



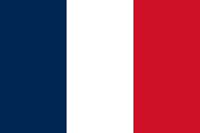


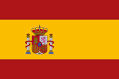



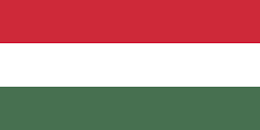

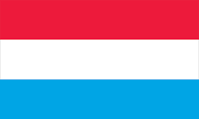





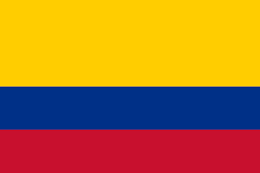
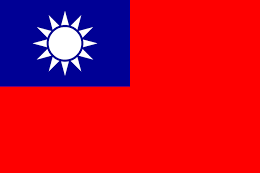
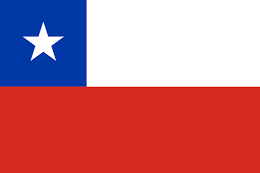



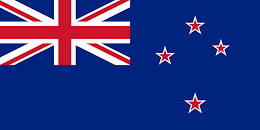
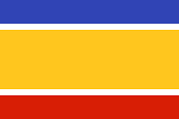
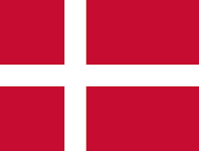
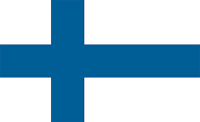







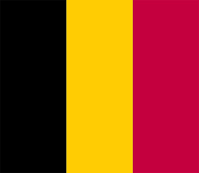

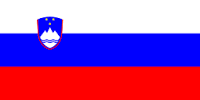





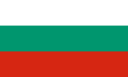


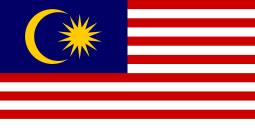



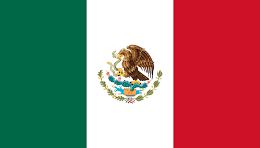

According to a study conducted by researchers at Stanford University School of Medicine and Unanimous AI, small groups of radiologists moderated by AI algorithms achieve higher diagnostic accuracy against individual radiologists or the machine learning algorithms alone.
The technology used for research is called Swarm AI. Swarm AI is a swarm intelligence technology by Unanimous AI that empowers networked groups of humans by combining their individual insights in real-time with the help of AI algorithms to converge on optimal solutions.
The research paper was presented on earlier this week at the SIIM Conference on Machine Intelligence in Medical Imaging.
The researchers performed the study with a group of eight radiologists at different locations, connected by Swarm AI algorithms. The radiologists reviewed a set of 50 chest x-rays and for each of the X-ray predicted the likelihood that the patient has pneumonia.
After a few seconds of individually assessing the results of the chest x-rays, the group worked together as a “Swarm”, converging on a probabilistic diagnosis to predict the likelihood of a patient having pneumonia. This generated a set of 50 probabilities for the 50 test cases.
At the same time, separately, the same set of 50 chest x-rays were run through CheXNet software algorithm, a state-of-the-art 121-layer convolutional neural network, that beat humans last year in predicting which patient suffering from pneumonia. CheXNet has been proved to outperform individual human radiologists in pneumonia screening tasks as per prior studies.
These two sets of probabilities were then, further compared using different statistical techniques.
The performance of the Swarm AI system involving a small group of human radiologists was evaluated against the software-only CheXNet system. These two methods were analyzed across three different performance metrics, namely, binary classification accuracy, Mean Absolute Error, and ROC analysis. Let’s see how these two methods performed.
Swarm AI system produced far more accurate results in the diagnosis of pneumonia than a state-of-the-art ML system, like CheXNet.
“Diagnosing pathologies like pneumonia from chest X-rays is extremely difficult, making it an ideal target for AI technologies. The results of this study are very exciting as they point towards a future where doctors and AI algorithms can work together in real-time, rather than human practitioners being replaced by automated algorithms,” says Dr. Matthew Lungren, Assistant Professor of Radiology at Stanford University, in the Unanimous AI blog.
This suggests that Swarm algorithms are a powerful tool when it comes to establishing Ground Truth for training use as well as for validating the machine learning systems.
“It is likely that the Swarm AI system excels in certain types of cases, while the ML system excels in others. We believe future research should identify these differences, so each method can be applied to those cases which are most appropriate. Additional research is warranted using more definitive Ground Truth and a wider range of cases,” write researchers in the paper.
For more information, check out the official research paper.
MIT’s Transparency by Design Network: A high performant model that uses visual reasoning for machine interpretability
A new Video-to-Video Synthesis model uses Artificial Intelligence to create photorealistic videos
Survey reveals how artificial intelligence is impacting developers across the tech landscape