



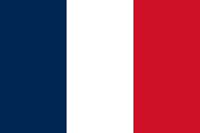


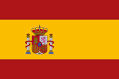



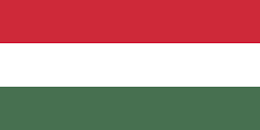

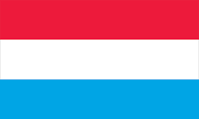





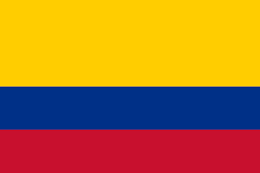
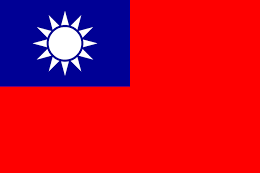
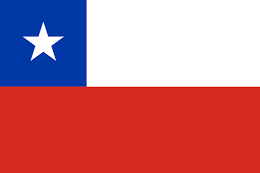



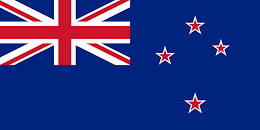
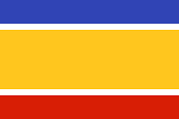
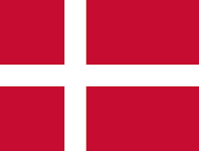
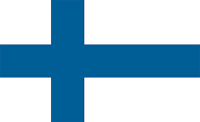







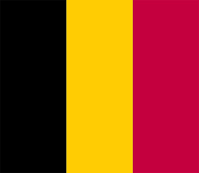

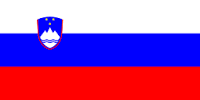





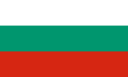


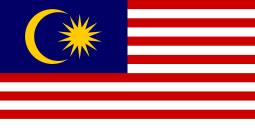



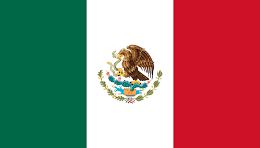

A team of researchers from Institute of Cancer Research London (ICR) and the University of Edinburgh have devised a method named repeated evolution in cancer, also known as REVOLVER. It uses a machine learning approach, specifically known as transfer learning to find out patterns in DNA mutation within cancer and uses the information to forecast future genetic changes.
REVOLVER exploits multiple independent noisy observations taken from single patients and transfers information between patients to de-noise data and highlight hidden evolutionary patterns. Along with explaining the data in each patient, the individual models also highlight subgroups of tumors that evolved similarly
The goal of this model is to solve the biggest challenge in oncology, that is, the tumor with time could progress from benign to malignant, become metastatic, and develop resistance to certain therapies. This occurs through a process of clonal evolution that involves cancer cells and their microenvironment, and results in intratumor heterogeneity (ITH). ITH results to the deadly outcome of cancer by providing the substrate of phenotypic variation on which adaptation can occur.
To accurately detect and compare changes in each tumour, the team used 768 tumour samples from 178 patients reported in previous studies for lung, breast, kidney and bowel cancer, and analysed the data within each cancer type respectively.
Dr Andrea Sottoriva, a team leader in evolutionary genomics and modelling at the ICR who was a part of this study, believes that this AI tool could help the doctors find a treatment in an earlier stage:
"By giving us a peek into the future, we could potentially use this AI tool to intervene at an earlier stage, predicting cancer's next move."
To explore more on REVOLVER method, check out the paper: Detecting repeated cancer evolution from multi-region tumor sequencing data.
Google, Harvard researchers build a deep learning model to forecast earthquake aftershocks location with over 80% accuracy
8 Machine learning best practices
How everyone at Netflix uses Jupyter notebooks from data scientists, machine learning engineers, to data analysts