The key idea to tackle this problem is to have a controller network which proposes a child model architecture with probability p, given a particular network given in input. The child is trained and evaluated for the particular task to be solved (say for instance that the child gets accuracy R). This evaluation R is passed back to the controller which, in turn, uses R to improve the next candidate architecture. Given this framework, it is possible to model the feedback from the candidate child to the controller as the task of computing the gradient of p and then scale this gradient by R. The controller can be implemented as a Recurrent Neural Network (see the following figure). In doing so, the controller will tend to privilege iteration after iterations candidate areas of architecture that achieve better R and...




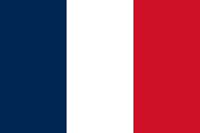


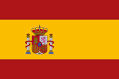



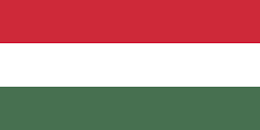

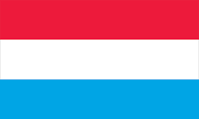





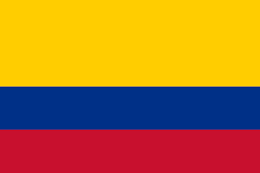
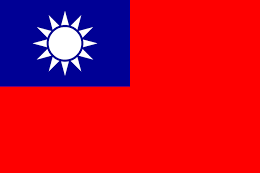
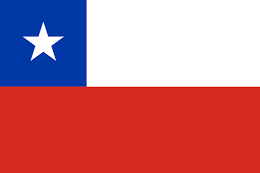



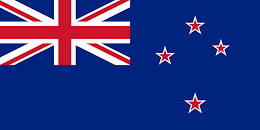
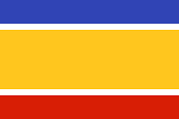
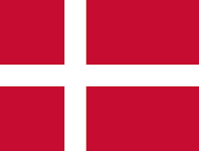
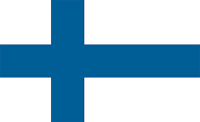







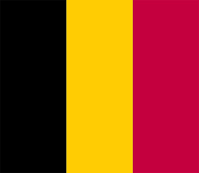

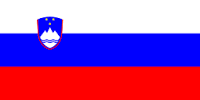





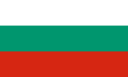


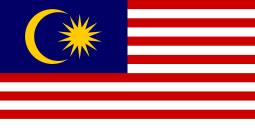



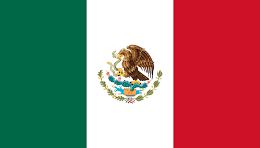
