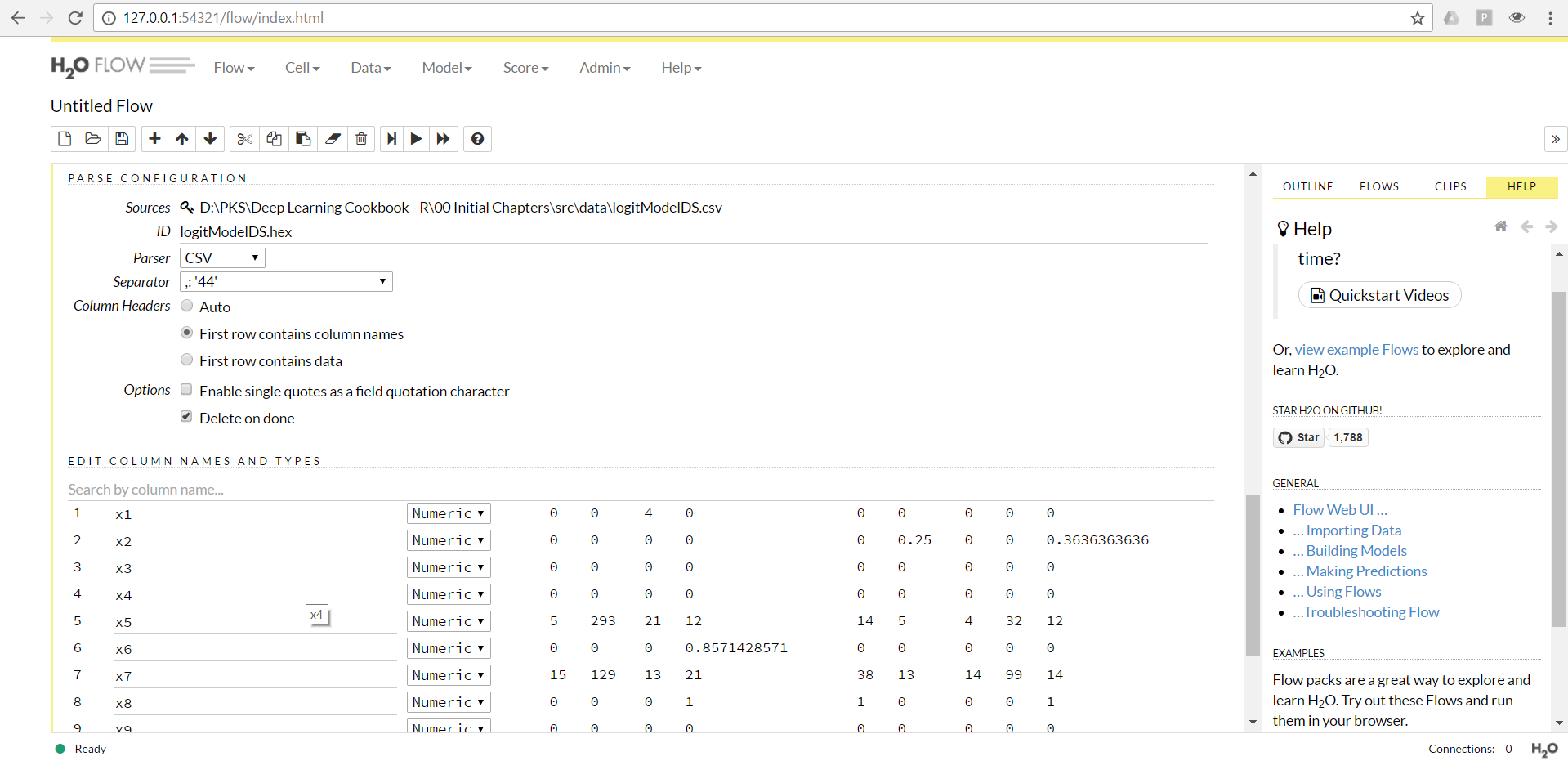
- The parsed data frame in H2O can be split into training and validation using the Data | Split Frame action, as shown in the following screenshot:
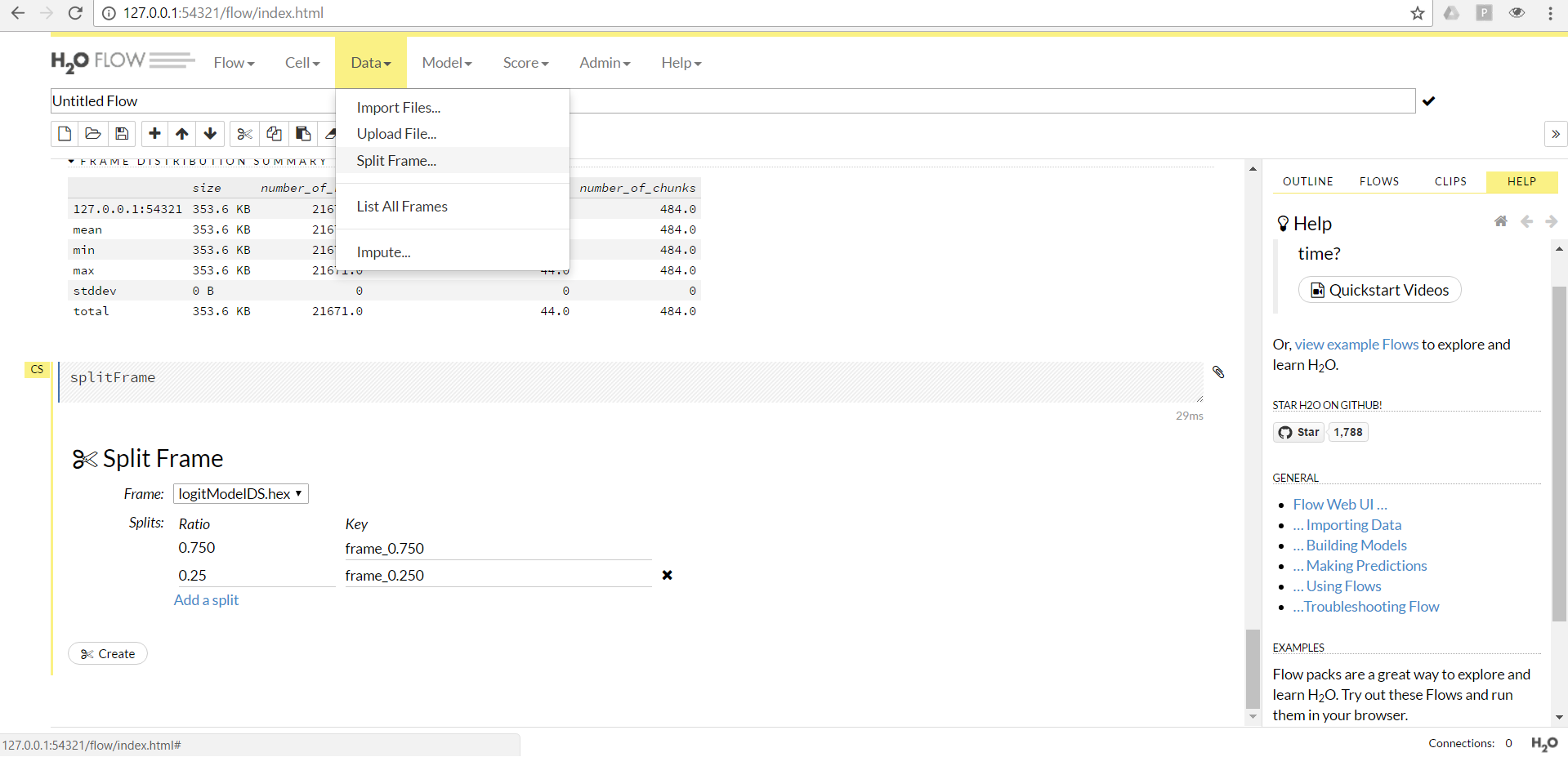
- Select the model from the Model menu and set up the model-related parameters. An example for a glm model is seen in the following screenshot:
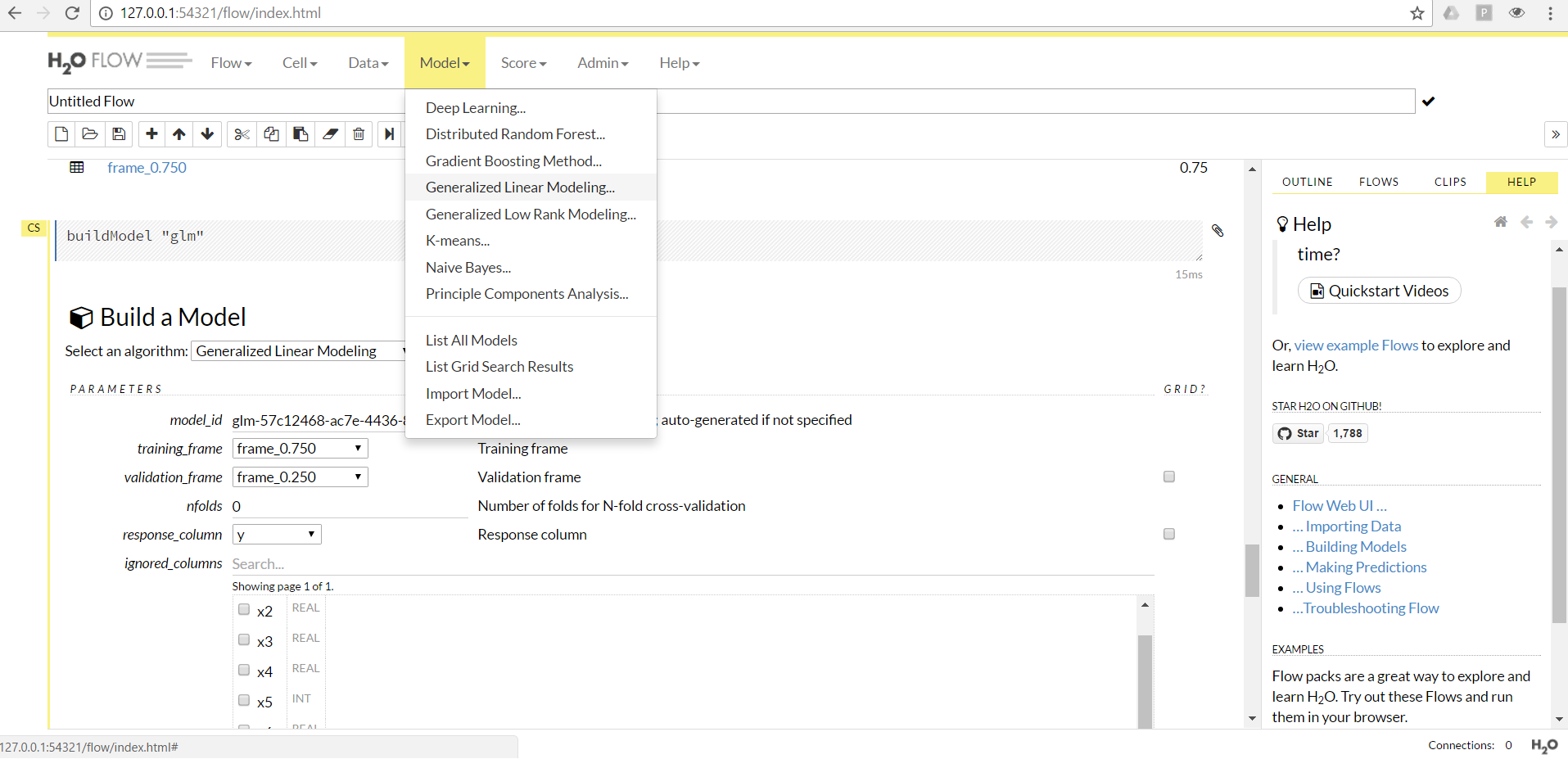
- The Score | predict action can be used to score another hex data frame in H2O:
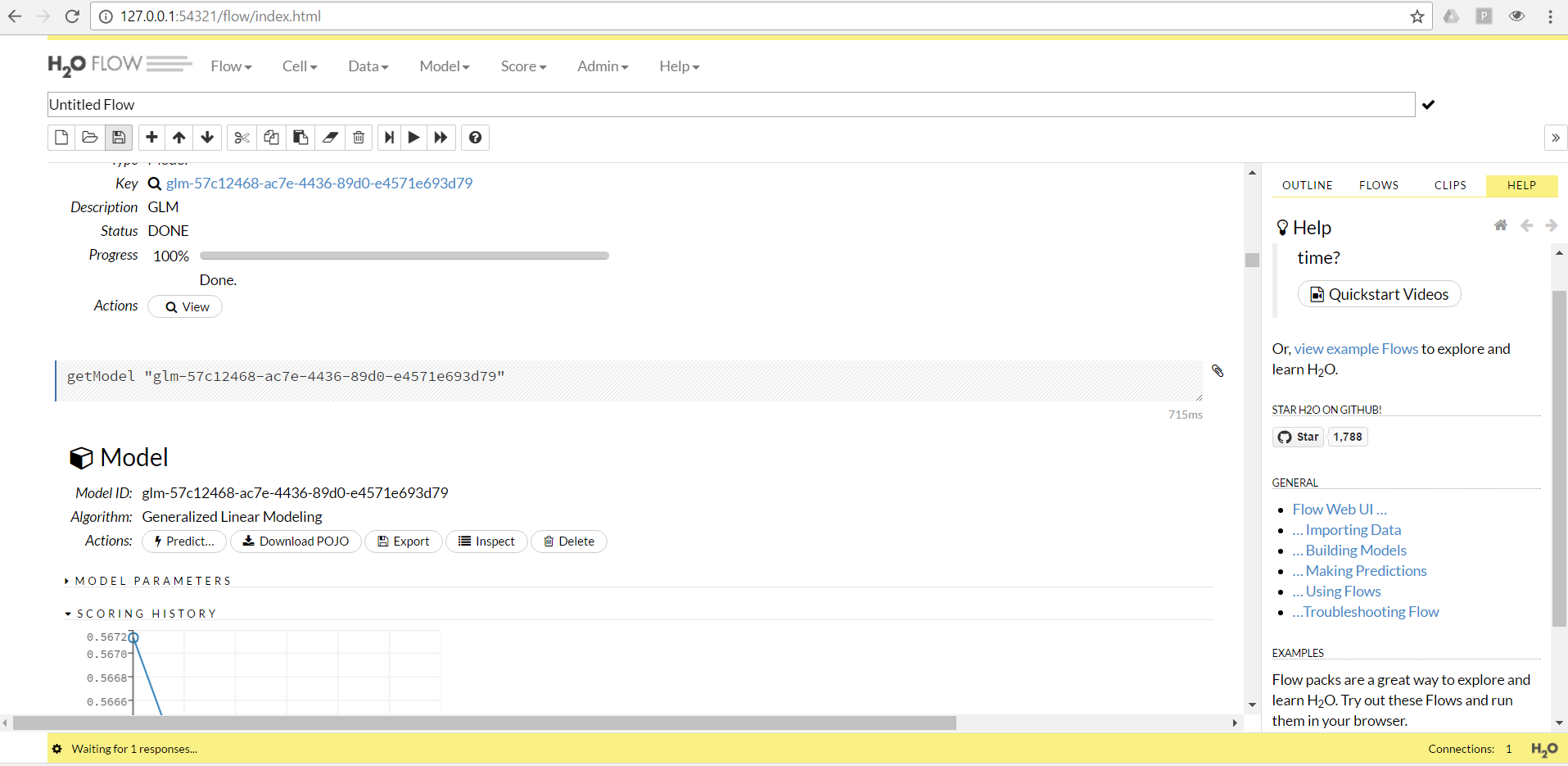
There's more...
For more complicated scenarios that involve a lot of preprocessing, H2O can be called from R directly. This book will focus more on building models using H2O from R directly. If H2O is set up at a different location instead of localhost, then it can be connected within R by defining the correct ip and port at which the cluster is running:
localH2O = h2o.init(ip = "localhost", port = 54321, nthreads = -1)
Another critical parameter is the number of threads to be used to build the model; by default, n threads are set to -2, which means that two cores will be used. The value of -1 for n threads will make use of all available cores.