Let's suppose we consider a multi-class classification problem where the conditional probability for a sample xi ∈ ℜm to belong to the yj class can be modeled as a multivariate Gaussian distribution (X is assumed to be made up of independent and identically distributed (i.i.d) variables with extremely low collinearities):

In this case, the class j is fully determined by the mean vector μj and the covariance matrix Σj. If we apply the Bayes' theorem, we can obtain the posterior probability p(yj|xi):
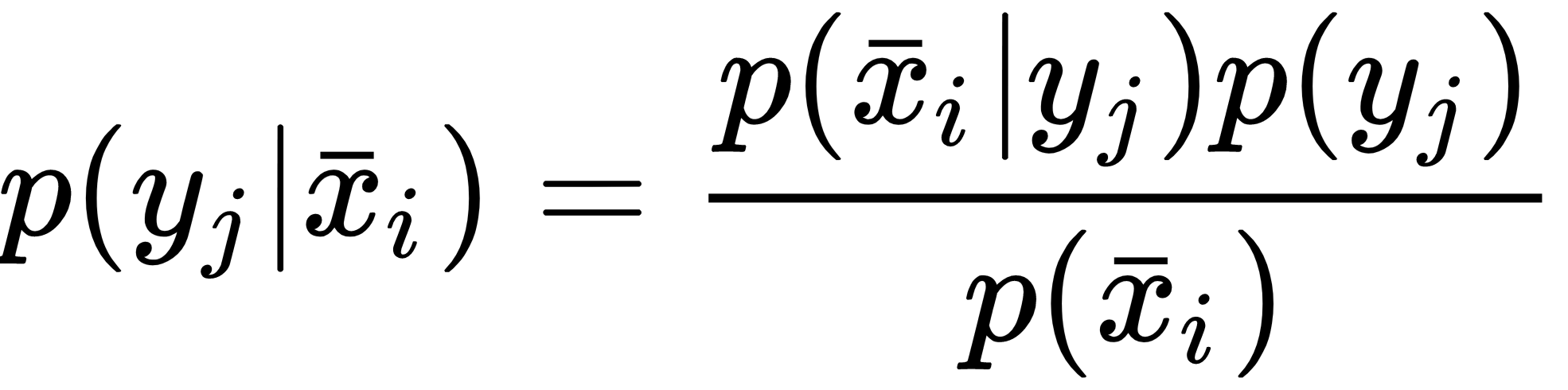
Considering the discussion of Gaussian Naive Bayes, it's not difficult to understand how it's possible to estimate μj and Σj using the training set, in fact, they correspond to the sample mean and covariance and can be easily computed in closed form.
Now, for simplicity, let's consider a binary problem...