Understanding MLOps
Software development is multidisciplinary, and it's changing to facilitate ML. MLOps is a new approach for fusing ML and software development by combining different domains. MLOps combines ML, DevOps, and data engineering, with the goal of reliably and efficiently building, deploying, and maintaining ML systems in production. Thus, MLOps can be explained by this intersection.
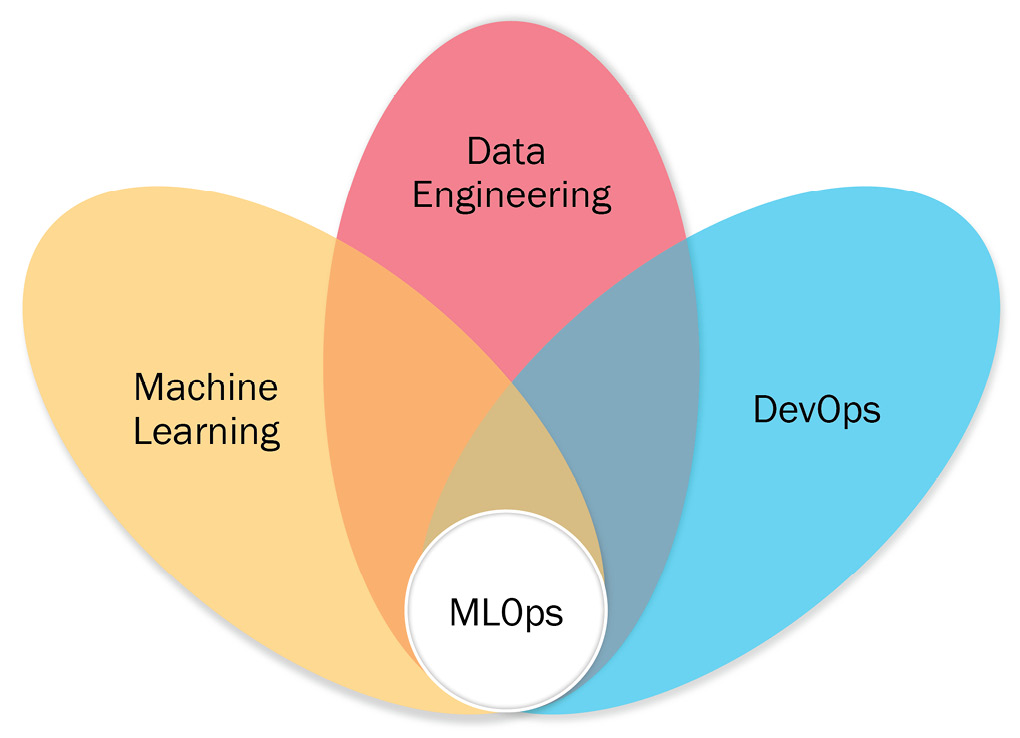
Figure 1.7 – MLOps intersection
To make this intersection (MLOps) operational, I have designed a modular framework by following the systematic design science method proposed by Wieringa (https://doi.org/10.1007/978-3-662-43839-8) to develop a workflow to bring these three together (Data Engineering, Machine Learning, and DevOps). Design science goes with the application of design to problems and context. Design science is the design and investigation of artifacts in a context. The artifact in this case is the MLOps workflow, which is designed iteratively by interacting with problem contexts (industry use cases for the application of AI):
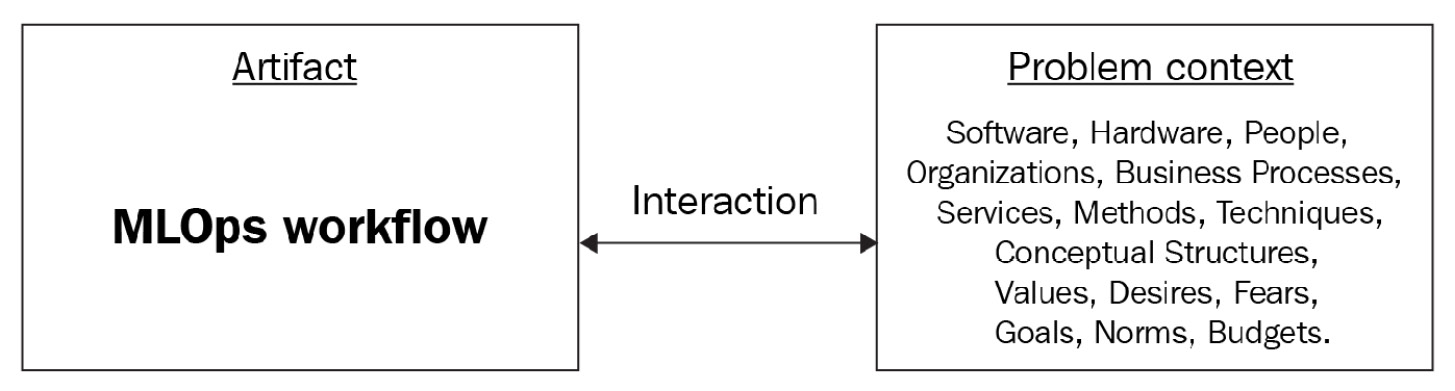
Figure 1.8 – Design science workflow
In a structured and iterative approach, the implementation of two cycles (the design cycle and the empirical cycle) was done for qualitative and quantitative analysis for MLOps workflow design through iterations. As a result of these cycles, an MLOps workflow is developed and validated by applying it to multiple problem contexts, that is, tens of ML use cases (for example, anomaly detection, real-time trading, predictive maintenance, recommender systems, virtual assistants, and so on) across multiple industries (for example, finance, manufacturing, healthcare, retail, the automotive industry, energy, and so on). I have applied and validated this MLOps workflow successfully in various projects across multiple industries to operationalize ML. In the next section, we will go through the concepts of the MLOps workflow designed as a result of the design science process.