



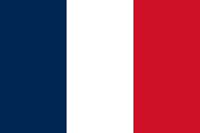


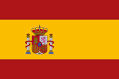



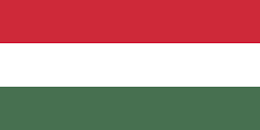

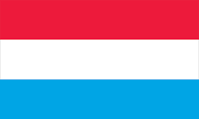





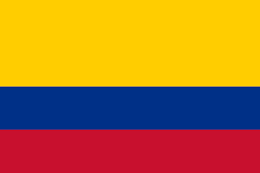
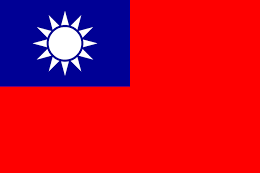
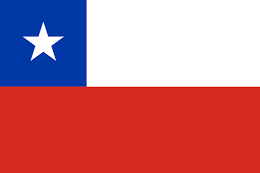



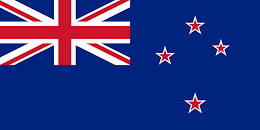
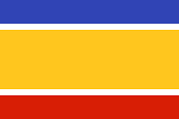
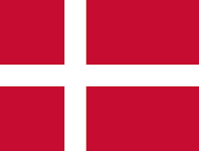
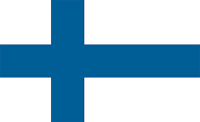







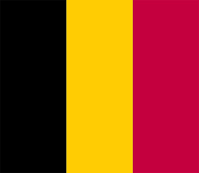

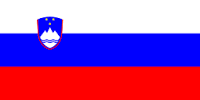





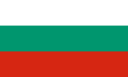


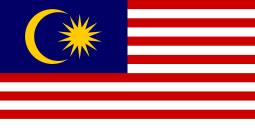



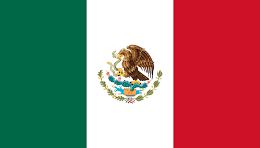

By John P. Desmond, AI Trends Editor
An infrastructure–first approach to AI investing has the potential to yield greater returns with a lower risk profile, suggests a recent account in Forbes. To identify the technologies supporting the AI system, deconstruct the workflow into two steps as a starting point: training and inference.
“Training is the process by which a framework for deep-learning is applied to a dataset,” states Basil Alomary, author of the Forbes account. An MBA candidate at Columbia Business School and MBA Associate at Primary Venture Partners, his background and experience are in early-stage SaaS ventures, as an operator and an investor. “That data needs to be relevant, large enough, and well-labeled to ensure that the system is being trained appropriately. Also, the machine learning models being created need to be validated, to avoid overfitting to the training data and to maintain a level of generalizability. The inference portion is the application of this model and the ongoing monitoring to identify its efficacy.”
He identifies these stages in the AI/ML development lifecycle: data acquisition, data preparation, training, inference, and implementation. The stages of acquisition, preparation, and implementation have arguably attracted the least amount of attention from investors.
Where to get the data for training the models is a chief concern. If a company is old enough to have historical customer data, it can be helpful. That approach should be inexpensive, but the data needs to be clean and complete enough to help in whatever decisions it works on. Companies without the option of historical data, can try publicly-available datasets, or they can buy the data directly. A new class of suppliers is emerging that primarily focus on selling clean, well-labeled datasets specifically for machine learning applications.
One such startup is Narrative, based in New York City. The company sells data tailored to the client’s use case. The OpenML and Amazon Datasets have marketplace characteristics but are entirely open source, which is limiting for those who seek to monetize their own assets.
“Essentially, the idea was to take the best parts of the e-commerce and search models and apply that to a non-consumer offering to find, discover and ultimately buy data,” stated Narrative founder and CEO Nick Jordan in an account in TechCrunch. “The premise is to make it as easy to buy data as it is to buy stuff online.”
In a demonstration, Jordan showed how a marketer could browse and search for data using the Narrative tools. The marketer could select the mobile IDs of people who have the Uber Driver app installed on their phone, or the Zoom app, at a price that is often subscription-based. The data selection is added to the shopping cart and checked out, like any online transaction.
Founded in 2016, Narrative collects data sellers into its market, vetting each one, working to understand how the data is collected, its quality, and whether it could be useful in a regulated environment. Narrative does not attempt to grade the quality of the data. “Data quality is in the eye of the beholder,” Jordan stated. Buyers are able to conduct their own research into the data quality if so desired. Narrative is working on building a marketplace of third-party applications, which could include scoring of data sets.
Data preparation is critical to making the machine learning model effective. Raw data needs to be preprocessed so that machine learning algorithms can produce a model, a structural description of the data. In an image database, for example, the images may have to be labelled, which can be labor-intensive.
Automating Data Preparation is an Opportunity Area
Platforms are emerging to support the process of data preparation with a layer of automation that seeks to accelerate the process. Startup Labelbox recently raised a $25 million Series B financing round to help grow its data labeling platform for AI model training, according to a recent account in VentureBeat.
Founded in 2018 in San Francisco, Labelbox aims to be the data platform that acts as a central hub for data science teams to coordinate with dispersed labeling teams. In April, the company won a contract with the Department of Defense for the US Air Force AFWERX program, which is building out technology partnerships.
A press release issued by Labelbox on the contract award contained some history of the company. “I grew up in a poor family, with limited opportunities and little infrastructure” stated Manu Sharma, CEO and one of Labelbox’s co-founders, who was raised in a village in India near the Himalayas. He said that opportunities afforded by the U.S. have helped him achieve more success in ten years than multiple generations of his family back home. “We’ve made a principled decision to work with the government and support the American system,” he stated.
The Labelbox platform is supporting supervised-learning, a branch of AI that uses labeled data to train algorithms to recognize patterns in images, audio, video or text. The platform enables collaboration among team members as well as these functions: rework, rework, quality assurance, model evaluation, audit trails, and model-assisted labeling.
“Labelbox is an integrated solution for data science teams to not only create the training data but also to manage it in one place,” stated Sharma. “It’s the foundational infrastructure for customers to build their machine learning pipeline.”
Deploying the AI model into the real world requires an ongoing evaluation, a data pipeline that can handle continued training, scaling and managing computing resources, suggests Alomary in Forbes. An example product is Amazon’s Sagemaker, supporting deployment. Amazon offers a managed service that includes human interventions to monitor deployed models.
DataRobot of Boston in 2012 saw the opportunity to develop a platform for building, deploying, and managing machine learning models. The company raised a Series E round of $206 million in September and now has $431 million in venture-backed funding to date, according to Crunchbase.
Unfortunately DataRobot in March had to shrink its workforce by an undisclosed number of people, according to an account in BOSTINNO. The company employed 250 full-time employees as of October 2019.
DataRobot announced recently that it was partnering with Amazon Web Services to provide its enterprise AI platform free of charge to anyone using it to help with the coronavirus response effort.
Read the source articles and releases in Forbes, TechCrunch, VentureBeat and BOSTINNO.