Spectral clustering
One of the most common problems with K-means and other similar algorithms is the assumption that we only have hyperspherical clusters. In fact, K-means is insensitive to the angle and assigns a label only according to the closest distance between a point and centroids. The resulting geometry is based on hyperspheres where all points share the same condition to be closer to the same centroid. This condition might be acceptable when the dataset is split into blobs that can be easily embedded into a regular geometric structure. However, it fails whenever the sets are not separable using regular shapes. Let's consider, for example, the following bidimensional dataset:
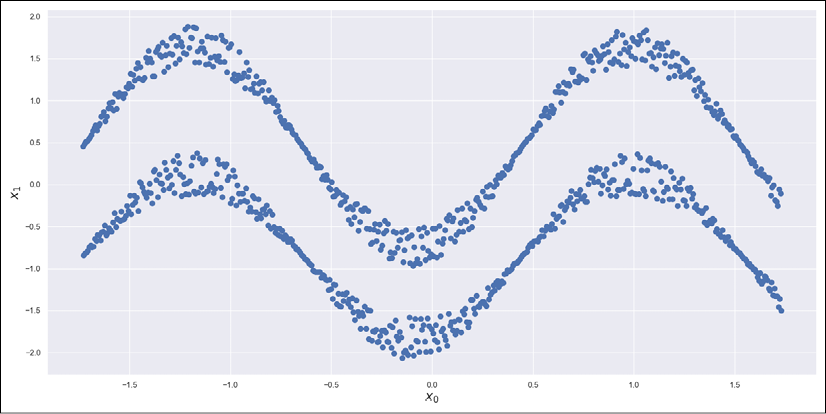
Sinusoidal dataset
As we are going to see in the example, any attempt to separate the upper sinusoid from the lower one using K-means will fail. The reason is obvious: a circle that contains the upper set will also contain part of the (or the whole) lower set. Considering the criterion...