Understanding the importance of model serving
Model serving is one of the critical steps in the ML life cycle but is often neglected. As shown in Figure 1.7, users can only start using the model after serving is done. So, model serving is the key step to the business success of a data science or ML team.
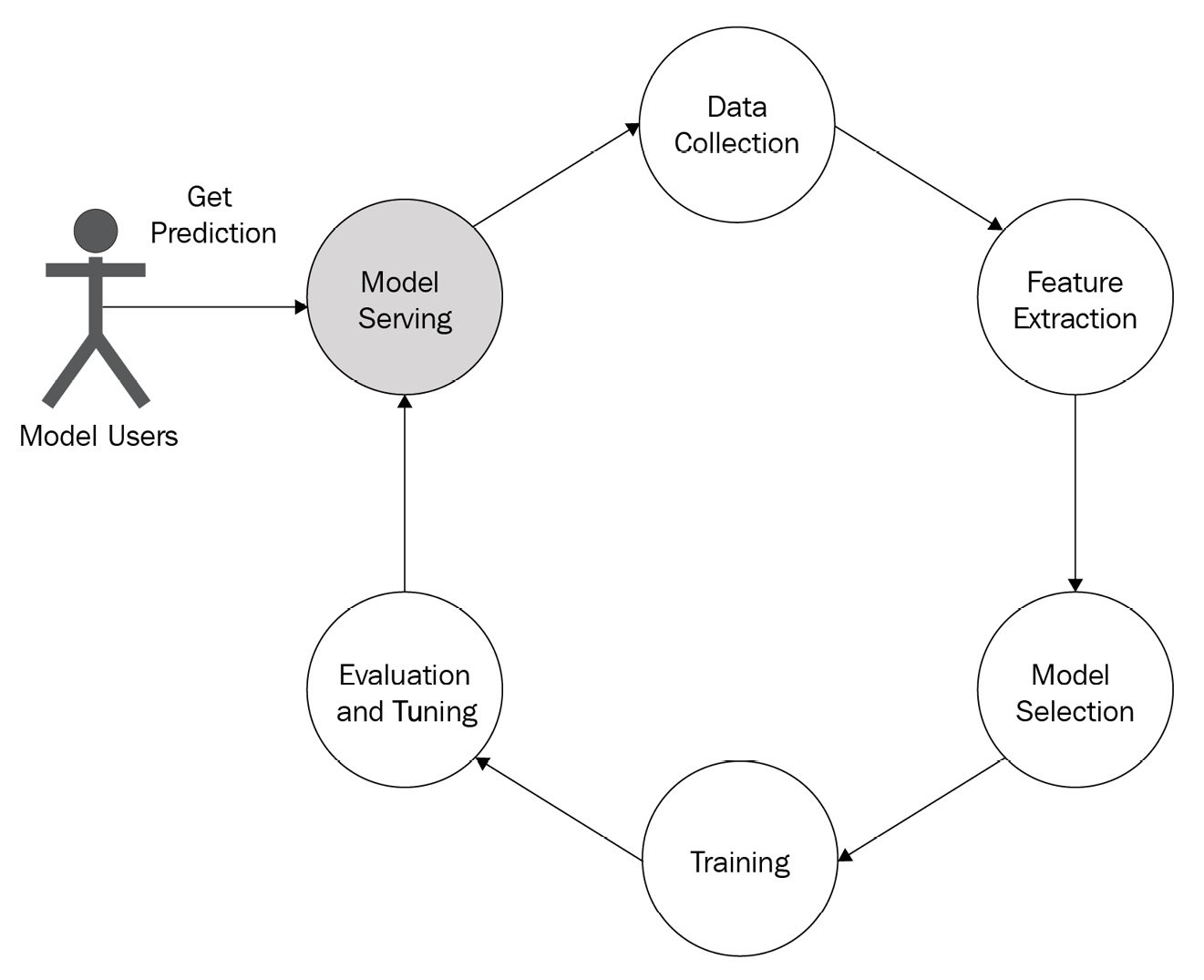
Figure 1.7 – ML life cycle
A lot of models remain unserved simply because model serving is hard. This happens mainly due to the following reasons:
- Separation of responsibilities: Often, model development is assigned to data scientists and serving is assigned to engineers, and there may be a gap between the domain knowledge of the two groups. For example, consider a data scientist who has developed a model using a notebook and is getting some predictions and a software engineer who will be serving the model. The product manager is asking for the model to be provided on a server in a production-ready state but some challenges come up:
- How should they save the model?
- Are the tools the software engineer is using compatible with the model the data scientist has developed?
- Data scientists tend to use a lot of fancy libraries to make models; are all those libraries supported in the platform the software engineering team is using?
- How will they maintain the version of the model? Is the model developed by data scientists easily maintainable?
- What kind of serving mechanism is needed?
- Which endpoint needs to be published for prediction?
- How does the data from users need to be processed on the server?
Challenges like this create barriers to serving a model after development.
- Choice of tool: Tool after tool is appearing for serving models. It makes serving more challenging, as a particular tool may be the perfect choice for a particular type of model, and you need to know which tool is best for which model type. For example, for a simple model, simple REST APIs developed using Flask may be sufficient. However, for complex models, developing a Flask API can be less effective as it was not developed to serve the purpose of stateful client-server communication. Switching from one tool to another can be a problem, and identifying which tool is better for which problem might be challenging. Therefore, as the volume of tools increases, the volume of confusion and challenges in model serving keep increasing.
- Versioning: In software engineering, the versioning of software is very easy. We need to redeploy the new updates through a continuous integration/continuous deployment (CI/CD) pipeline for a software application. However, in model serving, versioning is challenging because it involves new feature engineering, retraining with new data, and evaluation. Ensuring backward compatibility is not an easy task as it can lead to an error state in ML. Versioning models can be frustrating.
- Rollback: In software engineering, let’s say deployment to production is behaving incorrectly after the last change; we can then easily roll back the last change and go back to the previous state very quickly. However, in model serving, we cannot take advantage of this shortcut to roll back to the last model.
The preceding points gave us an idea about the challenges involved in model serving. In the following section, we will introduce you to some existing tools for model serving.