Fine-tuning BERT
This section will fine-tune a BERT model to predict the downstream task of Acceptability Judgments and measure the predictions with the Matthews Correlation Coefficient (MCC), which will be explained in the Evaluating using Matthews Correlation Coefficient section of this chapter.
Open BERT_Fine_Tuning_Sentence_Classification_GPU.ipynb
in Google Colab (make sure you have a Gmail account). The notebook is in Chapter03
in the GitHub repository of this book.
The title of each cell in the notebook is also the same as or very close to the title of each subsection of this chapter.
We will first examine why transformer models must take hardware constraints into account.
Hardware constraints
Transformer models require multiprocessing hardware. Go to the Runtime menu in Google Colab, select Change runtime type, and select GPU in the Hardware Accelerator drop-down list.
Transformer models are hardware-driven. I recommend reading Appendix II, Hardware Constraints for Transformer Models, before continuing this chapter.
The program will be using Hugging Face
modules, which we’ll install next.
Installing the Hugging Face PyTorch interface for BERT
Hugging Face provides a pretrained BERT model. Hugging Face developed a base class named PreTrainedModel
. By installing this class, we can load a model from a pretrained model configuration.
Hugging Face provides modules in TensorFlow and PyTorch. I recommend that a developer be comfortable with both environments. Excellent AI research teams use either or both environments.
In this chapter, we will install the modules required as follows:
#@title Installing the Hugging Face PyTorch Interface for Bert
!pip install -q transformers
The installation will run, or requirement satisfied messages will be displayed.
We can now import the modules needed for the program.
Importing the modules
We will import the pretrained modules required, such as the pretrained BERT tokenizer
and the configuration of the BERT model. The BERTAdam
optimizer is imported along with the sequence classification module:
#@title Importing the modules
import torch
import torch.nn as nn
from torch.utils.data import TensorDataset, DataLoader, RandomSampler, SequentialSampler
from sklearn.model_selection import train_test_split
from transformers import BertTokenizer, BertConfig
from transformers import AdamW, BertForSequenceClassification, get_linear_schedule_with_warmup
A nice progress bar module is imported from tqdm
:
from tqdm import tqdm, trange
We can now import the widely used standard Python modules:
import pandas as pd
import io
import numpy as np
import matplotlib.pyplot as plt
If all goes well, no message will be displayed, bearing in mind that Google Colab has pre-installed the modules on the VM we are using.
Specifying CUDA as the device for torch
We will now specify that torch uses the Compute Unified Device Architecture (CUDA) to put the parallel computing power of the NVIDIA card to work for our multi-head attention model:
#@title Harware verification and device attribution
device = torch.device("cuda" if torch.cuda.is_available() else "cpu")
!nvidia-smi
The output may vary with Google Colab configurations. See Appendix II: Hardware Constraints for Transformer Models for explanations and screenshots.
We will now load the dataset.
Loading the dataset
We will now load the CoLA based on the Warstadt et al. (2018) paper.
General Language Understanding Evaluation (GLUE) considers Linguistic Acceptability as a top-priority NLP task. In Chapter 5, Downstream NLP Tasks with Transformers, we will explore the key tasks transformers must perform to prove their efficiency.
The following cells in the notebook automatically download the necessary files:
import os
!curl -L https://raw.githubusercontent.com/Denis2054/Transformers-for-NLP-2nd-Edition/master/Chapter03/in_domain_train.tsv --output "in_domain_train.tsv"
!curl -L https://raw.githubusercontent.com/Denis2054/Transformers-for-NLP-2nd-Edition/master/Chapter03/out_of_domain_dev.tsv --output "out_of_domain_dev.tsv"
You should see them appear in the file manager:
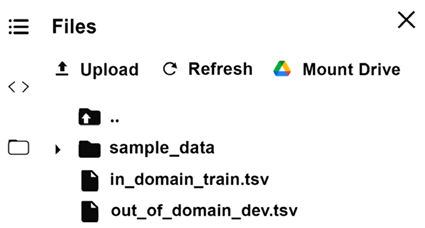
Figure 3.5: Uploading the datasets
Now the program will load the datasets:
#@title Loading the Dataset
#source of dataset : https://nyu-mll.github.io/CoLA/
df = pd.read_csv("in_domain_train.tsv", delimiter='\t', header=None, names=['sentence_source', 'label', 'label_notes', 'sentence'])
df.shape
The output displays the shape of the dataset we have imported:
(8551, 4)
A 10-line sample is displayed to visualize the Acceptability Judgment task and see if a sequence makes sense or not:
df.sample(10)
The output shows 10
lines of the labeled dataset, which may change after each run:
sentence_source |
label |
label_notes |
sentence |
|
|
|
|
|
|
|
|
|
|
|
|
|
|
|
|
|
|
|
|
|
|
|
|
|
|
|
|
|
|
|
|
|
|
|
|
|
|
|
|
|
|
|
|
|
|
|
|
|
|
|
Each sample in the .tsv
files contains four tab-separated columns:
- Column 1: the source of the sentence (code)
- Column 2: the label (
0
=unacceptable,1
=acceptable) - Column 3: the label annotated by the author
- Column 4: the sentence to be classified
You can open the .tsv
files locally to read a few samples of the dataset. The program will now process the data for the BERT model.
Creating sentences, label lists, and adding BERT tokens
The program will now create the sentences as described in the Preparing the pretraining input environment section of this chapter:
#@ Creating sentence, label lists and adding Bert tokens
sentences = df.sentence.values
# Adding CLS and SEP tokens at the beginning and end of each sentence for BERT
sentences = ["[CLS] " + sentence + " [SEP]" for sentence in sentences]
labels = df.label.values
The [CLS]
and [SEP]
have now been added.
The program now activates the tokenizer.
Activating the BERT tokenizer
In this section, we will initialize a pretrained BERT tokenizer. This will save the time it would take to train it from scratch.
The program selects an uncased tokenizer, activates it, and displays the first tokenized sentence:
#@title Activating the BERT Tokenizer
tokenizer = BertTokenizer.from_pretrained('bert-base-uncased', do_lower_case=True)
tokenized_texts = [tokenizer.tokenize(sent) for sent in sentences]
print ("Tokenize the first sentence:")
print (tokenized_texts[0])
The output contains the classification token and the sequence segmentation token:
Tokenize the first sentence:
['[CLS]', 'our', 'friends', 'wo', 'n', "'", 't', 'buy', 'this', 'analysis', ',', 'let', 'alone', 'the', 'next', 'one', 'we', 'propose', '.', '[SEP]']
The program will now process the data.
Processing the data
We need to determine a fixed maximum length and process the data for the model. The sentences in the datasets are short. But, to make sure of this, the program sets the maximum length of a sequence to 128
, and the sequences are padded:
#@title Processing the data
# Set the maximum sequence length. The longest sequence in our training set is 47, but we'll leave room on the end anyway.
# In the original paper, the authors used a length of 512.
MAX_LEN = 128
# Use the BERT tokenizer to convert the tokens to their index numbers in the BERT vocabulary
input_ids = [tokenizer.convert_tokens_to_ids(x) for x in tokenized_texts]
# Pad our input tokens
input_ids = pad_sequences(input_ids, maxlen=MAX_LEN, dtype="long", truncating="post", padding="post")
The sequences have been processed and now the program creates the attention masks.
Creating attention masks
Now comes a tricky part of the process. We padded the sequences in the previous cell. But we want to prevent the model from performing attention to those padded tokens!
The idea is to apply a mask with a value of 1
for each token, which 0s will follow for padding:
#@title Create attention masks
attention_masks = []
# Create a mask of 1s for each token followed by 0s for padding
for seq in input_ids:
seq_mask = [float(i>0) for i in seq]
attention_masks.append(seq_mask)
The program will now split the data.
Splitting the data into training and validation sets
The program now performs the standard process of splitting the data into training and validation sets:
#@title Splitting data into train and validation sets
# Use train_test_split to split our data into train and validation sets for training
train_inputs, validation_inputs, train_labels, validation_labels = train_test_split(input_ids, labels, random_state=2018, test_size=0.1)
train_masks, validation_masks, _, _ = train_test_split(attention_masks, input_ids,random_state=2018, test_size=0.1)
The data is ready to be trained, but it still needs to be adapted to torch.
Converting all the data into torch tensors
The fine-tuning model uses torch tensors. The program must convert the data into torch tensors:
#@title Converting all the data into torch tensors
# Torch tensors are the required datatype for our model
train_inputs = torch.tensor(train_inputs)
validation_inputs = torch.tensor(validation_inputs)
train_labels = torch.tensor(train_labels)
validation_labels = torch.tensor(validation_labels)
train_masks = torch.tensor(train_masks)
validation_masks = torch.tensor(validation_masks)
The conversion is over. Now we need to create an iterator.
Selecting a batch size and creating an iterator
In this cell, the program selects a batch size and creates an iterator. The iterator is a clever way of avoiding a loop that would load all the data in memory. The iterator, coupled with the torch DataLoader
, can batch train massive datasets without crashing the machine’s memory.
In this model, the batch size is 32
:
#@title Selecting a Batch Size and Creating and Iterator
# Select a batch size for training. For fine-tuning BERT on a specific task, the authors recommend a batch size of 16 or 32
batch_size = 32
# Create an iterator of our data with torch DataLoader. This helps save on memory during training because, unlike a for loop,
# with an iterator the entire dataset does not need to be loaded into memory
train_data = TensorDataset(train_inputs, train_masks, train_labels)
train_sampler = RandomSampler(train_data)
train_dataloader = DataLoader(train_data, sampler=train_sampler, batch_size=batch_size)
validation_data = TensorDataset(validation_inputs, validation_masks, validation_labels)
validation_sampler = SequentialSampler(validation_data)
validation_dataloader = DataLoader(validation_data, sampler=validation_sampler, batch_size=batch_size)
The data has been processed and is all set. The program can now load and configure the BERT model.
BERT model configuration
The program now initializes a BERT uncased configuration:
#@title BERT Model Configuration
# Initializing a BERT bert-base-uncased style configuration
#@title Transformer Installation
try:
import transformers
except:
print("Installing transformers")
!pip -qq install transformers
from transformers import BertModel, BertConfig
configuration = BertConfig()
# Initializing a model from the bert-base-uncased style configuration
model = BertModel(configuration)
# Accessing the model configuration
configuration = model.config
print(configuration)
The output displays the main Hugging Face parameters similar to the following (the library is often updated):
BertConfig {
"attention_probs_dropout_prob": 0.1,
"hidden_act": "gelu",
"hidden_dropout_prob": 0.1,
"hidden_size": 768,
"initializer_range": 0.02,
"intermediate_size": 3072,
"layer_norm_eps": 1e-12,
"max_position_embeddings": 512,
"model_type": "bert",
"num_attention_heads": 12,
"num_hidden_layers": 12,
"pad_token_id": 0,
"type_vocab_size": 2,
"vocab_size": 30522
}
Let’s go through these main parameters:
attention_probs_dropout_prob
:0.1
applies a0.1
dropout ratio to the attention probabilities.hidden_act
:"gelu"
is a non-linear activation function in the encoder. It is a Gaussian Error Linear Units activation function. The input is weighted by its magnitude, which makes it non-linear.hidden_dropout_prob
:0.1
is the dropout probability applied to the fully connected layers. Full connections can be found in the embeddings, encoder, and pooler layers. The output is not always a good reflection of the content of a sequence. Pooling the sequence of hidden states improves the output sequence.hidden_size
:768
is the dimension of the encoded layers and also the pooler layer.initializer_range
:0.02
is the standard deviation value when initializing the weight matrices.intermediate_size
:3072
is the dimension of the feed-forward layer of the encoder.layer_norm_eps
:1e-12
is the epsilon value for layer normalization layers.max_position_embeddings
:512
is the maximum length the model uses.model_type
:"bert"
is the name of the model.num_attention_heads
:12
is the number of heads.num_hidden_layers
:12
is the number of layers.pad_token_id
:0
is the ID of the padding token to avoid training padding tokens.type_vocab_size
:2
is the size of thetoken_type_ids
, which identify the sequences. For example, “thedog[SEP]
Thecat.[SEP]
" can be represented with token IDs[0,0,0, 1,1,1]
.vocab_size
:30522
is the number of different tokens used by the model to represent theinput_ids
.
With these parameters in mind, we can load the pretrained model.
Loading the Hugging Face BERT uncased base model
The program now loads the pretrained BERT model:
#@title Loading the Hugging Face Bert uncased base model
model = BertForSequenceClassification.from_pretrained("bert-base-uncased", num_labels=2)
model = nn.DataParallel(model)
model.to(device)
We have defined the model, defined parallel processing, and sent the model to the device. For more explanations, see Appendix II, Hardware Constraints for Transformer Models.
This pretrained model can be trained further if necessary. It is interesting to explore the architecture in detail to visualize the parameters of each sublayer, as shown in the following excerpt:
BertForSequenceClassification(
(bert): BertModel(
(embeddings): BertEmbeddings(
(word_embeddings): Embedding(30522, 768, padding_idx=0)
(position_embeddings): Embedding(512, 768)
(token_type_embeddings): Embedding(2, 768)
(LayerNorm): BertLayerNorm()
(dropout): Dropout(p=0.1, inplace=False)
)
(encoder): BertEncoder(
(layer): ModuleList(
(0): BertLayer(
(attention): BertAttention(
(self): BertSelfAttention(
(query): Linear(in_features=768, out_features=768, bias=True)
(key): Linear(in_features=768, out_features=768, bias=True)
(value): Linear(in_features=768, out_features=768, bias=True)
(dropout): Dropout(p=0.1, inplace=False)
)
(output): BertSelfOutput(
(dense): Linear(in_features=768, out_features=768, bias=True)
(LayerNorm): BertLayerNorm()
(dropout): Dropout(p=0.1, inplace=False)
)
)
(intermediate): BertIntermediate(
(dense): Linear(in_features=768, out_features=3072, bias=True)
)
(output): BertOutput(
(dense): Linear(in_features=3072, out_features=768, bias=True)
(LayerNorm): BertLayerNorm()
(dropout): Dropout(p=0.1, inplace=False)
)
)
(1): BertLayer(
(attention): BertAttention(
(self): BertSelfAttention(
(query): Linear(in_features=768, out_features=768, bias=True)
(key): Linear(in_features=768, out_features=768, bias=True)
(value): Linear(in_features=768, out_features=768, bias=True)
(dropout): Dropout(p=0.1, inplace=False)
)
(output): BertSelfOutput(
(dense): Linear(in_features=768, out_features=768, bias=True)
(LayerNorm): BertLayerNorm()
(dropout): Dropout(p=0.1, inplace=False)
)
)
(intermediate): BertIntermediate(
(dense): Linear(in_features=768, out_features=3072, bias=True)
)
(output): BertOutput(
(dense): Linear(in_features=3072, out_features=768, bias=True)
(LayerNorm): BertLayerNorm()
(dropout): Dropout(p=0.1, inplace=False)
)
)
Let’s now go through the main parameters of the optimizer.
Optimizer grouped parameters
The program will now initialize the optimizer for the model’s parameters. Fine-tuning a model begins with initializing the pretrained model parameter values (not their names).
The parameters of the optimizer include a weight decay rate to avoid overfitting, and some parameters are filtered.
The goal is to prepare the model’s parameters for the training loop:
##@title Optimizer Grouped Parameters
#This code is taken from:
# https://github.com/huggingface/transformers/blob/5bfcd0485ece086ebcbed2d008813037968a9e58/examples/run_glue.py#L102
# Don't apply weight decay to any parameters whose names include these tokens.
# (Here, the BERT doesn't have 'gamma' or 'beta' parameters, only 'bias' terms)
param_optimizer = list(model.named_parameters())
no_decay = ['bias', 'LayerNorm.weight']
# Separate the 'weight' parameters from the 'bias' parameters.
# - For the 'weight' parameters, this specifies a 'weight_decay_rate' of 0.01.
# - For the 'bias' parameters, the 'weight_decay_rate' is 0.0.
optimizer_grouped_parameters = [
# Filter for all parameters which *don't* include 'bias', 'gamma', 'beta'.
{'params': [p for n, p in param_optimizer if not any(nd in n for nd in no_decay)],
'weight_decay_rate': 0.1},
# Filter for parameters which *do* include those.
{'params': [p for n, p in param_optimizer if any(nd in n for nd in no_decay)],
'weight_decay_rate': 0.0}
]
# Note - 'optimizer_grouped_parameters' only includes the parameter values, not the names.
The parameters have been prepared and cleaned up. They are ready for the training loop.
The hyperparameters for the training loop
The hyperparameters for the training loop are critical, though they seem innocuous. Adam will activate weight decay and also go through a warm-up phase, for example.
The learning rate (lr
) and warm-up rate (warmup
) should be set to a very small value early in the optimization phase and gradually increase after a certain number of iterations. This avoids large gradients and overshooting the optimization goals.
Some researchers argue that the gradients at the output level of the sub-layers before layer normalization do not require a warm-up rate. Solving this problem requires many experimental runs.
The optimizer is a BERT version of Adam called BertAdam
:
#@title The Hyperparameters for the Training Loop
optimizer = BertAdam(optimizer_grouped_parameters,
lr=2e-5,
warmup=.1)
The program adds an accuracy measurement function to compare the predictions to the labels:
#Creating the Accuracy Measurement Function
# Function to calculate the accuracy of our predictions vs labels
def flat_accuracy(preds, labels):
pred_flat = np.argmax(preds, axis=1).flatten()
labels_flat = labels.flatten()
return np.sum(pred_flat == labels_flat) / len(labels_flat)
The data is ready. The parameters are ready. It’s time to activate the training loop!
The training loop
The training loop follows standard learning processes. The number of epochs is set to 4
, and measurement for loss and accuracy will be plotted. The training loop uses the dataloader
to load and train batches. The training process is measured and evaluated.
The code starts by initializing the train_loss_set
, which will store the loss and accuracy, which will be plotted. It starts training its epochs and runs a standard training loop, as shown in the following excerpt:
#@title The Training Loop
t = []
# Store our loss and accuracy for plotting
train_loss_set = []
# Number of training epochs (authors recommend between 2 and 4)
epochs = 4
# trange is a tqdm wrapper around the normal python range
for _ in trange(epochs, desc="Epoch"):
…./…
tmp_eval_accuracy = flat_accuracy(logits, label_ids)
eval_accuracy += tmp_eval_accuracy
nb_eval_steps += 1
print("Validation Accuracy: {}".format(eval_accuracy/nb_eval_steps))
The output displays the information for each epoch
with the trange
wrapper, for _ in trange(epochs, desc="Epoch")
:
***output***
Epoch: 0%| | 0/4 [00:00<?, ?it/s]
Train loss: 0.5381132976395461
Epoch: 25%|██▌ | 1/4 [07:54<23:43, 474.47s/it]
Validation Accuracy: 0.788966049382716
Train loss: 0.315329696132929
Epoch: 50%|█████ | 2/4 [15:49<15:49, 474.55s/it]
Validation Accuracy: 0.836033950617284
Train loss: 0.1474070605354314
Epoch: 75%|███████▌ | 3/4 [23:43<07:54, 474.53s/it]
Validation Accuracy: 0.814429012345679
Train loss: 0.07655430570461196
Epoch: 100%|██████████| 4/4 [31:38<00:00, 474.58s/it]
Validation Accuracy: 0.810570987654321
Transformer models are evolving very quickly, and deprecation messages and even errors might occur. Hugging Face is no exception to this, and we must update our code accordingly when this happens.
The model is trained. We can now display the training evaluation.
Training evaluation
The loss and accuracy values were stored in train_loss_set
as defined at the beginning of the training loop.
The program now plots the measurements:
#@title Training Evaluation
plt.figure(figsize=(15,8))
plt.title("Training loss")
plt.xlabel("Batch")
plt.ylabel("Loss")
plt.plot(train_loss_set)
plt.show()
The output is a graph that shows that the training process went well and was efficient:
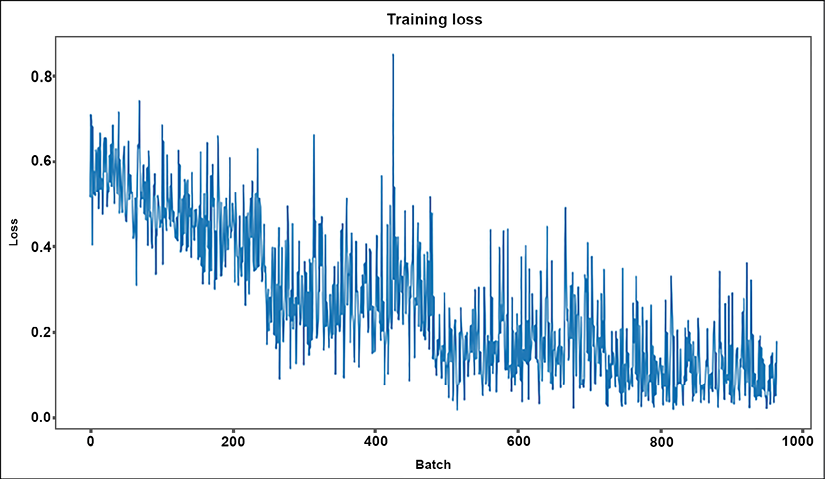
Figure 3.6: Training loss per batch
The model has been fine-tuned. We can now run predictions.
Predicting and evaluating using the holdout dataset
The BERT downstream model was trained with the in_domain_train.tsv
dataset. The program will now make predictions using the holdout (testing) dataset in the out_of_domain_dev.tsv
file. The goal is to predict whether the sentence is grammatically correct.
The following excerpt of the code shows that the data preparation process applied to the training data is repeated in the part of the code for the holdout dataset:
#@title Predicting and Evaluating Using the Holdout Dataset
df = pd.read_csv("out_of_domain_dev.tsv", delimiter='\t', header=None, names=['sentence_source', 'label', 'label_notes', 'sentence'])
# Create sentence and label lists
sentences = df.sentence.values
# We need to add special tokens at the beginning and end of each sentence for BERT to work properly
sentences = ["[CLS] " + sentence + " [SEP]" for sentence in sentences]
labels = df.label.values
tokenized_texts = [tokenizer.tokenize(sent) for sent in sentences]
.../...
The program then runs batch predictions using the dataloader
:
# Predict
for batch in prediction_dataloader:
# Add batch to GPU
batch = tuple(t.to(device) for t in batch)
# Unpack the inputs from our dataloader
b_input_ids, b_input_mask, b_labels = batch
# Telling the model not to compute or store gradients, saving memory and speeding up prediction
with torch.no_grad():
# Forward pass, calculate logit predictions
logits = model(b_input_ids, token_type_ids=None, attention_mask=b_input_mask)
The logits and labels of the predictions are moved to the CPU:
# Move logits and labels to CPU
logits = logits['logits'].detach().cpu().numpy()
label_ids = b_labels.to('cpu').numpy()
The predictions and their true labels are stored:
# Store predictions and true labels
predictions.append(logits)
true_labels.append(label_ids)
The program can now evaluate the predictions.
Evaluating using the Matthews Correlation Coefficient
The Matthews Correlation Coefficient (MCC) was initially designed to measure the quality of binary classifications and can be modified to be a multi-class correlation coefficient. A two-class classification can be made with four probabilities at each prediction:
- TP = True Positive
- TN = True Negative
- FP = False Positive
- FN = False Negative
Brian W. Matthews, a biochemist, designed it in 1975, inspired by his predecessors’ phi function. Since then, it has evolved into various formats such as the following one:
The value produced by MCC is between -1
and +1
. +1
is the maximum positive value of a prediction. -1
is an inverse prediction. 0
is an average random prediction.
GLUE evaluates Linguistic Acceptability with MCC.
MCC is imported from sklearn.metrics
:
#@title Evaluating Using Matthew's Correlation Coefficient
# Import and evaluate each test batch using Matthew's correlation coefficient
from sklearn.metrics import matthews_corrcoef
A set of predictions is created:
matthews_set = []
The MCC value is calculated and stored in matthews_set
:
for i in range(len(true_labels)):
matthews = matthews_corrcoef(true_labels[i],
np.argmax(predictions[i], axis=1).flatten())
matthews_set.append(matthews)
You may see messages due to library and module version changes. The final score will be based on the entire test set, but let’s look at the scores on the individual batches to get a sense of the variability in the metric between batches.
The scores of individual batches
Let’s view the scores of the individual batches:
#@title Score of Individual Batches
matthews_set
The output produces MCC values between -1
and +1
as expected:
[0.049286405809014416,
-0.2548235957188128,
0.4732058754737091,
0.30508307783296046,
0.3567530340063379,
0.8050112948805689,
0.23329882422520506,
0.47519096331149147,
0.4364357804719848,
0.4700159919404217,
0.7679476477883045,
0.8320502943378436,
0.5807564950208268,
0.5897435897435898,
0.38461538461538464,
0.5716350506349809,
0.0]
Almost all the MCC values are positive, which is good news. Let’s see what the evaluation is for the whole dataset.
Matthews evaluation for the whole dataset
The MCC is a practical way to evaluate a classification model.
The program will now aggregate the true values for the whole dataset:
#@title Matthew's Evaluation on the Whole Dataset
# Flatten the predictions and true values for aggregate Matthew's evaluation on the whole dataset
flat_predictions = [item for sublist in predictions for item in sublist]
flat_predictions = np.argmax(flat_predictions, axis=1).flatten()
flat_true_labels = [item for sublist in true_labels for item in sublist]
matthews_corrcoef(flat_true_labels, flat_predictions)
MCC produces a correlation value between –1
and +1
. 0
is an average prediction, -1
is an inverse one, and 1
is perfect. In this case, the output confirms that the MCC is positive, which shows that there is a correlation between this model and dataset:
0.45439842471680725
On this final positive evaluation of the fine-tuning of the BERT model, we have an overall view of the BERT training framework.