One of the major difficulties with image classification models is the lack of labeled data. It is difficult to assemble a labeled dataset of sufficient size to train a model well; it is an extremely time consuming and laborious task. This is not such a problem for MNIST, since the images are relatively simple. They are greyscale and largely consist only of target features, there are no distracting background features, and the images are all aligned the same way and are of the same scale. A small dataset of 60,000 images is quite sufficient to train a model well. It is rare to find such a well-organized and consistent dataset in the problems we encounter in real life. Images are often of variable quality, and the target features can be obscured or distorted. They can also be of widely variable scales and rotations. The solution is to use a model architecture that...




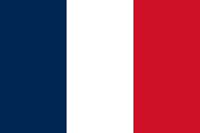


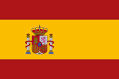



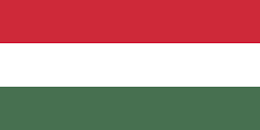

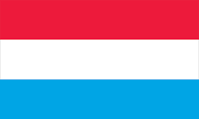





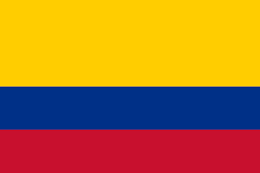
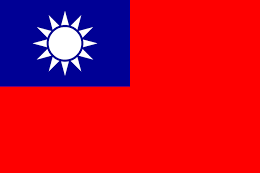
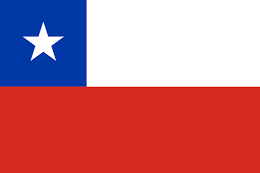



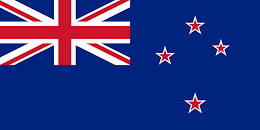
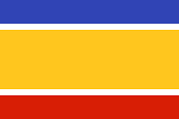
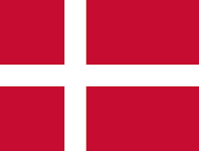
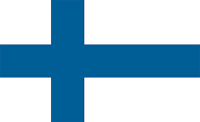







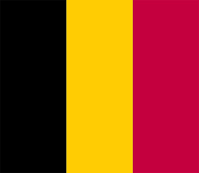

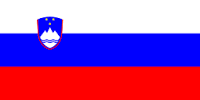





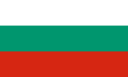


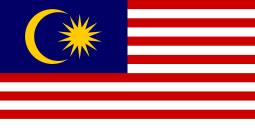



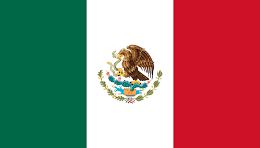
