1. Policy gradient theorem
As discussed in Chapter 9, Deep Reinforcement Learning, the agent is situated in an environment that is in state st, an element of state space, . The state space
may be discrete or continuous. The agent takes an action
from the action space
by obeying the policy,
.
may be discrete or continuous. As a result of executing the action
, the agent receives a reward rt+1 and the environment transitions to a new state, st+1. The new state is dependent only on the current state and action. The goal of the agent is to learn an optimal policy
that maximizes the return from all states:

The return, Rt, is defined as the discounted cumulative reward from time t until the end of the episode or when the terminal state is reached:
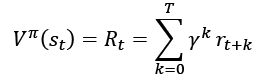
From Equation 9.1.2, the return can also be interpreted as a value of a given state by following the policy . It can be observed from Equation 9.1.1 that future rewards ...