



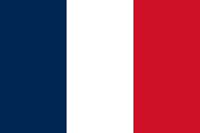


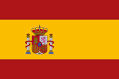



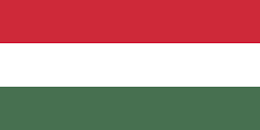

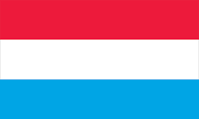





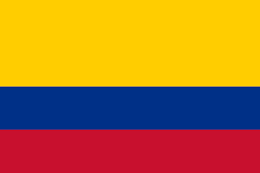
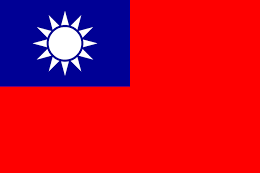
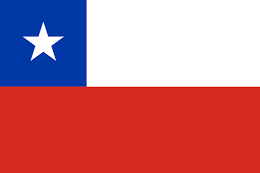



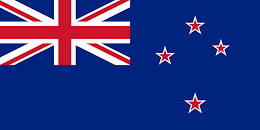
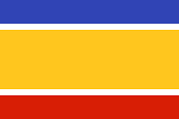
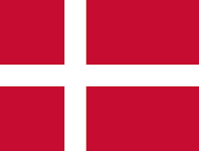
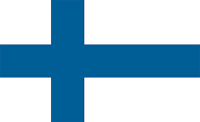







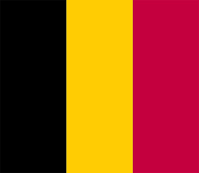

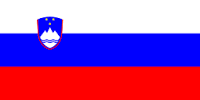





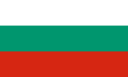


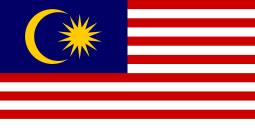



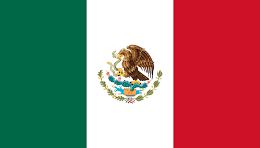

This line stands true for Deepmind’s latest AI program, AlphaZero.
AlphaZero is an advanced version of AlphaGo Zero--the AI that recently won all games of Go against its precursor AlphaGo--relies simply on self-play without any example games. AlphaZero is an improvement to it as it shows that the same program can master three different types of board games, Chess, Shogi and Go namely. It uses reinforcement learning algorithm to achieve state-of-the-art results.
AlphaZero mastered the game of chess, without having prior domain knowledge of the game, except the game rules. Additionally, it also mastered Shogi, a Japanese board game, as showcased in a recent DeepMind research paper.
Demis Hassabis, founder, and CEO, DeepMind introduced some additional details of AlphaZero at the Neural Information Processing Systems (NIPS) conference in Long Beach, California. “It doesn’t play like a human, and it doesn’t play like a program, it plays in a third, almost alien, way,” said Hassabis. It only took four hours to self-play and create chess knowledge beyond any human or computer program. Surprisingly, it defeated Stockfish 8 (A world champion chess engine) in four hours without any external help or any prior empirical data (a database of archived chess games, or well-known chess strategies and openings).
The hyper-parameter of AlphaGo Zero’s search was tuned by using Bayesian optimization algorithm. AlphaZero reuses the same hyper-parameter for playing all the board games without performing any game-specific tuning. Similar to AlphaGo Zero, AlphaZero’s board state is encoded by spatial planes based on specifically the basic rules for each game.
While training AlphaZero, the same algorithmic settings, network architecture, and hyper-parameters were used in all three games. A separate instance of AlphaZero was trained for each game. The training initiated for 700,000 steps (mini-batches of size 4,096) starting from randomly initialized parameters, with 5,000 first-generation TPUs to generate self-play games and 64 second-generation TPUs to train the neural networks.
After comprehensive analysis, it was found that AlphaZero outperformed
The achievements by AlphaZero are impressive, to say the least. Researchers at DeepMind say that it still needs to play many more practice games than a human chess champion. Human learning is based on watching other people play and also by learning in different ways, which a machine cannot achieve. But it can go beyond human thinking by expanding the capabilities of its program. To know more about how AlphaZero masters chess and Shogi using Reinforcement algorithm, you can have a look at the research paper here or tune into the game series on Youtube to watch the video.