Until now, we have trained models on single instances, iterating an algorithm in order to minimize a target loss function. This approach is based on so-called strong learners, or methods that are optimized to solve a specific problem by looking for the best possible solution (highest accuracy). Another approach is based on a set of weak learners, which, formally, are estimators that are able to achieve an accuracy slightly higher than 0.5. In the real world, the actual estimators used in Ensemble Learning are much more accurate than their theoretical counterparts, but generally they are able to specialize a single region of the sample space and show bad performance while considering the whole dataset. Moreover, they can be trained in parallel or sequentially (with slight modifications to the parameters) and used as an ensemble (group) based on...




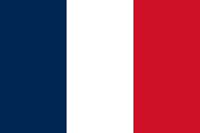


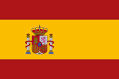



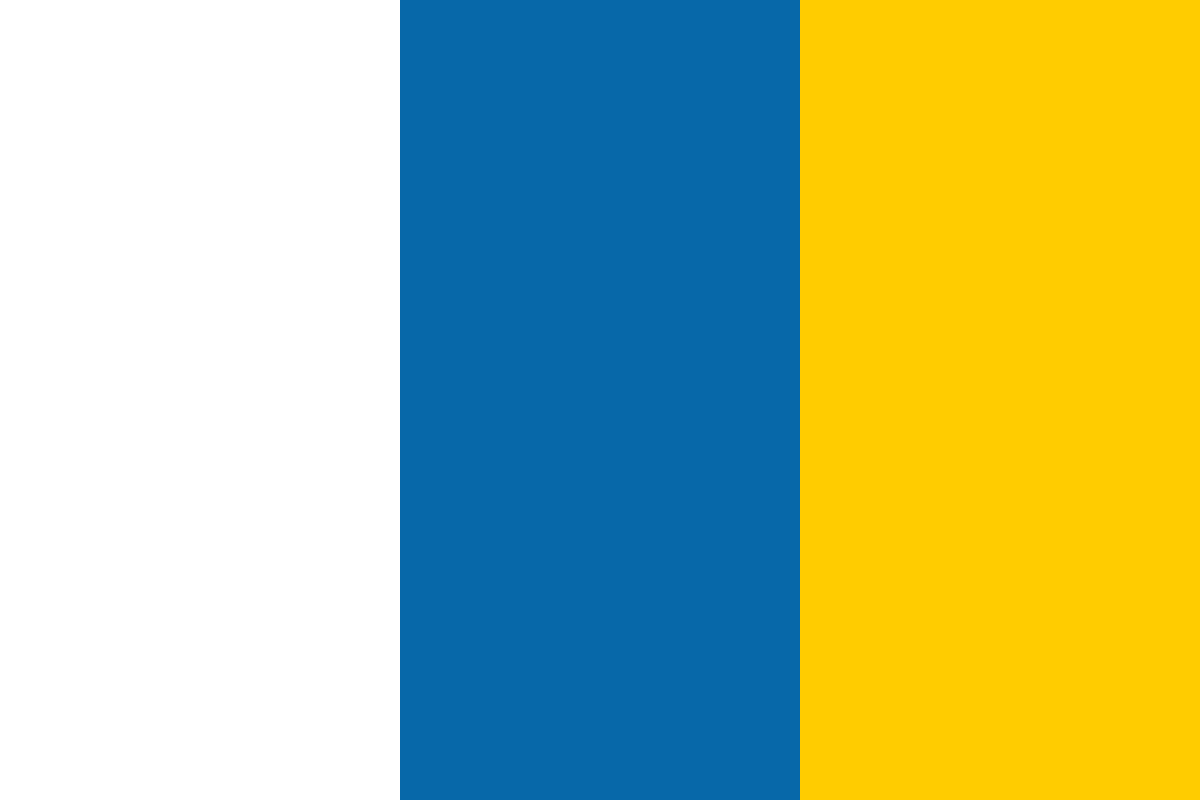
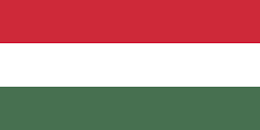

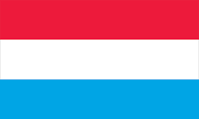





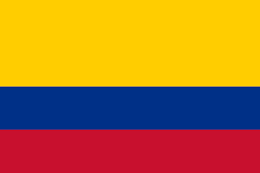
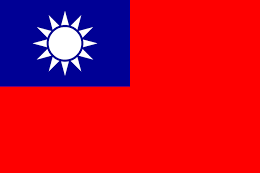
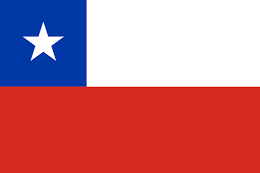



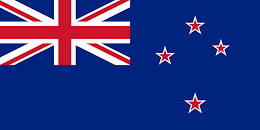
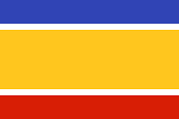
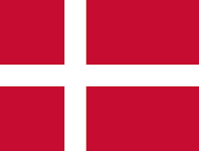
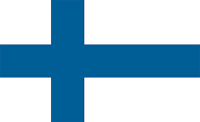







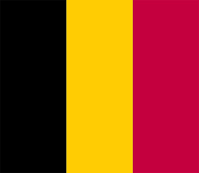

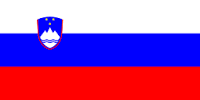





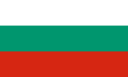


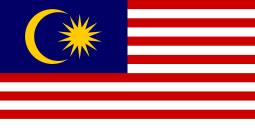



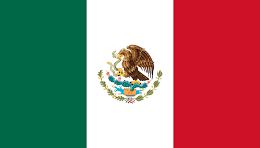
