To help understand the differences between tidy and messy data, let's take a look at a simple table that may or may not be in tidy form:
>>> state_fruit = pd.read_csv('data/state_fruit.csv', index_col=0)
>>> state_fruit
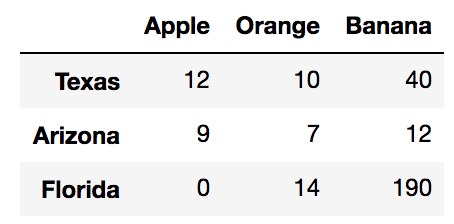
There does not appear to be anything messy about this table, and the information is easily consumable. However, according to the tidy principles, it isn't actually tidy. Each column name is actually the value of a variable. In fact, none of the variable names are even present in the DataFrame. One of the first steps to transform a messy dataset into tidy data is to identify all of the variables. In this particular dataset, we have variables for state and fruit. There's also the numeric data that wasn't identified anywhere in the context of the problem. We can label...