



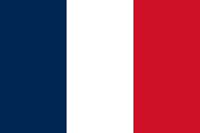


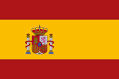



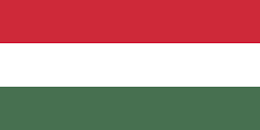

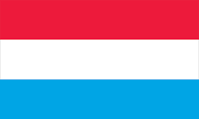





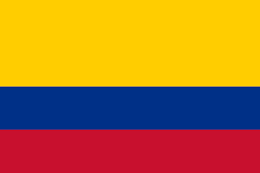
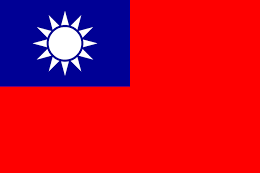
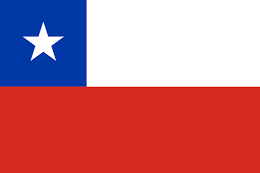



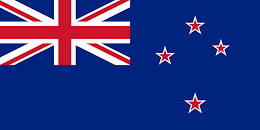
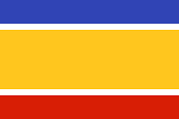
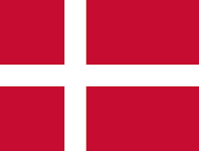
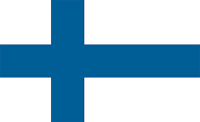







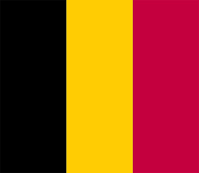

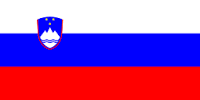





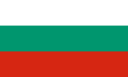


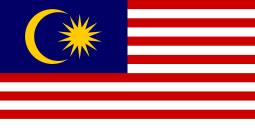



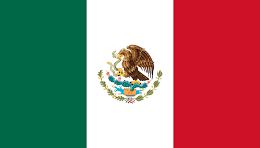

In case of any disaster, the first step is to formulate a critical response team to help those in distress. Before the team goes into action, it is important to analyze and assess the extent of damage and to ensure that the right aid goes first to those who need it the most. AI techniques such as image recognition and classification can be quite helpful in assessing the damage as they can analyze and observe images from the satellites. They can immediately and efficiently filter these images, which would have required months to be sorted manually. AI can identify objects and features such as damaged buildings, flooding, blocked roads from these images. They can also identify temporary settlements which may indicate that people are homeless, and so the first care could be directed towards them.
Artificial intelligence and machine learning tools can also aggregate and crunch data from multiple resources such as crowd-sourced mapping materials or Google maps. Machine learning approaches then combine all this data together, remove unreliable data, and identify informative sources to generate heat maps. These heat maps can identify areas in need of urgent assistance and direct relief efforts to those areas. Heat maps are also helpful for government and other humanitarian agencies in deciding where to conduct aerial assessments.
DigitalGlobe provides space imagery and geospatial content. Their Open Data Program is a special program for disaster response. The software learns how to recognize buildings on satellite photos by learning from the crowd. DigitalGlobe releases pre- and post-event imagery for select natural disasters each year, and their crowdsourcing platform, Tomnod, will prioritize micro-tasking to accelerate damage assessments.
Following the Nepal Earthquakes in 2015, Rescue Global and academicians from the Orchid Project used machine learning to carry out rescue activities. They took pre and post-disaster imagery and utilized crowd-sourced data analysis and machine learning to identify locations affected by the quakes that had not yet been assessed or received aid. This information was then shared with relief workforces to facilitate their activities.
911 is the first source of contact during any emergency situation. 911 dispatch centers are already overloaded with calls on a regular day. In case of a disaster or calamity, the number gets quadrupled, or even more. This calls for augmenting traditional 911 emergency centers with newer technologies for better management. Traditional 911 centers rely on voice-based calls alone. Next-gen dispatch services are upgrading their emergency dispatch technology with machine learning to receive more types of data. So now they can ingest the data from not just calls but also from text, video, audio, and pictures, to analyze them to make quick assessments. The insights gained from all this information can be passed on to the emergency response teams out in the field to efficiently carry out critical tasks.
The Association of Public-Safety Communications (APCO) have employed IBM’s Watson to listen to 911 calls. This initiative is to help emergency call centers improve operations and public safety by using Watson’s speech-to-text and analytics programs. Using Watson's speech-to-text function, the context of each call is fed into the AI's analytics program allowing improvements in how call centers respond to emergencies. It also helps in reducing call times, provide accurate information, and help accelerate time-sensitive emergency services.
Social media channels are a major source of news in present times. Some of the most actionable information, during a disaster, comes from social media users. Real-time images and comments from Facebook, Twitter, Instagram, and YouTube can be analyzed and validated by AI to filter real information from fake ones. These vital stats can help on-the-ground aid workers to reach the point of crisis sooner and direct their efforts to the needy. This data can also help rescue workers in reducing the time needed to find victims. In addition, AI and predictive analytics software can analyze digital content from Twitter, Facebook, and Youtube to provide early warnings, ground-level location data, and real-time report verification. In fact, AI could also be used to view the unstructured data and background of pictures and videos posted to social channels and compare them to find missing people.
AI-powered chatbots can help residents affected by a calamity. The chatbot can interact with the victim, or other citizens in the vicinity via popular social media channels and ask them to upload information such as location, a photo, and some description. The AI can then validate and check this information from other sources and pass on the relevant details to the disaster relief committee. This type of information can assist them with assessing damage in real time and help prioritize response efforts.
AI for Digital Response (AIDR) is a free and open platform which uses machine intelligence to automatically filter and classify social media messages related to emergencies, disasters, and humanitarian crises. For this, it uses a Collector and a Tagger. The Collector helps in collecting and filtering tweets using keywords and hashtags such as "cyclone" and "#Irma," for example. The Collector works as a word-filter. The Tagger is a topic-filter which classifies tweets by topics of interest, such as "Infrastructure Damage," and "Donations," for example. The Tagger automatically applies the classifier to incoming tweets collected in real-time using the Collector.
Emergency relief services are flooded with distress and help calls in the event of any emergency situation. Managing such a huge amount of calls is time-consuming and expensive when done manually. The chances of a critical information being lost or unobserved is also a possibility. In such cases, AI can work as a 24/7 dispatcher. AI systems and voice assistants can analyze massive amounts of calls, determine what type of incident occurred and verify the location. They can not only interact with callers naturally and process those calls, but can also instantly transcribe and translate languages. AI systems can analyze the tone of voice for urgency, filtering redundant or less urgent calls and prioritizing them based on the emergency.
Blueworx is a powerful IVR platform which uses AI to replace call center officials. Using AI technology is especially useful when unexpected events such as natural disasters drive up call volume. Their AI engine is well suited to respond to emergency calls as unlike a call center agent, it can know who a customer is even before they call. It also provides intelligent call routing, proactive outbound notifications, unified messaging, and Interactive Voice Response.
Machine learning and other data science approaches are not limited to assisting the on-ground relief teams or assisting only after the actual emergency. Machine learning approaches such as predictive analytics can also analyze past events to identify and extract patterns and populations vulnerable to natural calamities. A large number of supervised and unsupervised learning approaches are used to identify at-risk areas and improve predictions of future events. For instance, clustering algorithms can classify disaster data on the basis of severity. They can identify and segregate climatic patterns which may cause local storms with the cloud conditions which may lead to a widespread cyclone.
Predictive machine learning models can also help officials distribute supplies to where people are going, rather than where they were by analyzing real-time behavior and movement of people.
In addition, predictive analytics techniques can also provide insight for understanding the economic and human impact of natural calamities. Artificial neural networks take in information such as region, country, and natural disaster type to predict the potential monetary impact of natural disasters.
Recent advances in cloud technologies and numerous open source tools have enabled predictive analytics with almost no initial infrastructure investment. So agencies with limited resources can also build systems based on data science and develop more sophisticated models to analyze disasters.
Optima Predict, a suite of software by Intermedix collects and reads information about disasters such as viral outbreaks or criminal activity in real time. The software spots geographical clusters of reported incidents before humans notice the trend and then alerts key officials about it. The data can also be synced with FirstWatch, which is an online dashboard for an EMS (Emergency Medical Services) personnel.
Thanks to the multiple benefits of AI, government agencies and NGOs can start utilizing machine learning to deal with disasters. As AI and allied fields like robotics further develop and expand, we may see a fleet of drone services, equipped with sophisticated machine learning. These advanced drones could expedite access to real-time information at disaster sites using video capturing capabilities and also deliver lightweight physical goods to hard to reach areas.
As with every progressing technology, AI will also build on its existing capabilities. It has the potential to eliminate outages before they are detected and give disaster response leaders an informed, clearer picture of the disaster area, ultimately saving lives.