In this chapter, we worked on the last project of this entire book, predicting stock (specifically stock index) prices using machine learning regression techniques. We started with a short introduction to the stock market and factors that influence trading prices. To tackle this billion dollar problem, we investigated machine learning regression, which estimates a continuous target variable, as opposed to discrete output in classification. We followed with an in-depth discussion of three popular regression algorithms, linear regression, regression tree and regression forest, and SVR as well as neural networks. We covered the definition, mechanics, and implementation from scratch and with several popular frameworks including scikit-learn, tensorflow, and keras, along with their applications on toy datasets. We also learned the metrics used to evaluate a regression model...




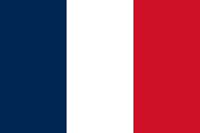


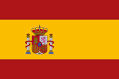




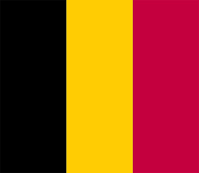
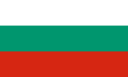
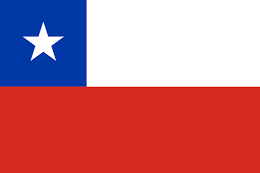
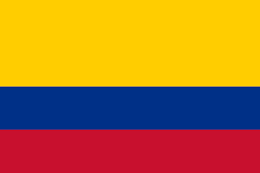
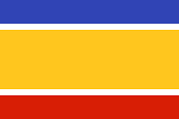

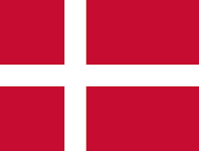



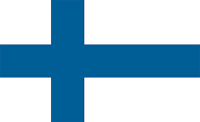

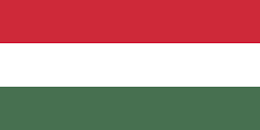






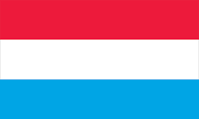
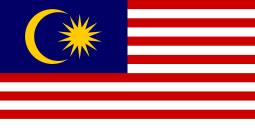

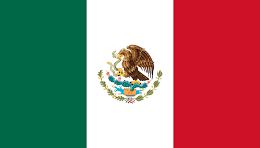

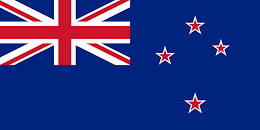







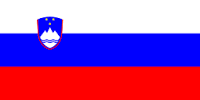




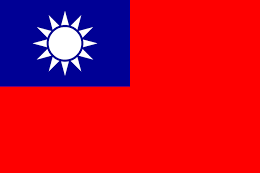


