In this chapter, firstly, we used convolutional layers that are capable of decomposing a given visual input space into hierarchically nested probabilistic activations of convolution filters that subsequently connect to dense neurons that perform classification. The filters in these convolutional layers learn weights corresponding to useful representations that may be queried in a probabilistic manner to map the set of input features present in a dataset to the respective output classes. Furthermore, we saw how we can dive deep into our convolution network to understand what it has learned. We saw four specific ways to do this: intermediate activation-based, saliency-based, gradient weighted class activations, and activation maximization visualizations. Each gives a unique intuition into which patterns are picked up by the different layers of our network. We visualized...




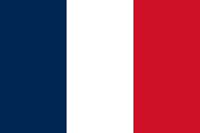


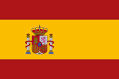



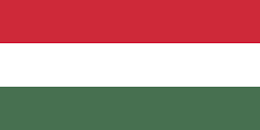

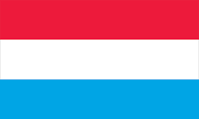





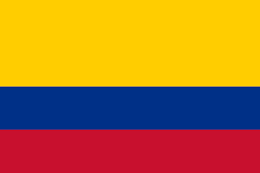
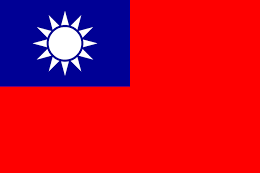
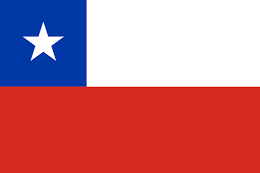



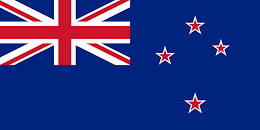
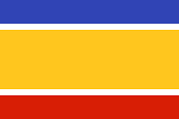
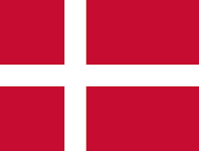
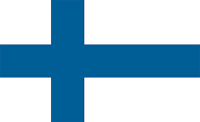







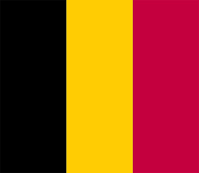

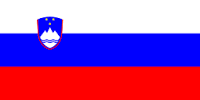





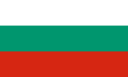


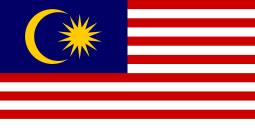



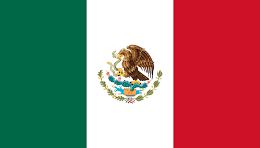
