Calculating error rates
To calculate the error rates on classification algorithms, we'll keep count of several things. We'll track how many positives are correctly and incorrectly identified as well as how many negatives are correctly and incorrectly identified. These values are usually called true positives, false positives, true negatives, and false negatives. The relationship of these values to the expected values and the classifier's outputs and to each other can be seen in the following diagram:
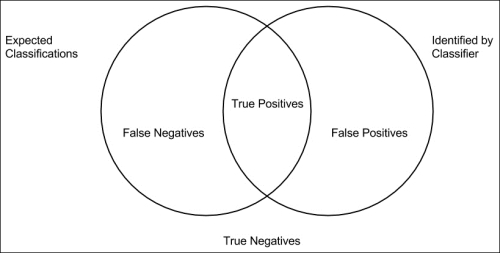
From these numbers, we'll first calculate the precision of the algorithm. This is the ratio of true positives to the number of all identified positives (both true and false positives). This tells us how many of the items that it identified as positives actually are positives.
We'll then calculate the recall. This is the ratio of true positives to all actual positives (true positives and false negatives). This gives us an idea of how many positives it's missing.
To calculate this, we'll use a standard...