RDD partitioning
As we have seen in previous chapters, Spark loads data into an RDD. Since Spark runs in distributed mode, different executors can run on different worker machines and RDD is loaded in to the executor(s) memory. RDDs being a distributed dataset gets split across executors. These splits are called RDD partitions.
In other words, partitions are the splits of RDD loaded in different executors memory. The following diagram depicts the logical representation of RDD partitioned across various worker nodes:
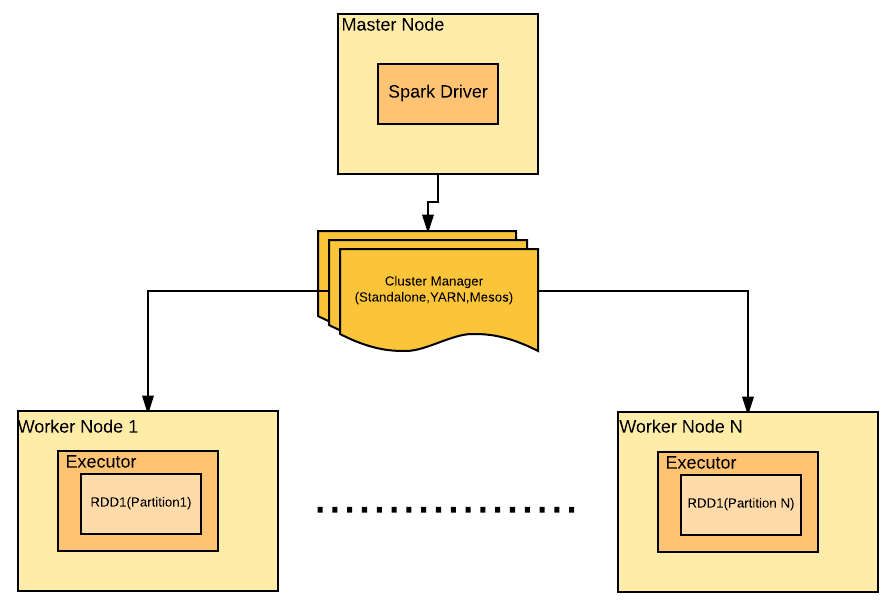
Note
More than one partition of an RDD can be loaded in an executor memory.
Spark partitions the RDD at the time of creation even if the user has not provided any partition count explicitly. However, the user can provide a partition count as well. Let's discuss it programmatically:
SparkConf conf = new SparkConf().setMaster("local").setAppName("Partitioning Example"); JavaSparkContext jsc = new JavaSparkContext(conf); JavaRDD<Integer> intRDD= jsc.parallelize(Arrays.asList(1...