To understand VAEs, we need to talk about regular autoencoders. An autoencoder is a feed-forward neural network that tries to reproduce its input. In other words, the target value (label) of an autoencoder is equal to the input data, yi = xi, where i is the sample index. We can formally say that it tries to learn an identity function, (a function that repeats its input). Since our labels are just input data, the autoencoder is an unsupervised algorithm.
The following diagram represents an autoencoder:
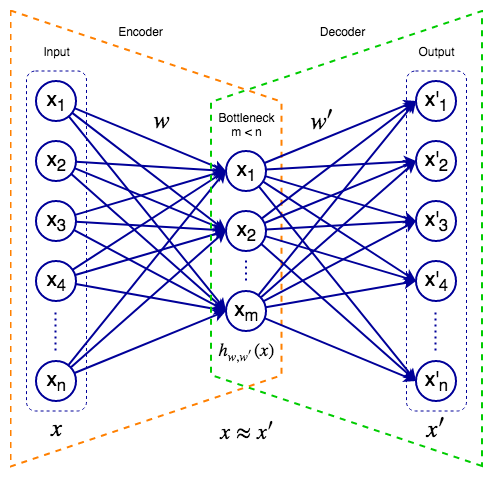
An autoencoder
An autoencoder consists of input, hidden (or bottleneck), and output layers. Similar to U-Net (Chapter 4, Object Detection and Image Segmentation), we can think of the autoencoder as a virtual composition of two components:
- Encoder: Maps the input data to the network's internal representation. For the sake of simplicity, in this example...