We ended Chapter 8, Reinforcement Learning Theory, with an example of an agent learning to play the cart-pole game with the help of Q-learning and a simple network with one hidden layer. The state of the cart-pole environment is described with four numerical variables: cart position and velocity, and pole angle and velocity. We used these variables as an input to the q-function approximation network and successfully trained the agent to prevent the pole from tipping over for more than 200 episode steps. But if it was a human playing the game, he or she would steer the cart based on the screen images he or she sees. That is, if we think of the human as an "agent," the environment "state" he or she would use would be the sequence of frames displayed on the screen. Compare this to just four variables our artificial agent used, and you'll see...




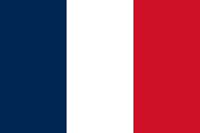


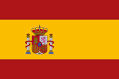



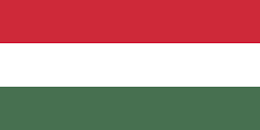

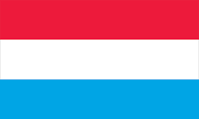





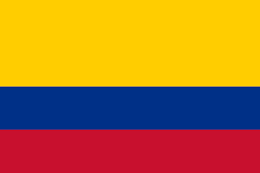
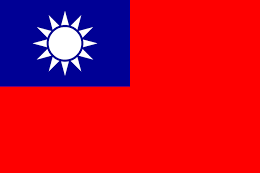
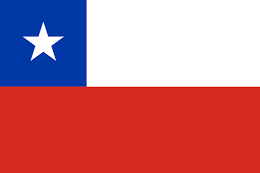



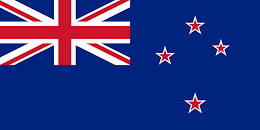
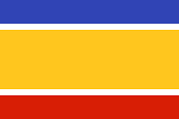
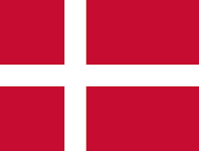
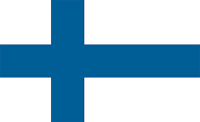







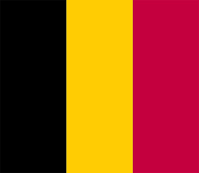

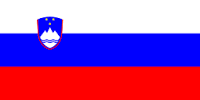





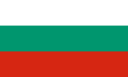


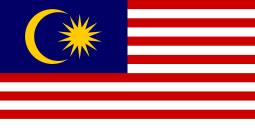



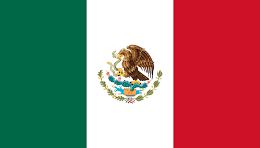
