A data architecture reference diagram
The reference architecture diagram that is abstractly defined for now in Figure 1.3 shows the typical structure of an end-to-end data platform in a (hybrid) cloud:
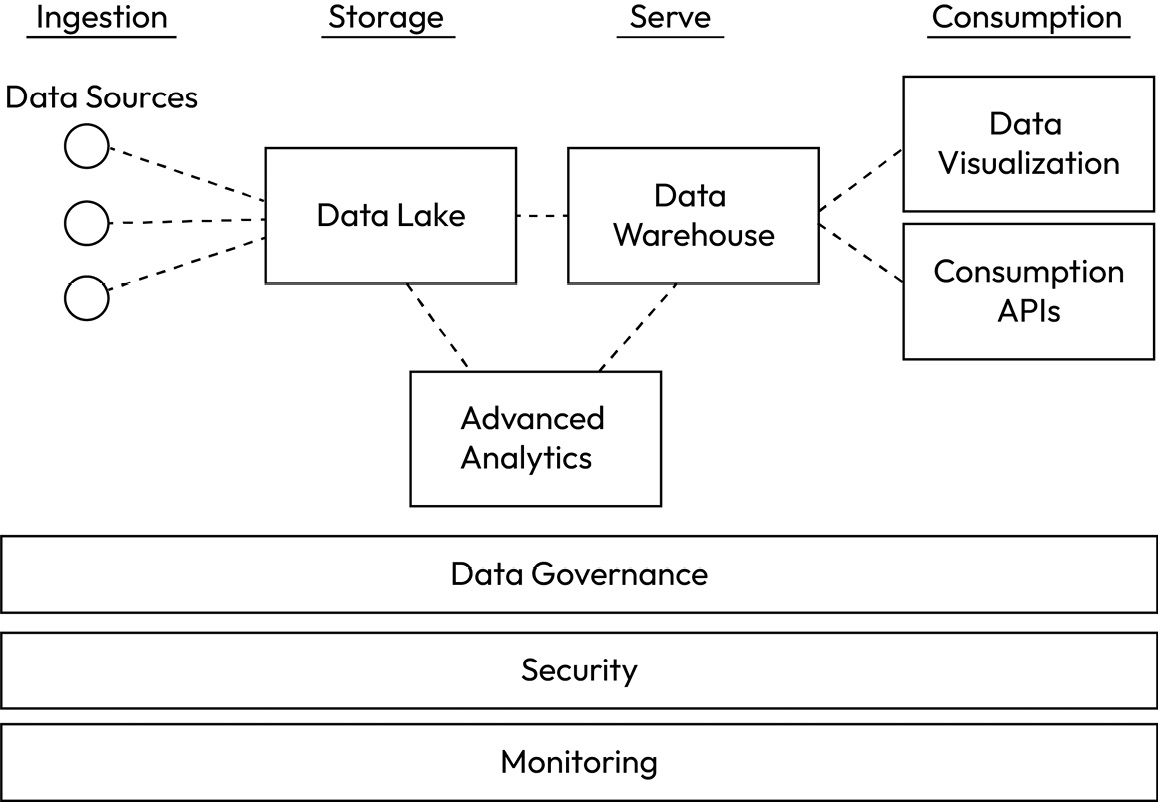
Figure 1.3 – A typical structure of an end-to-end data platform in a (hybrid) cloud
This reference diagram shows the key components of most modern cloud data platforms. There are limitless possible adaptations, such as accommodating streaming data, but the diagram in Figure 1.3 serves as the basis for more advanced data architectures. It’s like the Pizza Margherita of data architectures! The architecture diagram in Figure 1.3 already shows four distinct layers in the end-to-end architecture, as follows:
- The ingestion layer
- The storage layer
- The serving layer
- The consumption layer
Next to these layers, there are a couple of other key aspects of the data platform that span across multiple layers, as follows:
- Data orchestration and processing
- Advanced analytics
- Data governance and compliance
- Security
- Monitoring
Let’s cover the first layer next.
The ingestion layer
The ingestion layer serves as the data entrance to the cloud environment. Here, data from various sources is pulled into the cloud. These sources include on-premises databases, SaaS applications, other cloud environments, Internet of Things (IoT) devices, and many more. Let’s look at this layer in more detail:
- First, the number of data sources can vary greatly between businesses and could already bring a variety of challenges to overcome. In enterprise-scale organizations, when the amount of data sources can reach extraordinary levels, it is of exceptional importance to maintain a clear overview and management of these sources.
- Secondly, the sheer variety of sources is another common issue to deal with. Different data sources can have distinct methods of ingesting data into the cloud and, in some cases, require architectural changes to accommodate.
- Thirdly, managing authentication for data sources can be cumbersome. Authentication, which happens in a multitude of ways, is often unique to the data source. Every source requires its own tokens, keys, or other types of credentials that must be managed and seamlessly refreshed to optimize security.
From a design perspective, there are a few other aspects to keep in mind. The architect should consider the following:
- Data speed: Will incoming data from the source be ingested periodically (that is, batch ingestion) or continuously (that is, data streaming)?
- Level of the structure of the data: Will the incoming data be unstructured, semi-structured, or structured?
Regarding data speed, data will be ingested in batches in the vast majority of cases. This translates to periodical requests made to an application programming interface (API) to pull data from the data source. For the more uncommon cases of streaming data, architectural changes are required to provide an environment to store and process the continuous flow of data. In later chapters, you will discover how the platform architecture will differ to accommodate the streaming data.
Finally, the level of structure of the data will determine the amount of required data transformations, the methods of storing the data, or the destination of data movements. Unstructured data, such as images and audio files, will require different processing compared to semi-structured key-value pairs or structured tabular files.
(Add what data ingestion services will be discussed later in the book).
The storage layer
The definitions of the following layers can vary. Over the course of this book, the storage layer refers to the central (often large-scale) storage of data. Data lakes are the most common method for massive storage of data, due to their capacity and relatively low cost. Alternatives are graph-based databases, relational databases, NoSQL databases, flat file-based databases, and so on. The data warehouse, which holds business-ready data and is optimized for querying and analytics, does not belong to the storage layer but will fall under the serving layer instead.
Decisions made by the architect in the storage layer can have a great effect on costs, performance, and the data platform in its entirety. Here, the architect will have to consider redundancy, access tiers, and security. In the case of a data lake, a tier system needs to be considered for raw, curated, and enriched data, as well as a robust and scalable folder structure.
(Add what data storage services will be discussed later in the book).
The serving layer
In the serving layer, preprocessed and cleansed data is stored in a data warehouse, often regarded as the flagship of the data platform. This is a type of structured storage that is optimized for large-scale queries and analytics. The data warehouse forms one of the core components of BI.
The major difference between a data warehouse and the aforementioned data lake is the level of structure. A data warehouse is defined by schemas and enforces data types and structures. Conversely, a data lake can be seen as a massive dump of all kinds of data, with little to no regard for the enforcement of specific rules. The strong level of enforcement makes a data warehouse significantly more homogeneous, which results in far better performance for analytics.
The cloud data architect has various decisions to make in the serving layer. There are quite a few options for data warehousing on the Azure cloud, as follows:
- First, the architect should think about whether they want an Infrastructure-as-a-Service (IaaS), a Platform-as-a-Service (PaaS), or a SaaS solution. In short, this results in a trade-off between management responsibilities, development efforts, and flexibility. This will be discussed more in later chapters.
- Next, different services on Azure come with their own advantages and disadvantages. The architect could, for example, opt for a very cost-effective serverless SQL solution or leverage massive processing power in highly performant dedicated SQL pools, among numerous other options.
After deciding on the most fitting service, there are still decisions to be made within the data warehouse. The architect will have to determine structures to organize the data in the data warehouse, also known as schemas. Common schemas are star and snowflake schemas, which also come with their own benefits and drawbacks.
Chapter 6, Data Warehousing, will teach you all the necessary skills to confidently decide on the right solution. Chapter 7, The Semantic Layer, will introduce you to the concept of data marts, subsets of a data warehouse ready for business consumption.
The consumption layer
The consumption layer is the final layer of an end-to-end data architecture and typically follows the serving layer by extracting data from the data warehouse. There are numerous ways of consuming the data, which has been prepared and centralized in earlier stages.
The most common manner of consumption is through data visualization. This can happen through dashboarding and building reports. The combination of a data warehouse and a visualization service is often referred to as BI. Many modern dashboarding tools allow for interactivity and drill-down functionality within the dashboard itself. Although technically it is not a part of the Azure stack, Power BI is the preferred service for data visualization for Azure data platforms. However, Microsoft allows other visualization services to connect conveniently as well.
Another way to consume data is by making the data available to other applications or platforms using APIs.
Chapter 8, Visualizing Data Using Power BI, will teach you how to extract data from the data warehouse in various ways and visualize it using interactive dashboarding. In this chapter, you will also discover methods to perform self-service BI, allowing end users to create their own ad hoc dashboards and reports to quickly perform data analysis.
Data orchestration and processing
Contrary to the four layers mentioned previously, there are a couple of other core components of the data platform that span across the entire end-to-end process.
Data orchestration refers to moving data from one place to another, often using data pipelines. This process is often done by data engineers. When data is moved from one stage to the next, data undergoes transformations in the form of joining data, deriving new columns, computing aggregations, and so on. For example, when data is moved from a data lake to a data warehouse, it must be transformed to match the data model, which is enforced by the data warehouse. Another example is when moving data between tiers (raw, curated, and enriched tiers) in the data lake, where the data becomes more and more ready for business use whenever it moves up a tier.
Data pipelines allow data engineers to automate and scale the orchestration and processing of data. These components are critical to the performance and health of the data platform and must be monitored accordingly.
Here are two common methods of performing orchestration and processing:
- Extract-Transform-Load (ETL)
- Extract-Load-Transform (ELT)
In both cases, data is extracted from a source and loaded to a destination. The main difference between both methods is the location where the transformations take place. These will be further discussed in Chapter 4, Transforming Data on Azure. This chapter will also teach you how to create and monitor data pipelines according to best practices.
Advanced analytics
For analyses that may be too complex to perform in the serving layer, an analytics suite or data science environment can be added to the architecture to perform advanced analytics and unlock ML capabilities. This component can often be added in a later stage of platform development, as it will mostly not influence the core working of the other layers. A data platform in an early phase of development can perfectly exist without this component.
One option for the advanced analytics suite is an ML workspace where data scientists can preprocess data, perform feature engineering, and train and deploy ML models. The latter may require additional components such as a container registry for storing and managing model deployments. The Azure Machine Learning workspace allows users to create and run ML pipelines to scale their data science processes. It also enables citizen data scientists to train models using no-code and low-code features.
Apart from an environment for data scientists and ML engineers to build and deploy custom models, the Azure cloud also provides users with a wide array of pre-trained ML models. Azure Cognitive Services encompass many models for computer vision (CV), speech recognition, text analytics, search capabilities, and so on. These models are available through ready-to-use API endpoints. They often involve niche cases but, when used correctly, bring a lot of value to the solution and are exceptionally fast to implement.
Chapter 9, Advanced Analytics Using AI, will go deeper into end-to-end ML workflows, such as the connection to data storages, performing preprocessing, model training, and model deployments. This chapter will also introduce the concepts of ML operations, often referred to as MLOps. This encompasses continuous integration and continuous development (CI/CD) for ML workflows.
Data governance and compliance
The more a data platform scales, the harder it becomes to maintain a clear overview of existing data sources, data assets, transformations, data access control, and compliance. To avoid a build-up of technical backlog, it is strongly recommended to start the setup of governance and compliance processes from an early stage of development and have it scale with the platform.
To govern Azure data platforms, Microsoft developed Microsoft Purview, formerly known as Azure Purview. This tool, which is covered in Chapter 10, Enterprise-Level Data Governance and Compliance, allows users to gain clear insights into the governance and compliance of the platform. Therefore, it is essential to the skill set of any aspiring Azure data architect. In this chapter, you will learn how to do the following:
- Create a data map by performing scans on data assets
- Construct a data catalog to provide an overview of the metadata of data assets
- Build a business glossary to establish clear definitions of possibly ambiguous business terms
- Gain executive insights on the entire data estate
Security
With the growing rise of harmful cyber-attacks, security is another indispensable component of a data platform. Improper security or configurations may lead to tremendous costs for the business. Investing in robust security to prevent attacks from happening will typically be vastly cheaper than dealing with the damage afterward.
Cybersecurity can be very complex and therefore should be configured and managed using the help of a cybersecurity architect. However, certain aspects of security should fall into the responsibilities of the data architect as well. The data architect should have the appropriate skill set to establish data security. Examples are working with row- or column-level security, data encryption at rest and in transit, masking sensitive data, and so on.
Chapter 11, Introduction to Data Security, will teach you all that is necessary to ensure data is always well protected and access is always limited to a minimum.
Monitoring
Disruptions such as failing data pipelines, breaking transformations, and unhealthy deployments can shut down the workings of an entire data platform. To limit the downtime to an absolute minimum, these processes and deployments should be monitored continuously.
Azure provides monitoring and health reports on pipeline runs, Spark and SQL jobs, ML model deployments, data asset scans, and more. The monitoring of these resources will be further discussed in their own respective chapters.