What are support vector machines?
Support vector machines (SVM) are supervised learning models that are very popular in the realm of machine learning. SVMs are really good at analyzing labeled data and detecting patterns. Given a bunch of data points and the associated labels, SVMs will build the separating hyperplanes in the best possible way.
Wait a minute, what are hyperplanes? To understand that, let's consider the following figure:
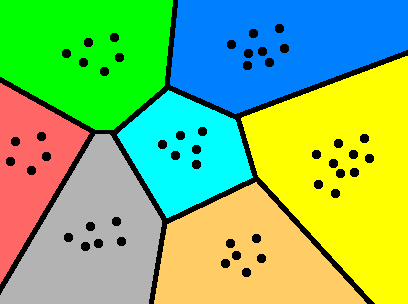
As you can see, the points are being separated by line boundaries that are equidistant from the points. This is easy to visualize in two dimensions. If it were in three dimensions, the separators would be planes. When we build features for images, the length of the feature vectors is usually in the six-digit range. So, when we go to such a high dimensional space, the equivalent of lines would be hyperplanes. Once the hyperplanes are formulated, we use this mathematical model to classify unknown data, based on where it falls on this map.