



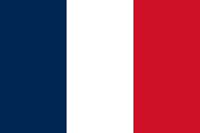


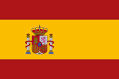



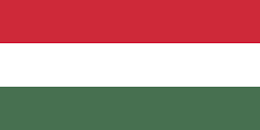

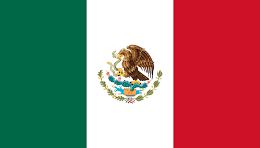


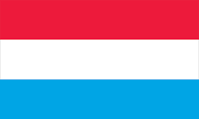



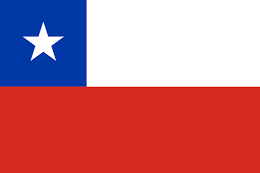


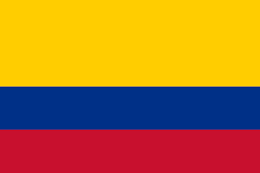
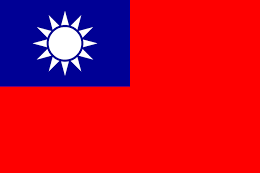


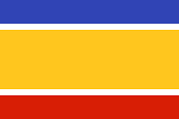
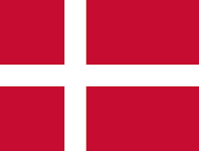
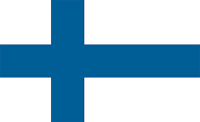



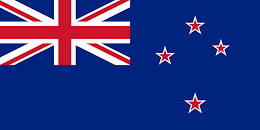





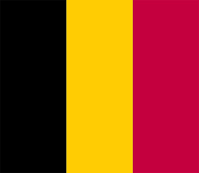

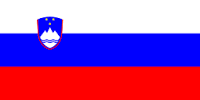




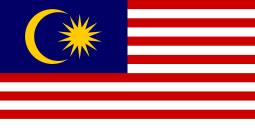


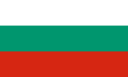



The world of technology, characterized by an incessant and rapid pace of evolution, is on the cusp of a seismic shift. Historically, the development and control of large language models—a key component in modern artificial intelligence systems—have been dominated by tech industry giants. However, emerging developments show that this might not be the status quo for much longer. The burgeoning field of open-source LLMs presents a potential disruption to the current balance of power in the tech industry, signaling a shift towards a more democratic and inclusive AI landscape.
Major tech firms like Microsoft and Google, armed with vast financial resources, have long held the reins of the LLM market. Their position seemed unassailable as recent earnings calls indicated a thriving business built around their AI services. Yet, a leaked internal document from Google has cast a shadow of uncertainty over this seemingly secure stronghold. The central idea gleaned from this document? No company has an unassailable fortress against competition in the realm of LLMs, not even the mighty OpenAI, the organization responsible for the groundbreaking GPT-3.
The story of GPT-3 is a pivotal chapter in the annals of AI history. Its 2020 release ignited a spark in the research community, illuminating the tantalizing promise of scale. With 175 billion parameters, GPT-3 showed capabilities that stretched beyond its initial training data. The success of this LLM prompted a surge of interest in the creation of larger, more complex models. This development led to an arms race among AI research labs, producing increasingly massive models such as Gopher, LaMDA, PaLM, and Megatron-Turing.
However, this race towards larger LLMs engendered a substantial increase in research and development costs. The staggering financial demands associated with training and running models like GPT-3 created an environment where LLM innovation was essentially confined to the wealthiest entities in tech. With this economic pressure to recoup their considerable investment, these companies began to commercialize their technology, leading to the erection of protective "moats" around their products. These mechanisms of defensibility safeguarded their investments against the competition, obscuring their research and constraining the sharing of intellectual resources.
Key elements of these moats included the proprietary control over training data, model weights, and the costs associated with training and inference. With their deep pockets, big tech companies kept the upper hand in managing the expenses tied to training and running large LLMs. This dominance rendered even open-source alternatives such as BLOOM and OPT175-B largely inaccessible to organizations without the fiscal means to support the hefty demands of these advanced models.
For a time, this state of affairs painted a bleak picture for the democratization of LLMs, with the field becoming increasingly exclusive and secretive. However, the ebb and flow of innovation and competition that define the tech industry were bound to respond. The open-source community rose to the challenge, their endeavors intensifying following the release of OpenAI's ChatGPT, an instruction-following language model that illustrated the vast potential of LLMs in a multitude of applications.
These open-source alternatives are changing the game by proving that performance is not solely a function of scale. Small, nimble LLMs trained on expansive datasets have proven the ability to compete head-to-head with their larger counterparts. Moreover, the open-source models, often consisting of 7-13 billion parameters, can be fine-tuned to remarkable degrees on a modest budget and can run on consumer-grade GPUs.
One such example, the open-source LLM developed by Meta, known as LLaMA, sparked a wave of similar models like Alpaca and Vicuna. These models, constructed on top of LLaMA, displayed an impressive capability for instruction-following akin to ChatGPT. The subsequent release of Dolly 2.0 by Databricks and Open Assistant further enriched the field by providing commercially usable, instruction-following LLMs that organizations can tailor to their specific needs.
The impact of these open-source models is profound. They potentially democratize access to advanced AI systems, reducing the cost of training by using techniques like low-rank adaptation (LoRA) and allowing businesses to incorporate LLMs into their operations at an affordable price. This development poses a significant challenge to the established order, undermining the monopoly of tech giants on LLMs.
Nonetheless, the rise of open-source models does not spell the end of cloud-based language models. Despite the democratization they promise, open-source LLMs face significant hurdles, including the prohibitive costs of pre-training. Furthermore, they may not be the best choice for all businesses. Companies without in-house machine learning expertise may still prefer the convenience of out-of-the-box, serverless solutions provided by the likes of Microsoft and Google. The entrenched distribution channels of these tech behemoths also present a formidable barrier for open-source LLMs to overcome.
However, the broader implications of the open-source movement in LLMs are unmistakable. It expands the market, opens up novel applications, and puts pressure on tech giants to offer more competitive pricing. By democratizing access to advanced AI, it allows for broader participation in the AI revolution, reducing the concentration of power and innovation within a few wealthy tech companies. As the LLM landscape continues to evolve rapidly, the rise of open-source models will leave an indelible mark on the tech industry.
Julian Melanson is one of the founders of Leap Year Learning. Leap Year Learning is a cutting-edge online school that specializes in teaching creative disciplines and integrating AI tools. We believe that creativity and AI are the keys to a successful future and our courses help equip students with the skills they need to succeed in a continuously evolving world. Our seasoned instructors bring real-world experience to the virtual classroom and our interactive lessons help students reinforce their learning with hands-on activities.
No matter your background, from beginners to experts, hobbyists to professionals, Leap Year Learning is here to bring in the future of creativity, productivity, and learning!