Training ML models
Developing an ML model usually requires performing the following essential steps:
- Collecting data.
- Annotating data.
- Designing an ML model.
- Training the model.
- Testing the model.
These steps are depicted in the following diagram:
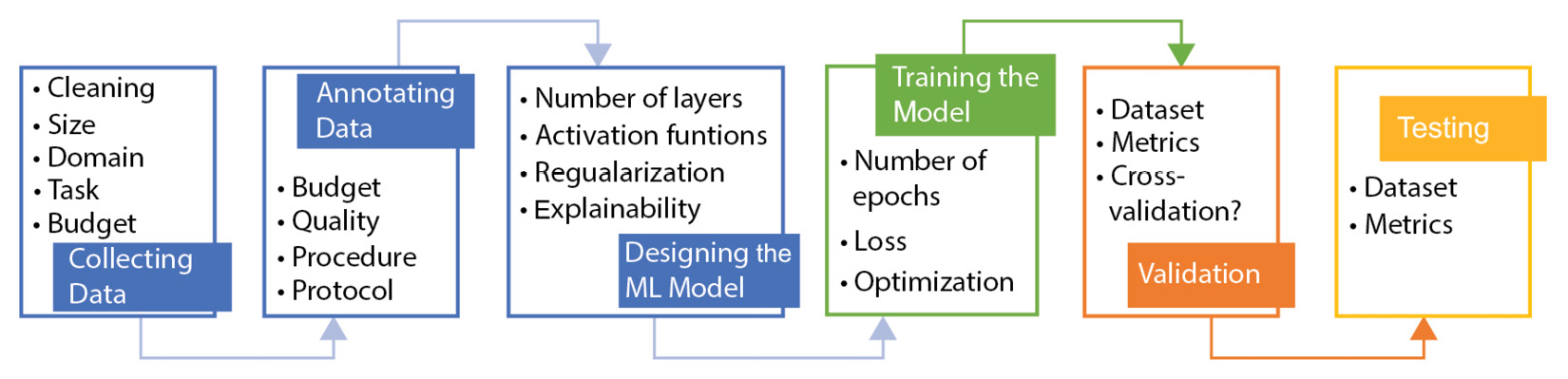
Figure 1.4 – Developing an ML model process
Now, let’s look at each of the steps in more detail to better understand how we can develop an ML model.
Collecting and annotating data
The first step in the process of developing an ML model is collecting the needed training data. You need to decide what training data is needed:
- Train using an existing dataset: In this case, there’s no need to collect training data. Thus, you can skip collecting and annotating data. However, you should make sure that your target task or domain is quite similar to the available dataset(s) you are planning to deploy. Otherwise, your model may train well on this dataset, but it will not perform well when tested on the new task or domain.
- Train on an existing dataset and fine-tune on a new dataset: This is the most popular case in today’s ML. You can pre-train your model on a large existing dataset and then fine-tune it on the new dataset. Regarding the new dataset, it does not need to be very large as you are already leveraging other existing dataset(s). For the dataset to be collected, you need to identify what the model needs to learn and how you are planning to implement this. After collecting the training data, you will begin the annotation process.
- Train from scratch on new data: In some contexts, your task or domain may be far from any available datasets. Thus, you will need to collect large-scale data. Collecting large-scale datasets is not simple. To do this, you need to identify what the model will learn and how you want it to do that. Making any modifications to the plan later may require you to recollect more data or even start the data collection process again from scratch. Following this, you need to decide what ground truth to extract, the budget, and the quality you want.
Next, we’ll explore the most essential element of an ML model development process. So, let’s learn how to design and train a typical ML model.
Designing and training an ML model
Selecting a suitable ML model for the problem a hand is dependent on the problem itself, any constraints, and the ML engineer. Sometimes, the same problem can be solved by different ML algorithms but in other scenarios, it is compulsory to use a specific ML model. Based on the problem and ML model, data should be collected and annotated.
Each ML algorithm will have a different set of hyperparameters, various designs, and a set of decisions to be made throughout the process. It is recommended that you perform pilot or preliminary experiments to identify the best approach for your problem.
When the design process is finalized, the training process can start. For some ML models, the training process could take minutes, while for others, it could take weeks, months, or more! You may need to perform different training experiments to decide which training hyperparameters you are going to continue with – for example, the number of epochs or optimization techniques. Usually, the loss will be a helpful indication of how well the training process is going. In DL, two losses are used: training and validation loss. The first tells us how well the model is learning the training data, while the latter describes the ability of the model to generalize to new data.
Validating and testing an ML model
In ML, we should differentiate between three different datasets/partitions/sets: training, validation, and testing. The training set is used to teach the model about the task and assess how well the model is performing in the training process. The validation set is a proxy of the test set and is used to tell us the expected performance of our model on new data. However, the test set is the proxy of the actual world – that is, where our model will be tested. This dataset should only be deployed so that we know how the model will perform in practice. Using this dataset to change a hyperparameter or design option is considered cheating because it gives a deceptive understanding of how your model will be performing or generalizing in the real world. In the real world, once your model has been deployed, say for example in industry, you will not be able to tune the model’s parameters based on its performance!
Iterations in the ML development process
In practice, developing an ML model will require many iterations between validation and testing and the other stages of the process. It could be that validation or testing results are unsatisfactory and you decide to change some aspects of the data collection, annotation, designing, or training.