Evolution of big data analytics
The previous section outlined how the computational and data analytics challenges were addressed for big data requirements. It was possible because of the convergence of several related trends such as low-cost commodity hardware, accessibility to big data, and improved data analytics techniques. Hadoop became a cornerstone in many large, distributed data processing infrastructures.
However, people soon started realizing the limitations of Hadoop. Hadoop solutions were best suited for only specific types of big data requirements such as ETL; it gained popularity for such requirements only.
There were scenarios when data engineers or analysts had to perform ad hoc queries on the data sets for interactive data analysis. Every time they ran a query on Hadoop, the data was read from the disk (HDFS-read) and loaded into the memory - which was a costly affair. Effectively, jobs were running at the speed of I/O transfers over the network and cluster of disks, instead of the speed of CPU and RAM.
The following is a pictorial representation of the scenario:
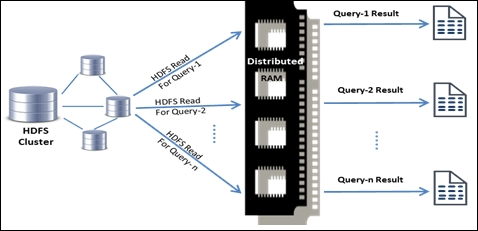
One more case where Hadoop's MapReduce model could not fit in well was with machine learning algorithms that were iterative in nature. Hadoop MapReduce was underperforming, with huge latency in iterative computation. Since MapReduce had a restricted programming model with forbidden communication between Map and Reduce workers, the intermediate results needed to be stored in a stable storage. So, those were pushed on to the HDFS, which in turn writes into the instead of saving in RAM and then loading back in the memory for the subsequent iteration, similarly for the rest of the iterations. The number of disk I/O was dependent on the number of iterations involved in an algorithm and this was topped with the serialization and deserialization overhead while saving and loading the data. Overall, it was computationally expensive and could not get the level of popularity compared to what was expected of it.
The following is a pictorial representation of this scenario:
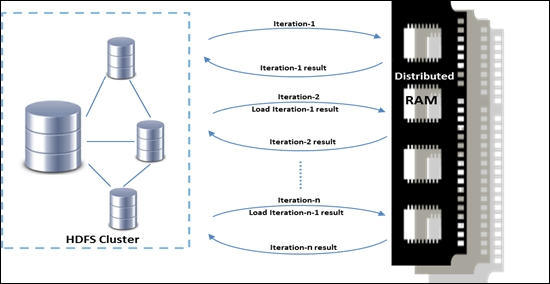
To address this, tailor-made solutions were developed, for example, Google's Pregel, which was an iterative graph processing algorithm and was optimized for inter-process communication and in-memory storage for the intermediate results to make it run faster. Similarly, many other solutions were developed or redesigned that would best suit some of the specific needs that the algorithms used were designed for.
Instead of redesigning all the algorithms, a general-purpose engine was needed that could be leveraged by most of the algorithms for in-memory computation on a distributed computing platform. It was also expected that such a design would result in faster execution of iterative computation and ad hoc data analysis. This is how the Spark project paved its way out at the AMPLab at UC Berkeley.