Graphs, AI agents, and LangGraph
LangChain introduced LangGraph in 2024, so it is still relatively new. It is an extension built on top of LangChain Expression Language (LCEL) to create composable and customizable agentic workloads. LangGraph leans heavily on graph theory concepts, such as nodes and edges (described earlier), but with a focus on using them to manage your AI agents. While an older way to manage agents, the AgentExecutor
class, still exists, LangGraph is now the recommended way to build agents in LangChain.
LangGraph adds two important components for supporting agents:
- The ability to easily define cycles (cyclical graphs)
- Built-in memory
It provides a pre-built object equivalent to AgentExecutor
, allowing developers to orchestrate agents using a graph-based approach.
Over the past couple of years, numerous papers, concepts, and approaches have emerged for building agents into RAG applications, such as orchestration agents, ReAct agents, self-refine agents, and multi-agent frameworks. A common theme among these approaches is the concept of a cyclical graph that represents the agent’s control flow. While many of these approaches, from an implementation standpoint, are going obsolete, their concepts are still highly useful and are captured in the graph-based environment of LangGraph.
LangGraph has become a powerful tool for supporting agents and managing their flow and process in RAG applications. It enables developers to describe and represent single and multi-agent flows as graphs, providing extremely controlled flows. This controllability is crucial for avoiding the pitfalls encountered by developers when creating agents early on.
As an example, the popular ReAct approach was an early paradigm for building agents. ReAct stands for reason + act. In this pattern, an LLM first thinks about what to do and then decides an action to take. That action is then executed in an environment and an observation is returned. With that observation, the LLM then repeats this process. It uses reasoning to think about what to do next, decides another action to take, and continues until it has been determined that the goal has been met. If you map this process out, it may look something like what you see here in Figure 12.2:
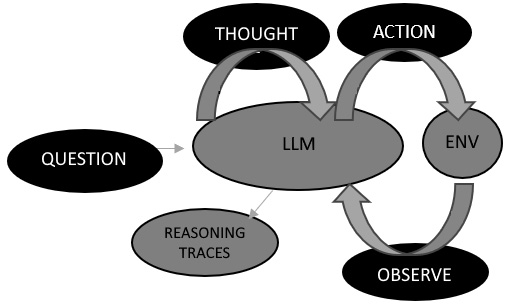
Figure 12.2 – ReAct cyclical graph representation
The set of loops in Figure 12.2 can be represented by cyclical graphs in LangGraph, with each step represented by nodes and edges. Using this graphing paradigm, you can see how a tool such as LangGraph, a tool for building graphs in LangChain, can form the backbone of your agent framework. As we build our agent framework, we can represent these agent loops using LangGraph, which helps you describe and orchestrate the control flow. This focus on the control flow is critical to addressing some of the early challenges with agents, where a lack of control leads to rogue agents that can’t complete their loops or focus on the wrong task.
Another key element that LangGraph has built into it is persistence. Persistence can be used to maintain the memory of the agent, giving it the information it needs to reflect on all of its actions so far, and representing the OBSERVE component presented in Figure 12.2. This is really helpful for having multiple conversations at the same time or remembering previous iterations and actions. This persistence also enables human-in-the-loop features that give you better control over what the agent is doing at key intervals during its actions.
The paper that introduced the ReAct approach to agent building can be found here: https://arxiv.org/abs/2210.03629
Let’s dive right into the code lab for building our agent and walk through more key individual concepts as we encounter them in the code.