Logistic Regression
The logistic or logit model is one such non-linear model that has been effectively used for classification tasks in a number of different domains. In this section, we will use it to classify images of hand-written digits. In understanding the logistic model, we also take an important step in understanding the operation of a particularly powerful machine learning model, artificial neural networks. So, what exactly is the logistic model? Like the linear model, which is composed of a linear or straight-line function, the logistic model is composed of the standard logistic function, which, in mathematical terms, looks something like this:
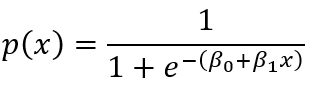
Figure 4.8: Logistic function
In practical terms, when trained, this function returns the probability of the input information belonging to a particular class or group.
Say we would like to predict whether a single entry of data belongs to one of two groups. As in the previous example, in linear regression, this would equate to y being either...