Chapter 2: Building and Using Your Own Algorithm Container Image
In the previous chapter, we performed a simplified end-to-end machine learning experiment with the Amazon SageMaker built-in algorithm called Linear Learner. At the time of writing, there are 17 built-in algorithms to choose from! Depending on our requirements, we may simply choose one or more algorithms from these 17 built-in algorithms to solve our machine learning problem. In real life, we will be dealing with pre-trained models and other algorithms that are not in this list of built-in algorithms from SageMaker. One of the strengths of Amazon SageMaker is its flexibility and support for custom models and algorithms by using custom container images. Let's say that you want to use an algorithm that's not available in the list of built-in algorithms from SageMaker, such as Support Vector Machines (SVM), to solve your machine learning problems. If that's the case, then this chapter is for you!
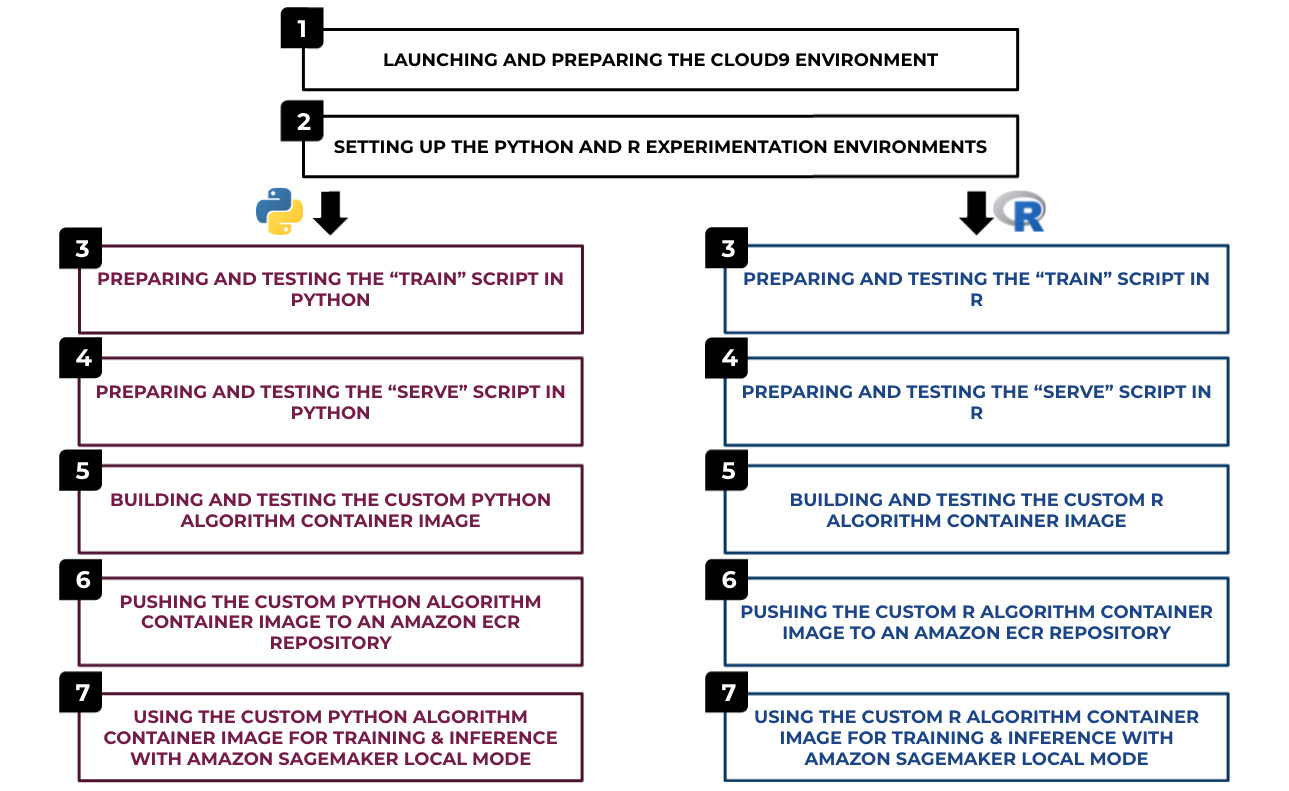
Figure 2.1 – Chapter 2 recipes
In this chapter, we will work on creating and using our own algorithm container images in Amazon SageMaker. With this approach, we can use any custom scripts, libraries, frameworks, or algorithms. This chapter will enlighten us on how we can make the most out of Amazon SageMaker through custom container images. As shown in the preceding diagram, we will start by setting up a cloud-based integrated development environment with AWS Cloud9, where we will prepare, configure, and test the scripts before building the container image. Once we have the environment ready, we will code the train and serve scripts inside this environment. The train script will be used during training, while the serve script will be used for the inference endpoint of the deployed model. We will then prepare a Dockerfile
that makes use of the train and serve scripts that we generated in the earlier steps. Once this Dockerfile
is ready, we will build the custom container image and use the container image for training and inference with the SageMaker Python SDK. We will work on these steps in both Python and R.
We will cover the following recipes in this chapter:
- Launching and preparing the Cloud9 environment
- Setting up the Python and R experimentation environments
- Preparing and testing the train script in Python
- Preparing and testing the serve script in Python
- Building and testing the custom Python algorithm container image
- Pushing the custom Python algorithm container image to an Amazon ECR repository
- Using the custom Python algorithm container image for training and inference with Amazon SageMaker Local Mode
- Preparing and testing the train script in R
- Preparing and testing the serve script in R
- Building and testing the custom R algorithm container image
- Pushing the custom R algorithm container image to an Amazon ECR repository
- Using the custom R algorithm container image for training and inference with Amazon SageMaker Local Mode
After we have completed the recipes in this chapter, we will be ready to use our own algorithms and custom container images in SageMaker. This will significantly expand what we can do outside of the built-in algorithms and container images provided by SageMaker. At the same time, the techniques and concepts used in this chapter will give you the exposure and experience needed to handle similar requirements, as you will see in the upcoming chapters.