Looking at different performance evaluation metrics
In the previous sections and chapters, we evaluated different machine learning models using the prediction accuracy, which is a useful metric with which to quantify the performance of a model in general. However, there are several other performance metrics that can be used to measure a model's relevance, such as precision, recall, and the F1 score.
Reading a confusion matrix
Before we get into the details of different scoring metrics, let's take a look at a confusion matrix, a matrix that lays out the performance of a learning algorithm.
A confusion matrix is simply a square matrix that reports the counts of the true positive (TP), true negative (TN), false positive (FP), and false negative (FN) predictions of a classifier, as shown in the following figure:
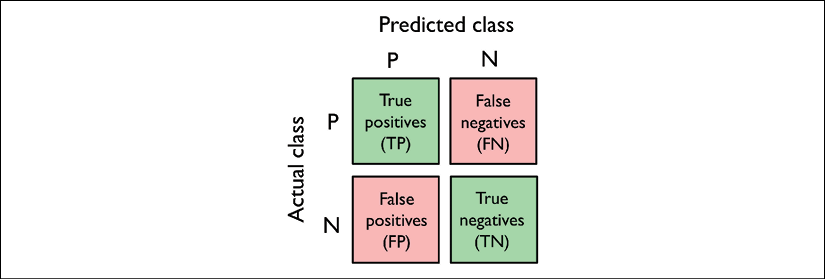
Although these metrics can be easily computed manually by comparing the true and predicted class labels, scikit-learn provides a convenient confusion_matrix...