Keeping track of how variables change during the training of a model can be a tedious job. For instance, in the linear regression example, we kept track of the MSE loss and of the parameters of the model by printing them every 40 epochs. As the complexity of the algorithms increases, there is an increase in the number of variables and metrics to be monitored. Fortunately, this is where TensorBoard comes to the rescue.
TensorBoard is a suite of visualization tools that can be used to plot metrics, visualize TensorFlow graphs, and visualize additional information. A typical TensorBoard screen is similar to the one shown in the following screenshot:
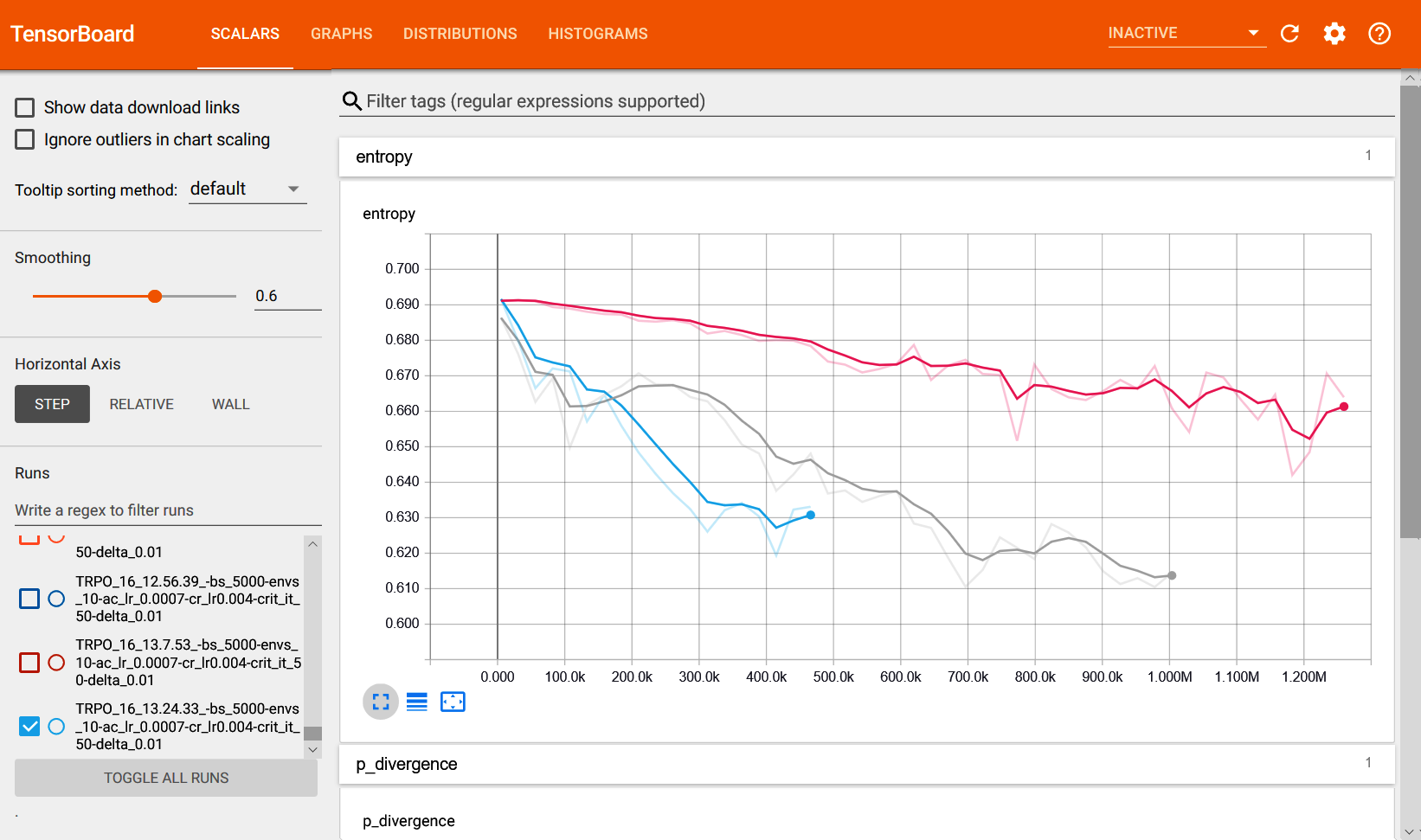
The integration of TensorBoard with TensorFlow code is pretty straightforward as it involves only a few tweaks to the code. In particular, to visualize the MSE loss over time...