In this chapter, you've learned how to process big data to create an analytics-ready dataset. You've also seen how SageMaker automates most of the steps of the machine learning life cycle, enabling you to build, train, and deploy models seamlessly. Additionally, we've illustrated some of the productivity features, such as hyperparameter optimization and experimentation service, which enable data scientists to run multiple experiments and deploy the winning model. Finally, we have looked at bringing our own models and containers to the SageMaker ecosystem. Through bringing our own models based on open source machine learning libraries, we can readily build solutions based on open source frameworks, while still leveraging all of the capabilities of the platform. Similarly, by bringing our own container, we can readily port solutions, written in other programming...





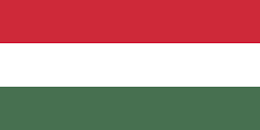

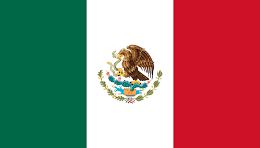


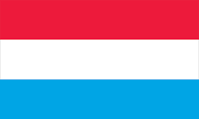



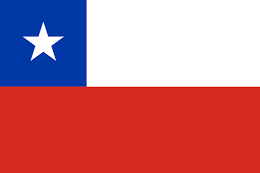



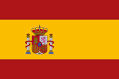


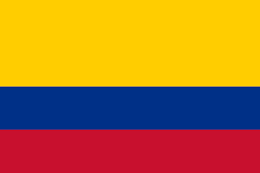
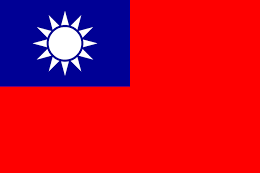


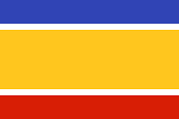
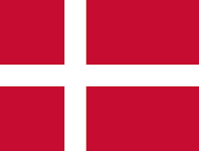
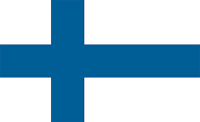



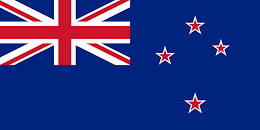


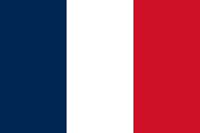



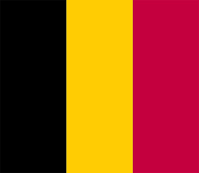

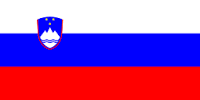




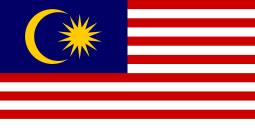


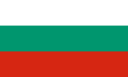



