Keras
Keras is designed to be a high-level neural network API that is built on top of frameworks such as TensorFlow, CNTK, and Theano. One of the great benefits of using Keras as an introduction to deep learning for beginners is that it is very user-friendly; advanced functions such as optimizers and layers are already built into the library and do not have to be written from scratch. This is why Keras is popular not only among beginners but also seasoned experts. Also, the library allows the rapid prototyping of neural networks, supports a wide variety of network architectures, and can be run on both CPUs and GPUs.
Note
You can find the library and all the documentation for Keras here: https://Keras.io/.
Keras is used to create and train neural networks and does not offer much in terms of other machine learning algorithms, including supervised algorithms such as support vector machines and unsupervised algorithms such as k-means clustering
. What Keras does offer, though, is a well-designed API that can be used to create and train neural networks, which takes away much of the effort that's required to apply linear algebra and multivariate calculus accurately.
The specific modules that are available from the Keras library, such as neural layers
, cost functions
, optimizers
, initialization schemes
, activation functions
, and regularization schemes
, will be explained thoroughly throughout this book. All these modules have relevant functions that can be used to optimize performance for training neural networks for specific tasks.
Advantages of Keras
Here are a few of the main advantages of using Keras for machine learning purposes:
- User-friendly: Much like scikit-learn, Keras features an easy-to-use API that allows users to focus on model-building rather than the specifics of the algorithms.
- Modular: The API consists of fully configurable modules that can all be plugged together and work seamlessly.
- Extensible: It is relatively simple to add new modules to the library. This allows users to take advantage of the many robust modules within the library while providing them the flexibility to create their own.
- Open source: Keras is an open source library and is constantly improving and adding modules to its code base thanks to the work of many collaborators working in conjunction to build improvements and help create a robust library for all.
- Works with Python: Keras models are declared directly in Python rather than in separate configuration files, which allows Keras to take advantage of working with Python, such as ease of debugging and extensibility.
Disadvantages of Keras
Here are a few of the main disadvantages of using Keras for machine learning purposes:
- Advanced customization: While simple surface-level customization such as creating simple custom loss functions or neural layers is facile, it can be difficult to change how the underlying architecture works.
- Lack of examples: Beginners often rely on examples to kick-start their learning. Advanced examples can be lacking in the Keras documentation, which can prevent beginners from advancing in their learning.
Keras offers those familiar with the Python programming language and machine learning the ability to create neural network architectures easily. Since neural networks are quite complicated, we will use scikit-learn to introduce many machine learning concepts before applying them to the Keras library.
More Than Building Models
While machine learning libraries such as scikit-learn and Keras were created to help build and train predictive models, their practicality extends much further. One common use case of building models is that they can be utilized to perform predictions on new data. Once a model has been trained, new observations can be fed into the model to generate predictions. Models may even be used as intermediate steps. For example, neural network models can be used as feature extractors
, classifying objects in an image that can then be fed into a subsequent model, as illustrated in the following image:
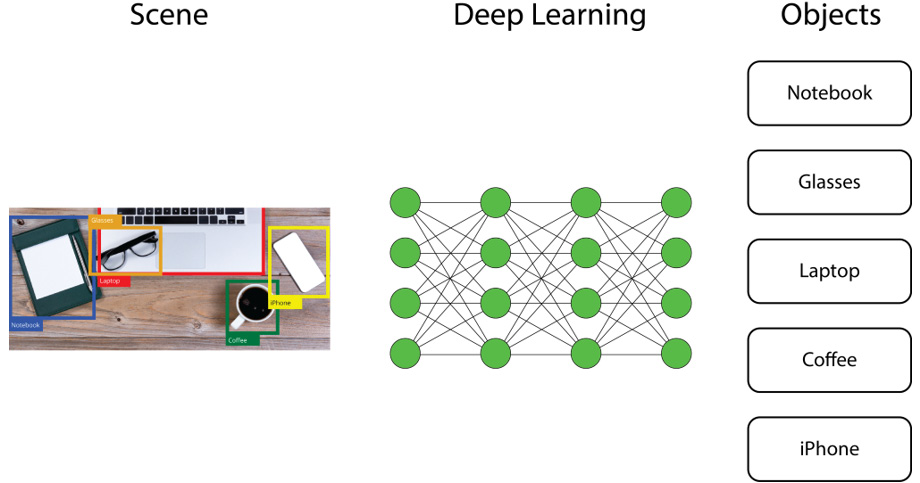
Figure 1.24: Classifying objects using deep learning
Another common use case for models is that they can be used to summarize datasets by learning representations of the data. Such models are known as auto-encoders, a type of neural network architecture that can be used to learn such representations of a given dataset. Therefore, the dataset can thus be represented in a reduced dimension with minimal loss of information:
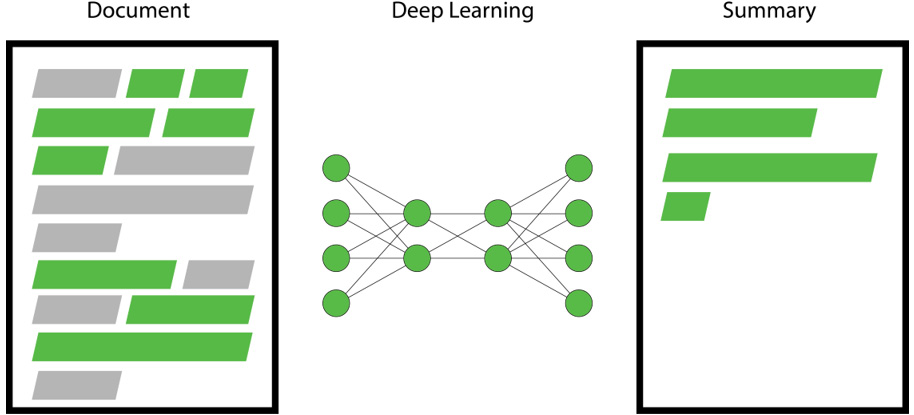
Figure 1.25: An example of using deep learning for text summarization