Data sources
Data source is a term for all the technology related to the extraction and storage of data. A data source can be anything from a simple text file to a big database. The raw data can come from observation logs, sensors, transactions, or user behavior.
A dataset is a collection of data, usually presented in a tabular form. Each column represents a particular attribute, and each row corresponds to a given member of the data, as is showed in the following screenshot.
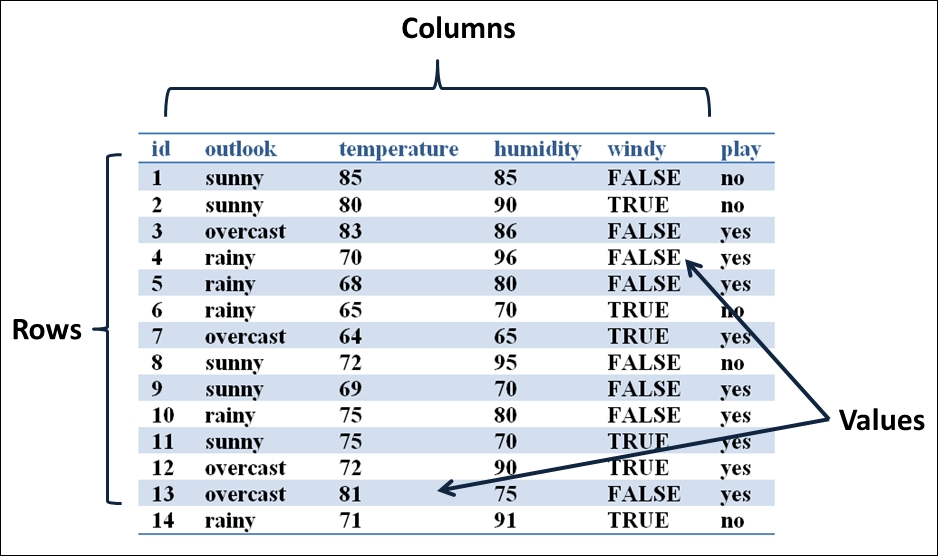
In this section, we will take a look at the most common forms for data sources and datasets.
Tip
The data in the preceding screenshot is from the classical Weather dataset of the UC Irvine Machine Learning Repository:
A dataset represents a logical implementation of a data source; the common features of a dataset:
- Dataset characteristics (multivariate and univariate)
- Number of instances
- Area (life, business, and many more)
- Attribute characteristics (real, categorical, and nominal)
- Number of...