Temporal difference learning
Temporal Difference (TD) learning is the central and novel theme of reinforcement learning. TD learning is the combination of both Monte Carlo (MC) and Dynamic Programming (DP) ideas. Like Monte Carlo methods, TD methods can learn directly from the experiences without the model of the environment. Similar to Dynamic Programming, TD methods update estimates based in part on other learned estimates, without waiting for a final outcome, unlike MC methods, in which estimates are updated after reaching the final outcome only.
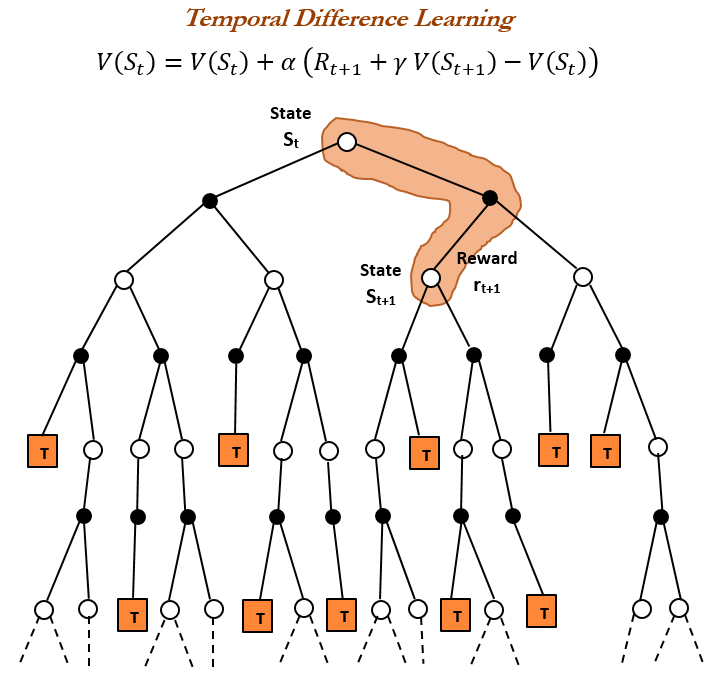
TD prediction
Both TD and MC use experience to solve z prediction problem. Given some policy π, both methods update their estimate v of vπ for the non-terminal states St occurring in that experience. Monte Carlo methods wait until the return following the visit is known, then use that return as a target for V(St).

The preceding method can be called as a constant - α MC, where MC must wait until the end of the episode to determine the increment...